Offre de thèse
ENACT Opérateurs neuronaux pilotés par l'IA pour la modélisation des transformations de phase dans les matériaux
Date limite de candidature
20-04-2025
Date de début de contrat
01-10-2025
Directeur de thèse
ZALOŽNIK Miha
Encadrement
Le thèse aura lieu à l'Institut Jean Lamour (IJL), et à l'Institut Élie Cartan de Lorraine (IECL) à Nancy. Elle sera encadrée par Miha Založnik, spécialiste de modélisation multi-échelles de la solidification, par Ludovick Gagnon, spécialiste des équations aux dérivées partielles et par Benoît Appolaire, spécialiste de la modélisation des transformations de phase à l'état solide et de l méthode du champ de phase. La/le doctorant/e profitera d'un environnement scientifique interdisciplinaire : modélisation multi-physique, mathématiques, matériaux, apprentissage automatique.
Type de contrat
école doctorale
équipe
DEPARTEMENT 3 - SI2M : 302 - Solidificationcontexte
This PhD offer is provided by the ENACT AI Cluster and its partners. Find all ENACT PhD offers and actions on https://cluster-ia-enact.ai Institut Jean Lamour (IJL) is one of the largest materials science research centers in Europe, with around 500 researchers, PhD students and technical staff. We work in metallurgy, nanomaterials, plasma physics, surface physicochemistry. The Department of Science and Engineering of Materials and Metallurgy (SI2M) focuses on metallurgical processes ranging from liquid metal treatment over solidification to solid transformation processes; all with the objective to control the formation of the structure of the final product. We work in tight collaboration with the industry and with international academic partners on a wide spectrum of projects, integrating industrial and fundamental problems. The Solidification group studies liquid-solid phase transformations to improve metallurgical products processed by casting, welding, additive manufacturing (3D printing) etc. We cover all the characteristic scales of solidification from the atom to the industrial product. The Microstructures and Stresses group studies the formation of microstructures in metallic alloys through phase transformations in solid state and internal stresses during thermal, thermomechanical or thermochemical processing. In both groups we focus on understanding the relationships between processing, microstructure and mechanical properties to optimize alloys for high-performance products in industries such as aeronautics, automotive, and nuclear energy. Our research combines original model experiments and multiscale numerical modeling with materials characterization. A synergy of fundamental and oriented research is developed in close collaboration with industrial and academic partners. Find more information on the research groups on: https://ijl.univ-lorraine.fr/en/research-groups/solidification-group https://ijl.univ-lorraine.fr/en/research-groups/microstructures-and-stresses-group Specializing in a wide range of pure and applied mathematical disciplines, the Institut Élie Cartan de Lorraine (IECL) is one of France's largest mathematics laboratories, and the largest in the Grand Est region. It has hosted world-renowned mathematicians such as Jean Leray, Jean Dieudonné, Laurent Schwartz (Fields Medal 1950), Jean-Pierre Serre (Fields Medal 1954), Alexander Grothendieck (Fields Medal 1966) and Jacques-Louis Lions. Partial differential equations (PDE) team is one of the four research groups of the IECL. It gathers around 30 permanent researchers in different aspects of the PDEs, from theoretical research to numerical analysis and scientific computing. Recent developments in scientific computing at IECL has led to numerous industrial collaborations. Find more information on PDE research group at : https://iecl.univ-lorraine.fr/partial-differential-equations/spécialité
Sciences des Matériauxlaboratoire
IJL - INSTITUT JEAN LAMOUR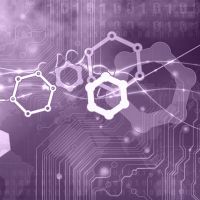
Mots clés
Transformations de phase, Matériaux, Modelès champ de phase, Équations aux dérivées partielles, Réseaux de neurones, Opérateurs neuronaux
Détail de l'offre
Les transformations de phase jouent un rôle important dans l'élaboration des matériaux métalliques, car elles déterminent la structure et, par conséquent, les propriétés du matériau. Les modèles de champ de phase décrivant ces transformations sont formulés par des équations aux dérivées partielles (EDP) de type réaction-diffusion. Cette description permet de coupler les transformations de phase à des champs externes (température, composition chimique, contraintes, écoulement liquide, etc.)
Aujourd'hui, ces EDP sont généralement résolues numériquement par des méthodes de discrétisation classiques. La nature non linéaire des modèles nécessite des discrétisations fines en espace et en temps, ce qui entraîne des calculs longs et des simulations coûteuses. Ce cout est prohibitif pour un passage à l'échelle du procédé et pour la réalisation d'études paramétriques approfondies. Les méthodes d'IA récemment développées pour l'approximation des solutions d'EDP ont le potentiel d'être beaucoup plus rapides et donc de révolutionner la modélisation des transformations de phase. Ces méthodes reposent sur des modèles de substitution basés sur des réseaux de neurones (RN).
La plupart des approches d'apprentissage des RN sont basés sur les données, nécessitant des ensembles de données conséquents et conduisant souvent à une instabilité des prédictions. Ces approches souffrent également d'une généralisation limitée – c'est-à-dire d'incapacité à prédire des résultats en dehors de la plage des paramètres de l'ensemble d'apprentissage. Une meilleure généralisation est offerte par les approches de type Physics-Informed Neural Networks (PINN). Les PINN sont entraînés à satisfaire les équations du modèle en minimisant les résidus des EDP. Cependant, les PINN classiques posent plusieurs défis : besoin d'un grand nombre de points de collocation, difficultés de convergence et temps d'apprentissage longs. De plus, une nouvelle architecture PINN doit être construite pour chaque condition aux limites et condition initiale différente, ce qui limite leur applicabilité.
Une approche plus pratique consiste à concevoir des réseaux neuronaux prenant les conditions initiales et aux limites comme entrées, donnant ainsi naissance aux opérateurs neuronaux qui apprennent directement le schéma numérique. Les modèles de champ de phase offrent un autre avantage : ils peuvent être formulés comme un problème variationnel, impliquant la minimisation d'une fonctionnelle. Ceci est exploité par la méthode Deep Ritz, une technique basée sur les RN qui utilise l'apprentissage profond pour approcher les solutions en minimisant une fonctionnelle d'énergie, plutôt qu'en résolvant directement les EDP comme le font les PINN. Cette stratégie s'est révélée plus efficace pour les EDP hautement non linéaires.
Nous avons récemment appliqué une approche Deep Ritz dans une nouvelle architecture d'opérateur neuronal de type réaction-diffusion pour les équations de champ de phase. Nous avons montré que cette architecture surpasse les modèles RN standards pour l'équation d'Allen-Cahn ainsi que pour un modèle de solidification dendritique, applicable aux conditions des procédés métallurgiques.
Les objectifs de cette thèse de doctorat sont d'étendre les approches développées afin de :
• améliorer la précision des approximations des solutions des EDP grâce à une architecture de RN adaptée ou plus sophistiquée ;
• améliorer la généralisation des modèles RN afin d'obtenir des prédictions robustes hors du domaine d'apprentissage ;
• étendre les approches Deep Ritz RN à d'autres modèles de champ de phase incluant divers couplages multiphysiques ;
• développer des approches RN pour les formulations à interface abrupte des transformations de phase, comme le problème de Stefan ;
développer des approches RN similaires pour les modèles phénoménologiques de transformation de phase permettant de simuler des échelles spatiales et temporelles plus larges, comme GEM pour la solidification dendritique.
Keywords
Phase transformations, Materials, Phase-field modeling, Partial differential equations, Neural networks, Neural operators
Subject details
Phase transformations play an important role in elaboration of metal materials because they determine the structure and, consequently, the properties of the material. They occur, for example, during the additive manufacturing (3D printing) of a metal part. First, the molten liquid metal transforms to a crystalline solid structure and then further phase transformations occur in solid state. The properties of the part depend on the final phases, their size and shape. Phase-field models that describe phase transformations in materials [Kur21,Tou22] are formulated by reaction-diffusion partial differential equations (PDEs). This description is such that it couples the phase transformations to external fields (temperature, chemical composition, stresses, liquid flow, etc.). Today, such PDEs are generally solved numerically with classical discretization methods (finite difference, finite element, Fourier spectral, etc.). The multi-scale and nonlinear nature of phase transformation models, however, requires fine space and time discretizations, leading to long computation times and costly simulations. These times are prohibitive for scaling up to the process level and for conducting extensive parametric studies. Recently developed artificial intelligence methods for approximating solutions of PDEs have the potential to be much faster and thus to revolutionize the modeling of phase transformations. These methods employ surrogate models based on neural networks (NN). Most NN training processes in literature are data-driven [Din24], requiring substantial datasets and frequently resulting in instability in long-term predictions. Such approaches also suffer from limited generalization – ability to predict results that are outside the parameter range of the training set. Better generalization properties are provided by Physics Informed Neural Network (PINN) type approaches [Kar21]. PINNs are trained to satisfy the governing physical equations by minimizing the residuals of the PDEs. Plain vanilla PINNs pose several challenges: need for large number of collocation points, difficulties in achieving convergence, and long training times [Mat24]. Also, a new PINN needs to be constructed for different boundary and initial conditions, limiting their applicability. A more practical approach is to design neural networks that take initial and boundary conditions as inputs, giving rise to so-called neural operators that learn the numerical scheme directly. Phase-field models offer another advantage: they can be formulated as a variational problem, involving the minimization of a functional. This is leveraged by the Deep Ritz method [Yu18], a NN-based technique that uses deep learning to approximate solutions by minimizing an energy functional rather than directly solving the PDEs as in PINNs. This strategy has been shown to be more efficient for highly nonlinear PDEs [Mat24,Hua25]. We recently employed a Deep Ritz approach in a novel Reaction-Diffusion neural operator architecture [Hua25] for phase-field equations. We have shown that this architecture outperforms standard NN models for the Allen-Cahn equation as well as for a model of dendritic solidification [Kar98], applicable in conditions of metallurgical processes. The objectives of the PhD thesis are to extend the developed approaches to: • achieve better accuracy of the PDE solution approximations with a suitable or more sophisticated neural network architecture; • achieve better generality of the NN models in order to obtain robust out-of-distribution predictions; • extend the Deep Ritz NN approaches to further phase-field models including other multiphysics couplings [Cot21,Ghi24]; • develop NN approaches for sharp-interface formulations of phase transformations, such as the Stefan problem; • develop similar NN approaches for phenomenological phase transformation models that can simulate larger spatial and temporal scales, such as GEM [Via20] for dendritic solidification.
Profil du candidat
Le financement est ouvert aux excellents étudiants en physique, mathématiques appliquées, génie mécanique/chimique/des procédés ou d'autres disciplines. Nous recherchons des candidats ayant :
• Un diplôme de master dans une discipline pertinente.
• Une solide formation en méthodes numériques et en apprentissage automatique.
• De bonnes compétences en programmation informatique.
• Une maîtrise de la rédaction de rapports techniques et de la présentation.
• Une excellente capacité de travail en équipe, un sens de l'initiative et une aptitude à évoluer dans un environnement pluridisciplinaire.
• Une maîtrise de l'anglais ; des connaissances en français sont un avantage.
Candidate profile
The funding is open to excellent students from physics, applied mathematics, mechanical/chemical/process engineering or other disciplines. We are looking for candidates with:
• Master's degree in a relevant discipline.
• Solid background in numerical methods and machine learning.
• Good computer programming skills.
• Proficiency in technical report writing and presentation.
• Excellent capacity for teamwork, sense of initiative, and ability to work in a multidisciplinary environment.
• Fluent in English, some knowledge of French is beneficial.
Référence biblio
[Cot21] M. Cottura et al., Acta Materialia 212 (2021), 116851.
[Cuo22] S. Cuomo, et al., Journal of Scientific Computing 92 (2022), 88.
[Din24] R. Dingreville et al., Modelling Simul. Mater. Sci. Eng. 32 (2024), 065019
[Ghi24] F. Ghiglione et al., Journal of the Mechanics and Physics of Solids 187 (2024), 105628.
[Hua25] C.-K. Huang, L. Gagnon, B. Appolaire, M. Založnik, Neural Network Approximation of a Phase-Field Model for Dendritic Growth, in preparation, 2025.
[Kar98] A. Karma and W.-J. Rappel, Physical review E, 57 (1998), 4323.
[Kar21] G.E. Karniadakis et al., Nature Reviews Physics 3 (2021), 422.
[Kur21] W. Kurz, M. Rappaz, R. Trivedi, International Materials Reviews 66 (2021), 30.
[Mat24] R. Mattey, S. Ghosh, Gradient Flow Based Phase-Field Modeling Using Separable Neural Networks, arXiv:2405.06119v1 [cs.LG], 09 May 2024.
[Tou22] D. Tourret, H. Liu, J. LLorca, Progress in Materials Science 123 (2022), 100810.
[Via20] A. Viardin et al., Acta Materialia 199 (2020), 680.
[Yu18] B. Yu et al., Communications in Mathematics and Statistics, 6 (2018), 1.