Offre de thèse
Analyse quantitative d'images d'angiographie par rayons X pour les AVC ischémiques
Date limite de candidature
15-05-2024
Date de début de contrat
01-10-2024
Directeur de thèse
KERRIEN Erwan
Encadrement
Weekly meetings with supervisors. Regular updates with clinical partners. Easy access to medical facilities and data when needed.
Type de contrat
école doctorale
équipe
MAGRITcontexte
Ischemic stroke is a major cause of disability and death worldwide. The occlusion of blood vessels results in a potentially large area of the brain to be oxygen deprived. Recent clinical trials have demonstrated Endovascular Thrombectomy (EVT) to be highly effective, which has led to its widespread adoption in clinical routine. In the vast majority of cases, EVT is successful in the sense that recanalization is observed : the blood flow is restored in the previously blocked blood vessels, which can be assessed using Digital Subtracted Angiography (DSA). However, even when treatment has been performed within the recommended 3 hour-window after stroke onset, it is estimated that about 50% of successful recanalizations do not result in a restored perfusion in the downstream brain tissues. This phenomenon is known as no-reflow. But the reperfusion status cannot be readily assessed in DSA images, requiring a clinical evaluation of the outcome. Trustful quantitative tools are missing to assess the level of reperfusion after EVT, better predict potential difficulties for this reperfusion to be restored for a patient, and help the medical community better understand the underlying mechanisms of successful reperfusion.spécialité
Informatiquelaboratoire
LORIA - Laboratoire Lorrain de Recherche en Informatique et ses Applications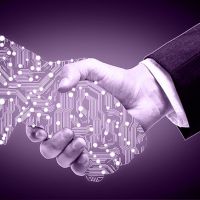
Mots clés
deep learning, medical imaging, video analysis, radiomics
Détail de l'offre
L'accident vasculaire cérébral (AVC) ischémique est une des causes majeures de handicap et de décès dans le monde. Dans la grande majorité des cas, le traitement endovasculaire est réussi dans le sens où le flux sanguin est restauré dans les vaisseaux sanguins précédemment obstrués. Cependant, on estime qu'environ 50 % des recanalisations réussies ne se traduisent pas par une restauration de la perfusion dans les tissus cérébraux en aval. Ce phénomène est connu sous le nom de 'no-reflow'. Les médecins ne savent pas l'évaluer pendant l'intervention aujourd'hui. L'objectif de ce projet est de tirer parti des récents modèles d'apprentissage profond pour développer des outils d'analyse d'images et concevoir des métriques appropriées pour permettre une évaluation immédiate de la reperfusion basée sur les images d'angiographie par rayons X acquises avant, pendant et après l'intervention.
Keywords
apprentissage profond, imagerie médicale, analyse vidéo, radiomiques
Subject details
Ischemic stroke is a major cause of disability and death worldwide. In the vast majority of cases, endovascular treatment is successful and the blood flow is restored in the previously blocked blood vessels. However, it is estimated that about 50% of such successful treatments do not result in a restored perfusion in the downstream brain tissues. This phenomenon is known as no-reflow. Clinicians have no means to evaluate the reperfusion status today. The objective of this project is to leverage recent deep learning models to develop image analysis tools and design appropriate metrics to enable an immediate assessement of reperfusion based on X-ray angiography images acquired before, during and after the intervention.
Profil du candidat
Nous recherchons des candidats possédant un excellent niveau de connaissances en vision par ordinateur et science des données, mais peuvent également postuler les personnes avec un profil académique solide dans des champs disciplinaires plus larges comme l'informatique, les mathématiques appliquées ou l'ingénierie biomédicale.
Le ou la candidate sera diplômée d'un Master (ou équivalent, par exemple école d'ingénieur) en vision par ordinateur, science des données, informatique, mathématiques appliquées ou ingénierie biomédicale.
Il est essentiel de parler couramment l'anglais, ou couramment le français avec un excellent niveau en anglais. Le développement logiciel du projet se fera en priorité en python avec PyTorch, Une solide expérience pratique de ce
langage et framework est donc attendue.
Il est attendu du candidat ou de la candidate un esprit ouvert à d'autres disciplines scientifiques, un goût pour la recherche clinique et les applications médicales, une aisance dans la communication et un vif désir d'apprendre et de participer à la dynamique d'un projet scientifique.
Candidate profile
We are looking for candidates with excellent skills and knowledge in computer vision and data science. A background in computer science and/or applied mathematics will be an advantage.
The applicant should hold a Master's degree (or equivalent, e.g. Engineering degree) in computer vision, data science, computer science or applied mathematics.
Fluency in English, or fluency in French with excellent level in English are mandatory to fill the position. Software development will primarily be realized in python using PyTorch. A solid practical experience in these language and framework is expected.
An open mind towards connected scientific fields, a taste for clinical research and medical applications, good communication skills, and a strong desire to learn and participate in a scientific project dynamics are expected from the candidate.
Référence biblio
[Su21] Su, R. et al. (2021). 'autoTICI: Automatic Brain Tissue Reperfusion Scoring on 2D DSA Images of Acute Ischemic Stroke Patients,' in IEEE Transactions on Medical Imaging, vol. 40, no. 9, pp. 2380-2391. https://doi.org/10.1109/TMI.2021.3077113.
[Haouchine21] Haouchine, N. et al. (2021). 'Estimation of High Framerate Digital Subtraction Angiography Sequences at Low Radiation Dose'. In: de Bruijne, M., et al. Medical Image Computing and Computer Assisted Intervention – MICCAI 2021. MICCAI 2021. Lecture Notes in Computer Science(), vol 12906. Springer, Cham. https://doi.org/10.1007/978-3-030-87231-1_17
[Mittmann22] Mittmann BJ, et al. (2022). 'Deep learning-based classification of DSA image sequences of patients with acute ischemic stroke'. Int J Comput Assist Radiol Surg. 17(9):1633-1641. https://doi.org/10.1007/s11548-022-02654-8
[Baur24] Baur, K, et al. (2024). 'Spatiotemporal Disentanglement of Arteriovenous Malformations in Digital Subtraction Angiography.' arXiv preprint arXiv:2402.09636.
[Kosior19] Kosior JC, et al. (2019). 'Exploring Reperfusion Following Endovascular Thrombectomy'. Stroke. 50(9):2389-2395. https://doi.org/10.1161/STROKEAHA.119.025537.
[van't Hof98] van't Hof, AW et al. (1998). 'Angiographic assessment of myocardial reperfusion in patients treated with primary angioplasty for acute myocardial infarction: myocardial blush grade'. Zwolle Myocardial Infarction Study Group. Circulation. 97:2302-6.
[Consoli23] Consoli, A et al. (2023). 'Unfavorable clinical outcomes in patients with good collateral scores following endovascular treatment for acute ischemic stroke of the anterior circulation: The UNCLOSE study'. Interventional Neuroradiology. https://doi.org/10.1177/15910199231212519.