Offre de thèse
Diagnostic et pronostic intelligents des arythmies cardiaques basés sur LLM : Application aux arythmies cardiaques à partir d'un système de membrane biomatériau de nouvelle génération - LLMARC
Date limite de candidature
10-07-2025
Date de début de contrat
01-10-2025
Directeur de thèse
THEILLIOL Didier
Encadrement
Co-Encadrant : Dr. Mayank Shekar JHA - mayank-shekhar.jha@univ-lorraine.fr - CRAN
Type de contrat
école doctorale
équipe
CID - Contrôle - Identification - Diagnosticcontexte
As part of the ENACT AI Cluster project, the research topic entitled 'Large Language Model (LLM) based Intelligent Diagnostics and Prognostics of Cardiac Arrhythmia: Application to Cardiac Arrhythmia from a New Generation Bio-Prosthesis Membrane System - LLMARC' is proposed by faculty members from two laboratories, CRAN and Institut Jean Lamour (IJL) at the Université de Lorraine, in which the Banook Group is involved by providing quantitative and qualitative data and analyzing the obtained results. This research topic is co-directed by Pr D. THEILLIOL CRAN, Dr JP JEHL and Dr MS JHA CRAN. These research activities are based, on the one hand, on the recent development of a new electrocardiogram measurement system using instrumented membranes that adapt to the patient's condition outside the hospital, developed by Nano-Bio Materials for Life team at the Institut Jean Lamour UMR 7198, CNRS, and on the other hand, on the expertise in the field of diagnostics and prognostics of continuous dynamic systems by CRAN UMR 7039, CNRS members within the Control Identification Diagnostic Department. As part of the research activities dedicated to this thesis topic, the Banook Group provides its anonymized and textual database. The Banook Group also brings its expertise in validating the results obtained from the developed methods through its representative, Dr. M. DIAW (Diaw 2024), who is involved in supporting this research work. It should be noted that this research and development work is at Technology Readiness Levels 3/4 (3 - Experimental proof of concept / 4 - Technology validated in lab) and is part of the continuation of the Innovative Development of Medical Repair and Assistance Devices - DREAM project led by the Institut Jean Lamour in cooperation with the Banook Group.spécialité
Automatique, Traitement du signal et des images, Génie informatiquelaboratoire
CRAN - Centre de Recherche en Automatique de Nancy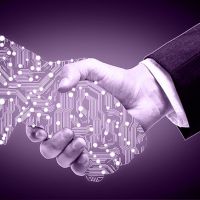
Mots clés
LLM, Diagnostic, Pronos, biomateriaux, Electrocardiogramme
Détail de l'offre
Les avancées récentes en IA, en particulier l'émergence des LLMs basés sur des architectures de transformateurs, ont catalysé des approches innovantes dans la détection des formes d'onde ECG et la prédiction des arythmies. Traditionnellement, ce domaine a été dominé par les techniques de traitement du signal et d'apprentissage profond conventionnelles. Désormais, ces méthodes conventionnelles sont surpassées par des stratégies basées sur les LLM qui tirent parti de la capacité des transformateurs à modéliser les dépendances à long terme dans les données séquentielles. Une innovation clé réside dans l'adaptation des LLM au domaine biomédical. Lorsqu'ils sont ajustés sur des données spécifiques au domaine, les LLM atteignent des niveaux de performance qui rivalisent avec les modèles d'apprentissage profond traditionnels. Les évaluations comparatives ont montré que des modèles comme GPT-4 présentent une compréhension améliorée de la terminologie médicale et des capacités interprétatives supérieures pour les données ECG complexes. Les systèmes multimodaux comme ECG-Chat intègrent les données de forme d'onde avec les informations cliniques textuelles. Cette synergie améliore la fiabilité de la prédiction des arythmies. Malgré ces développements prometteurs, des défis significatifs persistent dans le déploiement de l'analyse ECG basée sur les LLM. L'hétérogénéité des enregistrements ECG—démographie des patients, spécifications des dispositifs et conditions d'enregistrement—reste un obstacle critique. Les ensembles de données standardisés ne parviennent souvent pas à capturer toute la variabilité du monde réel, en particulier lorsque de nouvelles membranes ECG personnalisées sont introduites, nécessitant une caractérisation mécanique étendue. De plus, les modèles actuels sont généralement entraînés sur des données limitées et homogènes, ce qui restreint leur capacité à détecter des tendances subtiles essentielles pour le diagnostic et le pronostic précoces. L'intégration des données longitudinales des patients, y compris les dossiers historiques et les notes cliniques, ajoute une complexité supplémentaire à l'entraînement et à l'ajustement des modèles, en particulier dans divers sous-groupes de patients. En s'inspirant du domaine de la gestion des pronostics et de la santé (PHM)—où la fusion de données de capteurs et l'apprentissage profond ont été utilisés avec succès pour prédire les pannes de machines et estimer la durée de vie restante—cette recherche explore des méthodes analogues pour les diagnostics cardiaques. En transférant des techniques du PHM industriel, telles que la fusion de données multimodales et les protocoles d'apprentissage adaptatif, l'objectif est de développer des modèles basés sur les LLM qui offrent des diagnostics précoces et des pronostics personnalisés en cardiologie. Ainsi l'étude proposée dans le cadre de cette thèse est structurée autour de trois grands objectifs scientifiques : (1) le développement de diagnostics ECG basés sur LLMs multimodaux (LM2) qui utilisent des méthodes basées sur des transformateurs pour isoler les anomalies de forme d'onde en ligne en intégrant des signaux bruts avec des métadonnées supplémentaires des patients ; (2) la formulation de modèles de pronostic basés sur les LM2 qui prévoient l'évolution des conditions cardiaques en capturant les dépendances temporelles à long terme à partir de flux de données multimodales ; et (3) la conception de stratégies d'adaptation de domaine et d'ajustement pour adapter ces modèles à l'hétérogénéité inhérente aux différentes populations de patients et configurations de capteurs. En fusionnant les forces du traitement du langage naturel avec l'analyse des signaux biomédicaux, cette recherche vise à proposer des approches novatrices pour la détection et la prédiction des arythmies, améliorant ainsi les résultats cliniques en soins cardiovasculaires.
Keywords
Large Language Model, Diagnosis, Prognosis, Bio-prosthesis, Electrocardiogram
Subject details
Recent advancements in AI, particularly the emergence of large language models (LLMs) based on transformer architectures, have catalyzed innovative approaches in ECG waveform detection and arrhythmia prediction. Traditionally, this domain has been dominated by conventional signal processing and deep learning techniques—such as convolutional neural networks and recurrent neural networks —which require extensive feature engineering and often struggle with noise and inter-patient variability. Now, these conventional methods are being surpassed by LLM-based strategies that leverage the transformer's capacity to model long-range dependencies in sequential data. A key innovation lies in the adaptation of LLMs to the biomedical realm through the tokenization of continuous ECG signals to capture critical morphological and temporal characteristics. When fine-tuned on domain-specific data, LLMs not only generate comprehensive diagnostic narratives that blend signal analysis with clinical context but also achieve performance levels that rival traditional deep learning models. Comparative evaluations have shown that models like GPT-4 exhibit an improved understanding of medical terminology and superior interpretative abilities for complex ECG data. Multimodal systems like ECG‐Chat integrate waveform data with textual clinical information through contrastive learning frameworks, ensuring that signal features are accurately aligned with corresponding clinical terminologies. This synergy not only improves arrhythmia prediction reliability but also automates the generation of detailed diagnostic reports, a capability that could substantially streamline clinical workflows in high-pressure environments. Despite these promising developments, significant challenges persist in the deployment of LLM-based ECG analysis. The heterogeneity of ECG recordings—patient demographics, device specifications, and recording conditions—remains a critical hurdle. Standardized datasets, often fail to capture the full spectrum of real-world variability, particularly when new customized ECG membranes are introduced, which necessitate extensive mechanical characterization. Moreover, current models are typically trained on limited and homogeneous data, restricting their ability to detect subtle trends vital for early diagnosis and prognostics. Integrating longitudinal patient data, including historical records and clinical notes, adds further complexity to model training and fine-tuning, especially across diverse patient subgroups. Drawing inspiration from the Prognostics and Health Management (PHM) domain—where sensor data fusion and deep learning have been successfully employed to predict machinery failures and estimate Remaining Useful Life—this research explores analogous methods for cardiac diagnostics. By transferring techniques from industrial PHM, such as multi-modal data fusion and adaptive learning protocols, the aim is to develop LLM-based models that offer early diagnostics and personalized prognostics in cardiology. To achieve this, the study is structured around three major scientific objectives: (1) the development of large multimodal language models (LM2)-based ECG diagnostics that utilize transformer-based methods to isolate waveform anomalies on line by integrating raw signals with supplementary patient metadata; (2) the formulation of LM2-based prognostic models that forecast the evolution of cardiac conditions by capturing long-range temporal dependencies from multi-modal data streams; and (3) the design of domain adaptation and fine-tuning strategies to tailor these models to the heterogeneity inherent in different patient populations and sensor configurations. By merging the strengths of natural language processing with biomedical signal analysis, this research aims to propose novel approaches for arrhythmia detection and prognostication, ultimately improving clinical outcomes in cardiovascular care.
Profil du candidat
Les candidats doivent être titulaires d'un master en Automatique / Informatique (Traitement du Langage Naturel) ou en Mathématiques Appliquées et démontrer un excellent dossier académique ainsi que la capacité à mener des recherches indépendantes.
Une solide formation en systèmes dynamiques, en bases des réseaux de neurones profonds, en apprentissage automatique ainsi qu'une bonne connaissance de l'environnement Matlab/Simulink et de Python est requise. Des connaissances en ingénierie mécanique seraient appréciées.
Candidate profile
Candidates should have a master's degree on Automatic Control / Computer Sciences (Natural Language Processing) or Applied Mathematics and demonstrate excellent academic record and the ability to do independent research.
A strong background in dynamical systems, basics of Deep neural networks, Machine learning as well as a good knowledge of the Matlab/Simulink environment and Python is required, knowledge of mechanical engineering would be appreciated.
Référence biblio
* Supervisors Reference
Alsaif, K. M., Albeshri, A. A., Khemakhem, M. A., & Eassa, F. E. (2024). Multimodal Large Language Model-Based Fault Detection and Diagnosis in Context of Industry 4.0. Electronics, 13(24), 4912.
* Buciakowski, M., Witczak, M., Mrugalski, M., & Theilliol, D. (2017), A quadratic boundedness approach to robust DC motor fault estimation. Control Engineering Practice, Elsevier, 2017, 66, pp.181-194.
Chen, T., Zhang, J., & Wang, H. (2021). Remaining Useful Life Prediction of Aero-Engines Using a Transformer-Based Network. IEEE Access, 9, 12345–12355.
* De Beaulieu, M.H., Jha, M.S., Garnier, H., & Cerbah, F., (2024), Remaining Useful Life prediction based on physics-informed data augmentation, Reliability Engineering & System Safety, Volume 252.
Devlin, J., Chang, M.-W., Lee, K., & Toutanova, K. (2018). BERT: Pre-training of Deep Bidirectional Transformers for Language Understanding.
Diaw M., Analyse approfondie de l'ECG pour l'extraction de biomarqueurs de la dispersion mécanique cardiaque, Université de Lorraine, ED IAEM, Septembre 2024
El Hassani, I., Masrour, T., Kourouma, N., Motte, D., & Tavčar, J. (2024). Integrating Large Language Models for Improved Failure Mode and Effects Analysis (FMEA): A Framework and Case Study. Proceedings of the Design Society, 4, 2019–2028.
Fink, O., Wang, Q., Svensen, M., Dersin, P., Lee, W. J., & Ducoffe, M. (2020). Potential, challenges and future directions for deep learning in prognostics and health management applications. Engineering Applications of Artificial Intelligence, 92, 103678.
Gendler, M., Nadkarni, G. N., Sudri, K., Cohen-Shelly, M., Glicksberg, B. S., Efros, O., Soffer, S., & Klang, E. (2024). Large Language Models in Cardiology: A Systematic Review. doi:10.1101/2024.09.01.24312887
George B. Moody and Roger G. Mark. Mit-bih arrhythmia database, 1992. URL https:// physionet.org/physiobank/database/mitdb/.
Goldberger Ary L., Amaral Luis A. N., Glass Leon, Hausdorff Jeffrey M., Ivanov Plamen Ch., Mark Roger G., Mietus Joseph E., Moody George B., Peng Chung-Kang, and Stanley H. Eugene (2000). Physiobank, physiotoolkit, and physionet. Circulation, 101(23):e215–e220.
* Guibert, B., Poerio, A., Nicole, L., Budzinski, J., Leroux, M.M., Fleutot, S., Ponçot, M., Cleymand, F., Bastogne, T., & Jehl, J.P., (2025) Customizable patterned membranes for cardiac tissue engineering: a model-assisted design method, Journal of the Mechanical Behavior of Biomedical Materials, Vol. 162.
Guo, M., Zhou, Y., & Tang, S. (2024). Multimodal Models for Comprehensive Cardiac Diagnostics via ECG Interpretation. In Proceedings of the IEEE BIOMED Conference. doi:10.1109/bibm62325.2024.10822122
Gušev, M., Tudjarski, S., & Kanoulas, E. (2024). Heart Language Model: Training and Fine-tuning Transformer-Based Foundation Models With Electrocardiogram Annotations. doi:10.21203/rs.3.rs-4575811/v1
Hu, Y., Miao, X., Si, Y., Pan, E., & Zio, E. (2022). Prognostics and health management: A review from the perspectives of design, development and decision. Reliability Engineering & System Safety, 217, 108063.
Jardine, A. K., Lin, D., & Banjevic, D. (2006). A review on machinery diagnostics and prognostics implementing condition-based maintenance. Mechanical Systems and Signal Processing, 20(7), 1483–1510.
* Jehl, J.P., Poerio, A., Fleutot, S., Lovera-Leroux & M., Cleymand, F., (2022) Development of a cardiac bio-prosthesis, Tissue Engineering Part A, vol. 28.
* Jehl, J.P., Instrumented indentation of cardiac tissue: towards the development of a bio-prosthesis, Thèse Université de Lorraine, February 2021.
* Jha M.S., Garnier H., & Theilliol, D., (2023), Redundancy-Aware Physics Informed Neural Networks (R-PINNs) based Learning of Nonlinear Algebraic Systems with Non-Measurable States, 62nd IEEE Conference on Decision and Control, Dec. 13-15, Singapore.
Jie‐Lin Qiu, W. Han, J. Zhu, M. Xu, M. Rosenberg, E. Liu, D. Weber, & D. Zhao. (2023). Transfer Knowledge from Natural Language to Electrocardiography: Can We Detect Cardiovascular Disease Through Language Models? Findings, 442–453. doi:10.48550/arXiv.2301.09017
Kalpana, A. M. (2023). HybDeepNet: ECG Signal Based Cardiac Arrhythmia Diagnosis Using a Hybrid Deep Learning Model. Information Technology and Control. doi:10.5755/j01.itc.52.2.33302
* Kanso, S., Contributions to Safe Reinforcement Learning and Degradation Tolerant Control Design, Thèse, Université de Lorraine, December 2024.
* Kanso, S., Jha, M.S., & Theilliol, D., (2024). Off-policy model-based end-to-end safe reinforcement learning. Int J Robust Nonlinear Control, vol. 34, Issue 4. pp. 2313-2987
* Karimi Pour, F., Theilliol, D., Puig, V., & Cembrano, G., (2020) Health-aware control design based on remaining useful life estimation for autonomous racing vehicle. ISA Transactions, vol. 113, pp. 196-209.
Li, W., Zhang, X., & Li, Y. (2021). Transformer-Based Neural Network for Prognostics of Complex Systems. IEEE Transactions on Industrial Informatics, 17(5), 3507–3517.
Li, Y. F., Wang, H., & Sun, M. (2024). ChatGPT-like Large-scale Foundation Models for Prognostics and Health Management: A Survey and Roadmaps. Reliability Engineering & System Safety, 243, 109850.
Lukens, S., McCabe, L. H., Gen, J., & Ali, A. (2024, November). Large Language Model Agents as Prognostics and Health Management Copilots. Annual Conference of the PHM Society, 16(1).
Mohamed, H. K., & Abdelhafeez, A. (2022). Unveiling the Power of Convolutional Networks: Applied Computational Intelligence for Arrhythmia Detection from ECG Signals. International Journal of Artificial and Advanced Computing, 1(2), 63–72. doi:10.54216/ijaaci.010205
Novak, A., Rode, F. W., Lisicic, A., Nola, I. A., Zeljković, I., & Manola, Š. (2023). The Pulse of Artificial Intelligence in Cardiology: A Comprehensive Evaluation of State-of-the-Art Large Language Models for Potential Use in Clinical Cardiology. medRxiv. doi:10.1101/2023.08.08.23293689
* Poerio, A., Guibert, B., Leroux, M.M., Mano, J.F., Cleymand, F., & Jehl, J.P. (2023) Mechanical Characterization of 3D-Printed Patterned Membranes for Cardiac Tissue Engineering: An Experimental and Numerical Study, Biomedicines, vol. 11, n°3.
Rezaeianjouybari, B., & Shang, Y. (2020). Deep learning for prognostics and health management: State of the art, challenges, and opportunities. Measurement, 163, 107929.
Strodthoff, N., Wagner, P., Schaeffter, T., & Samek, W. (2020). Deep learning for ECG analysis: Benchmarks and insights from PTB-XL. IEEE journal of biomedical and health informatics, 25(5), 1519-1528.
* Suh, S., Mittal, D.A., Bello, H., Zhou, B., Jha, M.S., & P.Lukowicz (2024). Remaining useful life prediction of Lithium-ion batteries using spatio-temporal multimodal attention networks, Heliyon, Volume 10, Issue 16.
Sumalatha, U., Prakasha, K. K., Prabhu, S., & Nayak, V. C. (2024). Deep learning applications in ecg analysis and disease detection: An investigation study of recent advances. IEEE Access.
Susto, G. A., Schirru, A., Pampuri, S., McLoone, S., & Beghi, A. (2015). Machine learning for predictive maintenance: A multiple classifier approach. IEEE Systems Journal, 9(3), 849–857.
* Thuillier, J., Jha, M.S., Le Martelot, S., & Theilliol, D. (2024). Prognostics Aware Control Design for Extended Remaining Useful Life: Application to Liquid Propellant Reusable Rocket Engine. International Journal of Prognostics and Health Management, vol. 15, n°1.
Torres, J. R., De Los Rios, K., & Salinas Padilla, M. Á. (2020). Cardiac Arrhythmias Identification by Parallel CNNs and ECG Time-Frequency Representation. Computing in Cardiology Conference, 1–4. doi:10.22489/CINC.2020.456
Tsai, Y.-H. H., Liang, P. P., Zadeh, A., & Morency, L.-P. (2019). Multimodal Transformer for Unaligned Multimodal Language Sequences. Proceedings of the 57th Annual Meeting of the Association for Computational Linguistics.
Vaswani, A., Shazeer, N., Parmar, N., et al. (2017). Attention is All You Need. Advances in Neural Information Processing Systems.
Vinoth Kumar, M. (2023). HybDeepNet: A Hybrid Deep Learning Model for Detecting Cardiac Arrhythmia from ECG Signals. Information Technology and Control. doi:10.5755/j01.itc.52.2.32993
Zhang, J., Zhang, C., Lu, J., & Zhao, Y. (2025). Domain-specific Large Language Models for Fault Diagnosis of Heating, Ventilation, and Air Conditioning Systems by Labeled-data-supervised Fine-tuning. Applied Energy, 377, 124378.
Zhang, L., Lin, J., Liu, B., Zhang, Z., Yan, X., & Wei, M. (2019). A review on deep learning applications in prognostics and health management. IEEE Access, 7, 162415–162438.
Zheng, S., Pan, K., Liu, J., & Chen, Y. (2024). Empirical Study on Fine-tuning Pre-trained Large Language Models for Fault Diagnosis of Complex Systems. Reliability Engineering & System Safety, 252, 110382.