Offre de thèse
ENACT : Optimisation de la ventilation mécanique en réanimation pédiatrique par l'intelligence artificielle : développement d'un modèle de fondation respiratoire intégré à un système d'aide à la décision clinique
Date limite de candidature
01-07-2025
Date de début de contrat
01-10-2025
Directeur de thèse
SCHWEITZER Cyril
Encadrement
Co-Directeur à l'UL : Professeur Cyril SCHWEITZER, MD, PhD, HdR, Réanimation pédiatrique (UR DeVAH 3450) Co-encadrant pour la partie UL: Dr Arnaud WIEDEMANN, MD, PhD, demande d'HdR en cours, Réanimation pédiatrique (UR NGERE 1256) Direction au CANADA : Professeur Philippe JOUVET, MD, PhD, HdR, PICU Montréal
Type de contrat
école doctorale
équipe
Equipe 1 : Développement et adaptationcontexte
L'optimisation de ces paramètres est cruciale et nécessite une adaptation constante aux changements physiologiques du patient. Un SIADC (Système Informatisé d'Aide à la Décision Clinique, ou CDSS en anglais), capable d'analyser en temps réel ces variables et de recommander des ajustements, peut grandement améliorer la prise en charge ventilatoire. Les CDSS sont des outils logiciels qui assistent les cliniciens en analysant de grandes quantités de données afin de proposer des recommandations diagnostiques et thérapeutiques. L'intégration de l'intelligence artificielle dans ces systèmes permet de reconnaître des schémas complexes et d'anticiper l'évolution des paramètres physiologiques. Plusieurs approches d'IA sont envisagées pour ce projet : ● Apprentissage supervisé : utilisé pour prédire les gaz du sang (PaCO₂, PaO₂ , pH) à partir des réglages ventilatoires et des variables cliniques. ● Réseaux neuronaux profonds et Transformers : capables d'analyser des séries temporelles complexes pour détecter des tendances et anticiper les changements de l'état respiratoire . ● Apprentissage par renforcement : permettrait, via des simulations, d'optimiser en temps réel les paramètres ventilatoires en maximisant des indicateurs de stabilité physiologique.spécialité
Sciences de la Vie et de la Santé - BioSElaboratoire
DevAH - Développement, Adaptation et Handicap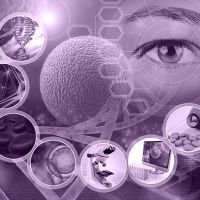
Mots clés
Intelligence artificielle , Ventilation mécanique enfant, Système d'aide à la décision clinique , Modélisation physiologique, Apprentissage automatique , Régulation ventilatoire
Détail de l'offre
Ce projet de thèse se déroulera au sein du CHRU de Nancy (Réanimation pédiatrique du CHRU Nancy) et dans les UR de l'Université de Lorraine. La réanimation pédiatrique est une unité reconnue pour son expertise en soins intensifs pédiatriques et pour ses approches avancées en physiologie respiratoire. Le service ambitionne de rejoindre le consortium international CDSS Lab, dirigé par l'équipe du Service des Soins Intensifs Pédiatriques (SIP) du CHU Sainte-Justine, Université de Montréal. Ce consortium est mondialement reconnu pour son expertise en application de l'intelligence artificielle (IA) à la ventilation mécanique pédiatrique depuis plus de 15 ans, ainsi que pour ses multiples publications scientifiques internationales et l'organisation d'un congrès international annuel.
Le projet doctoral bénéficiera d'une cotutelle internationale entre l'Université de Lorraine (France) et l'Université de Montréal (Canada). Il s'inscrit pleinement dans le cadre du programme IA Cluster ENACT, qui vise à renforcer la position du Grand Est comme pôle européen majeur dans les domaines de la recherche, de l'innovation et de la formation en intelligence artificielle. Il correspond à la thématique prioritaire de la « santé numérique » développée par ENACT. Un demi-financement est sollicité auprès du programme IA Cluster ENACT, avec un cofinancement complémentaire assuré par l'Université de Montréal, confirmé par un engagement écrit du Professeur Philippe Jouvet (PICU Sainte-Justine, Université de Montréal).
Ce projet accompagnera la mise en place, au sein du CHRU Nancy, d'un système de dossiers médicaux informatisés incluant un logiciel de prescription et de monitoring clinique, permettant l'extraction en temps réel des données issues des soins intensifs et la constitution de bases de données robustes pour les travaux d'intelligence artificielle et de machine learning. Cette démarche s'inspire directement des méthodes et des infrastructures mises en place avec succès à Sainte-Justine à Montréal et de mettre au point le simulateur SimulResp, qui permet de reproduire la physiologie respiratoire de l'enfant et de l'adolescent et de prévoir l'effet de différents réglages ventilatoires sur les paramètres physiologiques des patients. L'objectif de ce projet est de tirer parti de l'IA pour faire évoluer SimulResp en un modèle de fondation respiratoire plus robuste, capable de prédire en temps réel l'état gazométrique et d'assister la prise de décision ventilatoire.
Ce projet ambitieux conjugue physiologie respiratoire et intelligence artificielle pour répondre à un besoin clinique critique en réanimation pédiatrique. En améliorant le simulateur SimulResp via un modèle de fondation respiratoire enrichi par l'IA, nous proposons une innovation de rupture : un outil capable de prévoir l'évolution des gaz du sang et de recommander en temps réel les réglages ventilatoires optimaux pour chaque patient.
L'intégration d'un système informatisé d'aide à la décision clinique basé sur l'intelligence artificielle pour la ventilation mécanique en réanimation pédiatrique constitue une avancée majeure. Ces systèmes exploitent des algorithmes capables d'analyser en temps réel un volume considérable de données cliniques, permettant ainsi d'anticiper les réponses aux ajustements ventilatoires et de proposer des recommandations optimisées. Leur utilisation a démontré une réduction de la variabilité des pratiques, une amélioration des résultats cliniques et une diminution des délais d'extubation . Contrairement aux protocoles statiques, un SIADC s'adapte dynamiquement aux spécificités physiologiques individuelles des patients pédiatriques. Dans ce contexte, l'amélioration du simulateur SimulResp grâce à l'IA représente une opportunité d'optimiser la prise en charge des patients en soins intensifs. Un tel système pourrait révolutionner la médecine personnalisée en ventilation pédiatrique, offrant une adaptation en temps réel aux besoins spécifiques de chaque enfant.
Keywords
Artificial intelligence, Pediatric mechanical ventilation, Physiological modeling, Clinical decision support system , Machine learning, ventilatory regulation
Subject details
This PhD project will take place within the Pediatric Intensive Care unit at CHRU Nancy (University of Lorraine), an internationally recognized unit for its expertise in the management of critically ill children and its advanced approaches in respiratory physiology. The Pediatric Intensive Care Unit at CHRU Nancy aims to join the international CDSS Lab consortium, led by the Pediatric Intensive Care Service (SIP) team at CHU Sainte-Justine, University of Montreal. This consortium has been globally recognized for over 15 years for its expertise in applying artificial intelligence (AI) to pediatric mechanical ventilation, as well as for its numerous international scientific publications and the organization of an annual international conference. The doctoral project will benefit from an international co-supervision between the University of Lorraine (France) and the University of Montreal (Canada). This project is fully aligned with the IA Cluster ENACT program, which aims to strengthen the Grand Est region as a major European hub for research, innovation, and training in artificial intelligence. It specifically corresponds to the priority theme of 'digital health' developed by ENACT. A partial funding request has been submitted to the IA Cluster ENACT program, with additional co-funding provided by the University of Montreal, confirmed by a written commitment from Professor Philippe Jouvet (PICU Sainte-Justine, University of Montreal). At the same time, this project will support the implementation of a computerized medical record system at CHRU Nancy, including prescription and clinical monitoring software, enabling real-time extraction of intensive care data and the creation of robust databases for artificial intelligence and machine learning research. This approach directly draws inspiration from the methods and infrastructures successfully implemented at the Pediatric Intensive Care Unit (SIP) of Sainte-Justine. SIP of Sainte-Justine has developed the SimulResp simulator, which replicates the respiratory physiology of children and adolescents and predicts the effects of different ventilatory settings on patients' physiological parameters. This simulator has significant educational value, as it allows for learning the principles of mechanical ventilation and observing the effects of prescribed modifications on patient evolution. It may also play a clinical role, as its integration into a CDSS could help optimize ventilatory settings for patients who are challenging to stabilize. The objective of this project is to leverage AI to enhance SimulResp into a more robust foundational respiratory model, capable of real-time blood gas prediction and assisting ventilatory decision-making. This ambitious project combines respiratory physiology and artificial intelligence to address a critical clinical need in pediatric intensive care. By improving SimulResp with an AI-powered foundational respiratory model, we propose a disruptive innovation: a tool capable of predicting blood gas evolution and recommending optimal ventilatory settings in real time. The integration of an AI-based Clinical Decision Support System for mechanical ventilation in pediatric intensive care represents a major advancement. These systems leverage algorithms capable of analyzing a vast volume of clinical data in real time, allowing for the anticipation of responses to ventilatory adjustments and the proposal of optimized recommendations. Their use has been shown to reduce practice variability, improve clinical outcomes, and shorten extubation times. Unlike static protocols, a CDSS dynamically adapts to the individual physiological specificities of pediatric patients. In this context, enhancing the SimulResp simulator with AI presents a unique opportunity to optimize the management patients. By continuously refining its predictions and recommendations through clinical data learning, such a system could revolutionize personalized medicine in pediatric ventilation.
Profil du candidat
Profil et compétences requises
IA et Apprentissage Automatique : Apprentissage Machine/Profond (ML/DL), Séries temporelles, Apprentissage Supervisé et Non Supervisé, Apprentissage par Renforcement, Apprentissage de Représentation, Modélisation Prédictive, Réseaux de Neurones, IA pour la Santé (Prédiction en Soins Intensifs, Tri de l'Hypoxie), Interprétabilité des Modèles ML, IA Fiable.
Programmation et Science des Données : Python (NumPy, Pandas, Scikit-learn, TensorFlow, PyTorch, Keras), Prétraitement des Données, Ingénierie des Caractéristiques, Suivi des Expériences (Weights & Biases), Optimisation des Algorithmes.
Biologie et Sciences de la Vie : Biologie Computationnelle et Systémique, Neurophysiologie, Signalisation Cellulaire, Métabolisme, Modélisation Epidémiologique (SIR, SEIRS).
Compétences Interpersonnelles : Recherche et Rédaction Scientifique, Collaboration Interdisciplinaire, Résolution de Problèmes, Pensée Critique.
Candidate profile
Profile and skills required
AI & Machine Learning: ML/DL, Time Series, Supervised & Unsupervised Learning, Reinforcement Learning, Representation Learning, Predictive Modeling, Neural Networks, AI for Healthcare (ICU prediction, hypoxia triage), ML Model Interpretability, Trustworthy AI.
Programming & Data Science: Python (NumPy, Pandas, Scikit-learn, TensorFlow, PyTorch, Keras), Data Preprocessing, Feature Engineering, Experiment Tracking (Weights & Biases), Algorithmic Optimization.
Biology & Life Sciences: Computational & Systems Biology, Neurophysiology, Cell Signaling, Metabolism, Epidemiological Modeling (SIR, SEIRS).
Soft Skills: Research & Scientific Writing, Cross-disciplinary Collaboration, Problem-Solving, Critical Thinking.
Référence biblio
1. Brossier, D.; Flechelles, O.; Sauthier, M.; Engert, C.; Chahir, Y.; Emeriaud, G.; Cheriet, F.; Jouvet, P.; de Montigny, S. Evaluation of the SIMULRESP: A Simulation Software of Child and Teenager Cardiorespiratory Physiology. Pediatr. Pulmonol. 2023, 58, 2832–2840, doi:10.1002/ppul.26595.
2. Lone, N.I.; Walsh, T.S. Prolonged Mechanical Ventilation in Critically Ill Patients: Epidemiology, Outcomes and Modelling the Potential Cost Consequences of Establishing a Regional Weaning Unit. Crit. Care 2011, 15, R102, doi:10.1186/cc10117.
3. Gallifant, J.; Zhang, J.; del Pilar Arias Lopez, M.; Zhu, T.; Camporota, L.; Celi, L.A.; Formenti, F. Artificial Intelligence for Mechanical Ventilation: Systematic Review of Design, Reporting Standards, and Bias. BJA Br. J. Anaesth. 2022, 128, 343–351, doi:10.1016/j.bja.2021.09.025.
4. Al-Jaghbeer, M.; Kellum, J.A. Acid-Base Disturbances in Intensive Care Patients: Etiology, Pathophysiology and Treatment. Nephrol. Dial. Transplant. Off. Publ. Eur. Dial. Transpl. Assoc. - Eur. Ren. Assoc. 2015, 30, 1104–1111, doi:10.1093/ndt/gfu289.
5. West's Respiratory Physiology: The Essentials, Tenth Edition Available online: https://thepoint.lww.com/book/show/625100# (accessed on 10 March 2025).
6. Front Matter. In Nunn's Applied Respiratory Physiology (Eighth Edition); Lumb, A.B., Ed.; Elsevier, 2017; pp. i–ii ISBN 978-0-7020-6294-0.
7. Tobin, M.J. Mechanical Ventilation. N. Engl. J. Med. 1994, 330, 1056–1061, doi:10.1056/NEJM199404143301507.
8. Slutsky, A.S.; Ranieri, V.M. Ventilator-Induced Lung Injury. N. Engl. J. Med. 2013, 369, 2126–2136, doi:10.1056/NEJMra1208707.
9. Baptistella, A.; Carvalho, D.; Dallacosta, F.; Filho, J.R.N. NexoVent: Artificial Intelligence Applied to the Management of Mechanical Ventilation. ERJ Open Res. 2024, 10, doi:10.1183/23120541.RFMV-2024.57.
10. Jonkman, A.H.; de Vries, H.J.; Heunks, L.M.A. Physiology of the Respiratory Drive in ICU Patients: Implications for Diagnosis and Treatment. Crit. Care 2020, 24, 104, doi:10.1186/s13054-020-2776-z.
11. Briel, M.; Meade, M.; Mercat, A.; Brower, R.G.; Talmor, D.; Walter, S.D.; Slutsky, A.S.; Pullenayegum, E.; Zhou, Q.; Cook, D.; et al. Higher vs Lower Positive End-Expiratory Pressure in Patients with Acute Lung Injury and Acute Respiratory Distress Syndrome: Systematic Review and Meta-Analysis. JAMA 2010, 303, 865–873, doi:10.1001/jama.2010.218.
12. Acute Respiratory Distress Syndrome Network; Brower, R.G.; Matthay, M.A.; Morris, A.; Schoenfeld, D.; Thompson, B.T.; Wheeler, A. Ventilation with Lower Tidal Volumes as Compared with Traditional Tidal Volumes for Acute Lung Injury and the Acute Respiratory Distress Syndrome. N. Engl. J. Med. 2000, 342, 1301–1308, doi:10.1056/NEJM200005043421801.
13. Arnal, J.-M.; Katayama, S.; Howard, C. Closed-Loop Ventilation. Curr. Opin. Crit. Care 2023, 29, 19–25, doi:10.1097/MCC.0000000000001012.
14. Murali, M.; Ni, M.; Karbing, D.S.; Rees, S.E.; Komorowski, M.; Marshall, D.; Ramnarayan, P.; Patel, B.V. Clinical Practice, Decision-Making, and Use of Clinical Decision Support Systems in Invasive Mechanical Ventilation: A Narrative Review. BJA Br. J. Anaesth. 2024, 133, 164–177, doi:10.1016/j.bja.2024.03.011.
15. Misseri, G.; Piattoli, M.; Cuttone, G.; Gregoretti, C.; Bignami, E.G. Artificial Intelligence for Mechanical Ventilation: A Transformative Shift in Critical Care. Ther. Adv. Pulm. Crit. Care Med. 2024, 19, 29768675241298918, doi:10.1177/29768675241298918.
16. Han, H.-J.; Lee, B.; Park, J.D. Individualized Estimation of Arterial Carbon Dioxide Partial Pressure Using Machine Learning in Children Receiving Mechanical Ventilation. BMC Pediatr. 2024, 24, 149, doi:10.1186/s12887-024-04642-0.
17. Händel, C.; Frerichs, I.; Weiler, N.; Bergh, B. Prediction and Simulation of PEEP Setting Effects with Machine Learning Models. Med. Intensiva Engl. Ed. 2024, 48, 191–199, doi:10.1016/j.medine.2023.09.005.
18. Stivi, T.; Padawer, D.; Dirini, N.; Nachshon, A.; Batzofin, B.M.; Ledot, S. Using Artificial Intelligence to Predict Mechanical Ventilation Weaning Success in Patients with Respiratory Failure, Including Those with Acute Respiratory Distress Syndrome. J. Clin. Med. 2024, 13, 1505, doi:10.3390/jcm13051505.
19. Kim, G.-H.; Kim, J.-W.; Kim, K.H.; Kang, H.; Moon, J.Y.; Shin, Y.M.; Park, S. FT-GAT: Graph Neural Network for Predicting Spontaneous Breathing Trial Success in Patients with Mechanical Ventilation. Comput. Methods Programs Biomed. 2023, 240, 107673, doi:10.1016/j.cmpb.2023.107673.
20. Viderman, D.; Ayazbay, A.; Kalzhan, B.; Bayakhmetova, S.; Tungushpayev, M.; Abdildin, Y. Artificial Intelligence in the Management of Patients with Respiratory Failure Requiring Mechanical Ventilation: A Scoping Review. J. Clin. Med. 2024, 13, 7535, doi:10.3390/jcm13247535.
21. Liu, S.; Xu, Q.; Xu, Z.; Liu, Z.; Sun, X.; Xie, G.; Feng, M.; See, K.C. Reinforcement Learning to Optimize Ventilator Settings for Patients on Invasive Mechanical Ventilation: Retrospective Study. J. Med. Internet Res. 2024, 26, e44494, doi:10.2196/44494.
22. Kondrup, F.; Jiralerspong, T.; Lau, E.; de Lara, N.; Shkrob, J.; Tran, M.D.; Precup, D.; Basu, S. Towards Safe Mechanical Ventilation Treatment Using Deep Offline Reinforcement Learning. In Proceedings of the Proceedings of the Thirty-Seventh AAAI Conference on Artificial Intelligence and Thirty-Fifth Conference on Innovative Applications of Artificial Intelligence and Thirteenth Symposium on Educational Advances in Artificial Intelligence; AAAI Press, February 7 2023; Vol. 37, pp. 15696–15702.
23. Dickinson, C.J. A Computer Model of Human Respiration : Ventilation, Blood Gas Transport and Exchange, Hydrogen Ion Regulation : For Teaching, Research and Clinical Use, “MacPuf”; Baltimore : University Park Press, 1977; ISBN 978-0-8391-1144-3.
24. Radermacher, P.; Maggiore, S.M.; Mercat, A. Fifty Years of Research in ARDS. Gas Exchange in Acute Respiratory Distress Syndrome. Am. J. Respir. Crit. Care Med. 2017, 196, 964–984, doi:10.1164/rccm.201610-2156SO.
25. Moazemi, S.; Vahdati, S.; Li, J.; Kalkhoff, S.; Castano, L.J.V.; Dewitz, B.; Bibo, R.; Sabouniaghdam, P.; Tootooni, M.S.; Bundschuh, R.A.; et al. Artificial Intelligence for Clinical Decision Support for Monitoring Patients in Cardiovascular ICUs: A Systematic Review. Front. Med. 2023, 10, 1109411, doi:10.3389/fmed.2023.1109411.
26. Mahmud, F.Bt.; Mohd Yusof, M.; Shahrul, A.N. Ontological Based Clinical Decision Support System (CDSS) for Weaning Ventilator in Intensive Care Unit (ICU). In Proceedings of the Proceedings of the 2011 International Conference on Electrical Engineering and Informatics; July 2011; pp. 1–5.
27. Burki, T.K. Artificial Intelligence Hold Promise in the ICU. Lancet Respir. Med. 2021, 9, 826–828, doi:10.1016/S2213-2600(21)00317-9.