Offre de thèse
ENACT Optimisation de modèles d'apprentissage automatique pour l'hydrogénation sélective du CO2 en méthanol catalysée par des composés inter-métalliques.
Date limite de candidature
01-10-2025
Date de début de contrat
01-10-2025
Directeur de thèse
GAUDRY Emilie
Encadrement
Florian BRIX
Type de contrat
école doctorale
équipe
DEPARTEMENT 1 - P2M : 102 - Surfaces et Spectroscopiescontexte
The Institut Jean Lamour (https://ijl.univ-lorraine.fr/en/laboratory/presentation) is a joint unit of the CNRS and Université de Lorraine (UMR 7198), leading fundamental and applied research activities materials science. It is a fundamental and applied research laboratory, covering materials, metallurgy, nanosciences, plasmas, surfaces and electronics in response to societal challenges such as energy, environment, the industry of the future, mobility, the preservation of resources and health. Its research work is carried out by 25 groups, supported by 8 technical platforms and 4 support services. The PhD student will join a team focused on surfaces and spectroscopy for various applications (https://ijl.univ-lorraine.fr/en/research-groups/surfaces-spectroscopies-and-modeling-group-supreme). The research activity will take place at the ARTEM campus in Nancy, which provides an inspiring environment in a city known for its rich cultural heritage and dynamic student life. At the European level, the PhD project will benefit from several networks -- the European Integrated Center for the Development of New metallic Alloys and Compounds (https://www.ecmetac.eu/), the International Lab between IJL and JSI (Josef Stefan Institute, Ljubljana, Slovenia, https://www.ijs.si/ijsw/JSI). The university also offers mobility grants for PhD students who would like to spend several months abroad (http://doctorat.univ-lorraine.fr/en/international/dream-en), thus contributing to the international dimension of the PhD. The selected candidate will have access to computational resources provided by GENCI (https://www.genci.fr/), EXPLOR (https://explor.univ-lorraine.fr/) and ENACT (https://cluster-ia-enact.ai/). This PhD offer is provided by the ENACT AI Cluster and its partners. Find all ENACT PhD offers and actions on https://cluster-ia-enact.ai/spécialité
Sciences des Matériauxlaboratoire
IJL - INSTITUT JEAN LAMOUR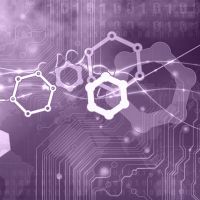
Mots clés
apprentissage automatique, Théorie de la Fonctionnelle de la Densité, CO2, surfaces, hydrogénation, composés intermétalliques
Détail de l'offre
L'hydrogénation sélective du CO2 en méthanol permet de convertir le dioxyde de carbone, un gaz à effet de serre majeur, en un produit à forte valeur ajoutée, offrant une alternative durable aux méthodes traditionnelles de synthèse du méthanol qui dépendent des gaz de synthèse et des technologies de reformage. Cependant, le catalyseur industriel utilisé dans le processus actuel (Cu/ZnO/Al2O3) souffre de désactivation et d'une faible sélectivité pour le méthanol dans les conditions d'hydrogénation du CO2. Des études récentes ont démontré que des stratégies d'alliage dans les composés inter-métalliques sp-TM (tels que Cu−Ga, Cu−Zn, Pd−Ga, Pd−In...) [1] peuvent améliorer la performance catalytique. Les méthodes computationnelles peuvent aider à explorer les catalyseurs à base de sp-TM comme alternatives aux catalyseurs industriels conventionnels. Cependant, des verrous ont limité les progrès dans cette direction, notamment (i) le manque d'informations détaillées concernant la structure du catalyseur et le site actif dans des conditions operando et (ii) la complexité de la modélisation de l'hydrogénation du CO2, ce qui entraîne des défis computationnels significatifs [2]. C'est là que les approches d'apprentissage automatique (ML) deviennent intéressantes, offrant un moyen d'accélérer considérablement l'optimisation de nouveaux systèmes catalytiques.
L'objectif principal de cette thèse est d'appliquer et d'adapter des modèles avancés d'apprentissage automatique pour prédire les propriétés catalytiques clés des systèmes à base de sp-TM. Nous nous concentrerons sur les réseaux neuronaux à propagation de messages (MPNN message passing neural networks) et les champs de forces machine learning (MLFF machine learning force fields), qui ont montré leur potentiel dans la modélisation des interactions interatomiques complexes avec une grande précision [3]. Ces modèles ont déjà fait leurs preuves dans des domaines connexes, mais leur application à la catalyse reste peu explorée. En entraînant ces modèles sur des ensembles de données catalytiques et en incorporant des informations clés spécifiques à la réaction, nous visons à affiner et étendre leur pouvoir prédictif. Les activités de recherche porteront également sur des techniques d'apprentissage actif pour optimiser l'efficacité du modèle en se concentrant sur les points de données les plus informatifs [4], réduisant ainsi le coût computationnel associé aux méthodes traditionnelles [5]. L'apprentissage actif permettra aussi l'amélioration itérative des modèles en sélectionnant les données qui améliorent au maximum leurs performances, facilitant des prédictions plus rapides et plus précises. Cette méthodologie aidera à prédire les barrières énergétiques et les mécanismes de réaction pour l'hydrogénation du CO2 avec une vitesse et une précision sans précédent, tout en examinant la transférabilité des modèles à de nouveaux systèmes catalytiques inexplorés. L'intégration des effets de température, souvent négligés mais cruciaux dans les processus catalytiques réels, sera également un aspect important de ce travail. En intégrant ces effets dans le cadre de l'apprentissage automatique, nous améliorerons l'applicabilité des modèles à des conditions de réaction plus réalistes.
Les résultats attendus de ce projet sont doubles : (1) réduire la dépendance aux calculs ab initio coûteux et chronophages, permettant ainsi une exploration plus rapide de systèmes chimiques complexes, et (2) faire progresser le domaine de l'apprentissage automatique pour la catalyse, ouvrant la voie au développement de matériaux catalytiques plus efficaces et durables. En tirant parti des dernières avancées en sciences computationnelles et en apprentissage automatique, cette thèse apportera une contribution significative à l'optimisation des systèmes catalytiques pour la conversion du CO2, soutenant ainsi la transition vers des processus industriels plus durables.
Keywords
machine learning, Density Functional Theory, CO2, surfaces, hydrogenation, intermetallic compounds
Subject details
The selective hydrogenation of CO2 to methanol presents an innovative way to convert CO2, a major greenhouse gas, into a high-demand, value-added product, providing a sustainable alternative to traditional methanol synthesis methods that rely on syngas and reforming technologies. However, the industrial catalyst used in the current process (Cu/ZnO/Al2O3) suffers from deactivation and low methanol selectivity under CO2 hydrogenation conditions. Recent studies have demonstrated that alloying strategies in sp-TM intermetallic compounds (such as Cu−Ga, Cu−Zn, Pd−Ga, Pd-In...), [1] can improve catalytic performance. Computational methods can help to explore sp-TM-based catalysts as alternatives to conventional industrial catalysts. But major challenges have limited progress in this direction, especially (i) the lack of detailed information concerning the catalyst structure and active site under operando conditions and (ii) the complexity of modeling CO2 hydrogenation, which leads to significant computational challenges.[2] This is where machine learning (ML) approaches become invaluable, offering a way to significantly accelerate the optimization of new catalytic systems. The core objective of this thesis is to apply and adapt advanced ML models to predict the key catalytic properties of sp-TM-based systems. We will focus on Message Passing Neural Networks (MPNNs) and Machine Learned Force Fields (MLFFs), which have shown promise in modeling complex interatomic interactions with high precision.[3] These models have already demonstrated success in related fields, but their application to catalysis remains underexplored. By training these models on catalytic datasets and incorporating key reaction-specific information, we aim to refine and extend their predictive power. Additionally, the research will employ active learning techniques to optimize model efficiency by focusing on the most informative data points,[4] thereby reducing the computational cost associated with traditional methods.[5] Active learning will also allow for the iterative improvement of models by selecting data that maximally improves their performance, facilitating faster and more accurate predictions. This methodology will help predict energy barriers and reaction mechanisms for CO2 hydrogenation with unprecedented speed and accuracy, while also examining the transferability of the models to new, unexplored catalytic systems. Incorporating temperature effects, which are often neglected but crucial in real-world catalytic processes, will also be an important aspect of this work. By integrating these effects into the machine learning framework, we will enhance the models' applicability to more realistic reaction conditions. The expected outcomes of this project are twofold: (1) reducing the reliance on costly and time-consuming ab initio calculations, thus enabling faster exploration of complex chemical systems, and (2) advancing the field of machine learning for catalysis, paving the way for the development of more efficient and sustainable catalytic materials. By leveraging the latest advancements in computational science and machine learning, this thesis will make a significant contribution to the optimization of catalytic systems for CO2 conversion, ultimately supporting the transition to more sustainable industrial pocesses
Profil du candidat
Nous invitons les candidats ayant une solide formation en physique computationnelle, chimie, science des matériaux ou équivalent, avec de bonnes compétences en analyse statistique de données, à postuler et à nous rejoindre dans cette recherche passionnante et impactante. Une expérience préalable en chimie computationnelle pour la catalyse, en simulations de dynamique moléculaire, et en calculs de structure électronique ab-initio sera considérée comme un atout supplémentaire. La candidature doit inclure une déclaration d'intérêt de recherche, un CV avec les noms de deux personnes à contacter pour des recommandations, une liste de publications et d'autres documents pertinents, si disponibles. Ce projet offre une excellente opportunité de travailler à la pointe de la catalyse computationnelle tout en développant des modèles d'apprentissage automatique de pointe avec des applications réelles pour la durabilité environnementale.
Candidate profile
We invite candidates with a strong background in Computational Physics, Chemistry, Material Science or equivalent, with a good background in statistical data analysis, to apply and join us in this exciting and impactful research. Previous experience in computational chemistry for catalysis, molecular dynamics simulations, ab-initio electronic structure calculations will be considered as an extra merit. The application should include a statement of research interest, a CV with the names of two people to contact for a recommendation, a list of publications and other relevant materials, if available. This project provides an excellent opportunity to work at the forefront of computational catalysis while developing cutting-edge ML models with real-world applications in environmental sustainability.
Référence biblio
1 E. Gaudry et al., Catalytic activation of a non-noble intermetallic surface through nanostructuration under hydrogenation conditions revealed by atomistic thermodynamics, J. Mater. Chem. A 8 (2020) 7422
2 F. Brix, V. Desbuis, L. Piccolo, É. Gaudry, Tuning Adsorption Energies and Reaction Pathways by Alloying: PdZn versus Pd for CO2 Hydrogenation to Methanol, The Journal of Physical Chemistry Letters 2020 11 (18), 7672-7678
3 I. Batatia et al., Mace : Higher order equivariant message passing neural networks for fast and accurate force fields. In Advances in Neural Information Processing Systems 35 (NeurIPS 2022), 2022. Available at : https://github.com/ACEsuit/mace.
4 N. Boulangeot, F. Brix, F. Sur, E. Gaudry, Hydrogen, Oxygen, and Lead Adsorbates on Al13Co4(100): Accurate Potential Energy Surfaces at Low Computational Cost by Machine Learning and DFT-Based Data. J Chem Theory Comput. 20 (2024) 7287
5 Jun Luo et al., Automatic identification of slip pathways in ductile inorganic materials by combining the active learning strategy and neb method. npj Computational Materials, 11 (2025) 41; Lars L Schaaf et al., Accurate energy barriers for catalytic reaction pathways : an automatic training protocol for machine learning force fields. npj Computational Materials, 9 (2023)