Offre de thèse
Causalité, processus ponctuels et application au mixed marketing
Date limite de candidature
01-04-2024
Date de début de contrat
01-10-2024
Directeur de thèse
CLAUSEL Marianne
Encadrement
Encadrement avce A. Leite
Type de contrat
école doctorale
équipe
PROBAS STATScontexte
Ce projet de thèse est partie intégrante d'une collaboration entre Pernod Ricard et l'équipe TAU d'INRIA Saclaspécialité
Mathématiqueslaboratoire
IECL - Institut Elie Cartan de Lorraine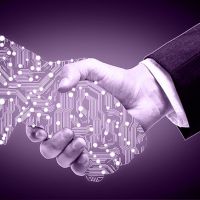
Mots clés
causalité, processus ponctuels
Détail de l'offre
L'utilisation de techniques d'apprentissage automatique dans le contexte industriel a prouvé son utilité pour une variété d'applications. Dans le domaine du marketing, une litt´erature abondante, des preuves de concepts et des projets pilotes ont mis en évidence l'importance de ces méthodes dans la modélisation de la relation entre l'allocation (publicité, distribution, promotion, produit) et les ventes. La quantification de l'impact de ces dépenses représente une opportunité pour l'amélioration de la pertinence des actifs publicitaires, l'optimisation des allocations des ressources financières et de l'amélioration du processus décisionnel. Toutefois, ce potentiel demeure sous exploité en raison de la complexité du problème sous jacent.
Cette thèse abordera certains de ces défis dans le contexte de la modélisation du mix marketing chez Pernod Ricard, en tenant compte des contraintes opérationnelles et environnementales. Nous nous concentrerons particulièrement sur
l'efficacité à long terme des campagnes marketing qui est une question stratégique pour Pernod Ricardpr
Keywords
causality, point process
Subject details
The use of machine learning techniques in the industrial context has proven its usefulness for a variety of applications. of applications. In the field of marketing, an abundance of literature, proofs of concept and pilot projects have demonstrated the importance of these methods in modelling the relationship between allocation (advertising, distribution, promotion, product) and sales, distribution, promotion, product) and sales. Quantifying the impact of these expenses represents an opportunity for for improving the relevance of advertising assets, optimizing the allocation of financial resources and improving the quality of advertising. improving the decision-making process. However, this potential remains under-exploited due to the complexity of the underlying problem. underlying problem. This thesis will address some of these challenges in the context of marketing mix
Profil du candidat
Master IA
Candidate profile
AI Master
Référence biblio
[1] C. K. Assaad, E. Devijver, and E. Gaussier. “Survey and evaluation of causal discovery methods for time series”.
In: Journal of Artificial Intelligence Research 73 (2022), pp. 767–819 (cit. on p. 3).
[2] P. Cain. “Modelling short-and long-term marketing effects in the consumer purchase journey”. In: International
Journal of Research in Marketing 39.1 (2022) (cit. on p. 2).
[3] M. Dekimpe and D. Hanssens. “Consumer Attitude Metrics for Guiding Marketing Mix Decisions”. In: Marketing
Science 14.1 (1995), pp. 1–21 (cit. on p. 2).
[4] P. Feldwick. “What is brand equity anyway, and how do you measure it?” In: International Journal of Market
Research 38.2 (1996) (cit. on p. 2).
[5] F. Gamboa, A. Janon, T. Klein, and A. Lagnoux. “Sensitivity analysis for multidimensional and functional outputs”.
In: Electronical Journal of Statististics 8.1 (2014), pp. 575–603 (cit. on p. 3).
[6] D. Hanssens, K. Pauwels, S. Srinivasan, M. Vanhuele, and G. Yildirim. “Consumer Attitude Metrics for Guiding
Marketing Mix Decisions”. In: Marketing Science 14 (2014) (cit. on p. 2).
[7] A. G. Hawkes. “Point spectra of some mutually exciting point processes”. In: Journal of the Royal Statistical Society:
Series B (Methodological) 33.3 (1971), pp. 438–443 (cit. on p. 4).
[8] Y. Jin, Y. Wang, Y. Sun, D. Chan, and J. Koehler. Bayesian Methods for Media Mix Modeling with Carryover and
Shape Effects. Tech. rep. Google Inc., 2017 (cit. on p. 1).
[9] S. Makridakis, E. Spiliotis, and V. Assimakopoulos. “The M4 Competition: 100,000 time series and 61 forecasting
methods”. In: International Journal of Forecasting 36.1 (2020). M4 Competition, pp. 54–74 (cit. on p. 3).
[10] S. Makridakis, E. Spiliotis, and V. Assimakopoulos. “The M5 competition: Background, organization, and
implementation”. In: International Journal of Forecasting (2021) (cit. on p. 3).
[11] K. E. Mokhtari, B. P. Higdon, and A. Ba¸sar. “Interpreting financial time series with SHAP values”. In: 29th Annual
International Conference on Computer Science and Software Engineering. 2019, pp. 166–172 (cit. on p. 3).
[12] R. Moraffah, P. Sheth, M. Karami, A. Bhattacharya, Q. Wang, A. Tahir, A. Raglin, and H. Liu. “Causal inference
for time series analysis: Problems, methods and evaluation”. In: Knowledge and Information Systems 63 (2021),
pp. 3041–3085 (cit. on p. 3).
[13] J. Peters, D. Janzing, and B. Sch¨olkopf. Elements of causal inference. The MIT Press, 2017 (cit. on p. 3).
[14] O. Shchur, A. C. T¨urkmen, T. Januschowski, and S. G¨unnemann. “Neural temporal point processes: A review”. In:
arXiv:2104.03528 (2021) (cit. on p. 3).
[15] A. Vaswani, N. Shazeer, N. Parmar, J. Uszkoreit, L. Jones, A. N. Gomez, L. Kaiser, and I. Polosukhin. “Attention
is all you need”. In: Advances in neural information processing systems 30 (2017) (cit. on p. 3).
[16] J. Yan. “Recent advance in temporal point process: from machine learning perspective”. In: SJTU Technical Report
(2019) (cit. on p. 3)