Offre de thèse
ENACT APPROCHES D'APPRENTISSAGE FÉDÉRÉ BASÉES SUR LES GRANDS MODÈLES DE LANGAGE (LLMs) POUR UNE MAINTENANCE PRÉDICTIVE INTELLIGENTE ET INTÉGRÉE. DÉPLOIEMENT DANS DES ENVIRONNEMENTS INDUSTRIELS TEMPS RÉELS, EMBARQUÉS ET FRUGAUX.
Date limite de candidature
21-04-2025
Date de début de contrat
01-10-2025
Directeur de thèse
PONSART Jean-Christophe
Encadrement
Prof. KNITTEL Dominique - LEM3 (Université de Lorraine)
Type de contrat
école doctorale
équipe
CID - Contrôle - Identification - Diagnosticcontexte
This PhD offer is provided by the ENACT AI Cluster and its partners. Find all ENACT PhD offers and actions on https://cluster-ia-enact.ai/. The laboratory CRAN was created in 1980, CRAN is a “Joint Research Unit ‐ UMR 7039” shared between the University of Lorraine (Scientific Pole “Automation, Mathematics, Computer Science, and their Interactions ‐ AM2I”) and CNRS (Institute “CNRS Computer Science”). The laboratory brings together 250 members including 120 researchers (8 CNRS researchers ‐ section 7 of CoNRS), researchers from the Lorraine Cancer Institute (ICL), the Regional Hospital Centers (CHRU Nancy and CHR Thionville), or several other external organizations. The administrative service and research support services bring together 30 staff members. CRAN hosts nearly hundred doctoral and post-doctoral students. The research works are based on more than 20 prototypes, demonstrators, platforms or software. Some of them are labelled INFRA+. CRAN benefits from industrial collaborations with major companies such as Airbus, ArcelorMittal, CNES, EDF, Dassault Aviation, POST Luxembourg, Renault, SAFRAN, SAFT SAS, Schneider, SPIE. CRAN has strong collaborations with foreign universities or research centers. The research conducted at CRAN covers the fields of automation, signal and image processing, computer engineering, manufacturing, biology, and neurology in connection with cancer research and neurosciences. The laboratory is organized into three scientific departments: - Control Identification Diagnosis Department (CID): automatic control, dynamical systems; - Modeling, Control, and Safety of Industrial Systems Department (MPSI): automation, manufacturing, computer engineering; - Biology, Signals, and Systems in Oncology and Neurosciences Department (BioSiS): biology related to cancer and neurology, signal and image processing. The laboratory LEM3 (Laboratory for the Study of Microstructures and Mechanics of Materials) is an academic research laboratory whose missions are threefold: to manage research projects in mechanics and materials, to promote the results of this research and to contribute to university education and continuing education through and for research. The laboratory LEM3 is a joined research unit (UMR) attached to the University of Lorraine, CNRS and Arts et Métiers. It is part of M4 scientific Pole (Matter, Materials, Metallurgy, Mechanics) of University of Lorraine. LEM3 brings together around 250 people including 150 permanent staff and more than 80 doctoral and post-doctoral students. The main LEM3 building is located in Metz, on the Technopôle university campus. Other research teams are based in Nancy and in Saint-Dié-des-Vosges . LEM3 is a major force in research in the mechanics of materials and manufacturing processes. Its experimental and theoretical activities are transdisciplinary, combining solid mechanics, metallurgy, materials science, chemistry and physics. It is organized within three scientific departments: (1) Mechanics of materials, structures and life (MMSV), (2) Engineering of microstructures, processes, anisotropy, behaviour (IPACT), (3) Thermomechanics of processes and tool-material interactions (T-PRIOM). By maintaining the balance between fundamental and applied approaches, the laboratory ensures high visibility of its cutting-edge research and effective transfer of academic knowledge to industrial partners. Idemoov is a French startup specializing in IoT (Internet of Things) engineering and AI-driven solutions. Founded in 2020 and based in Alsace, Idemoov has a multidisciplinary team of 22 experts in cybersecurity, DevOps, embedded technologies, data science, and artificial intelligence. The company designs and develops custom IoT solutions, integrating hardware and software expertise across multiple domains. Idemoov is actively engaged in industrial predictive maintenance through its IdeIndus project, which focuses on developing AI-powered predictive maintenance systems for industrial applications. The company has a full-stack approach, handling the entire data processing pipeline—from sensor deployment and data acquisition to cloud-based analytics and real-time monitoring. Given that industrial malfunctions account for approximately €22 billion in damages annually, Idemoov aims to mitigate these losses through advanced predictive maintenance solutions. Studies suggest that nearly 40% of these failures could be prevented with timely interventions, highlighting the critical need for AI-driven condition monitoring and fault detection. As part of France 2030, Idemoov has received financial support from the French government and the Grand Est region under the PIA4 (Programme d'Investissements d'Avenir) initiative. The academic research team associated to this thesis is composed of four people. Two are members of CRAN laboratory: Prof. J-C. Ponsart and Dr. M.S. Jha and the two others are members of LEM3 laboratory: Prof. D. Knittel and Prof. M. Nouari. The research topics associated to each member are: - Prof. J-C. Ponsart: Diagnostic and Fault-Tolerant Control, Health Aware Control, Multi-Agent Systems, ground and aerial vehicles applications; - Dr. M.S. Jha: Reinforcement Learning, Health Aware Control, Diagnosis and Prognosis - Prof. D. Knittel: Mechatronics and robust control, AI for manufacturing systems; - Prof. M. Nouari: Numerical simulation and experimental analysis of manufacturing processes. Our industry partner Idemoov (an engineering company located at Entzheim (67)) is also involved in this thesis project. Idemoov will test in industrial manufacturing environment the obtained results along the different research phases and give the feedback for helping improvements.spécialité
Automatique, Traitement du signal et des images, Génie informatiquelaboratoire
CRAN - Centre de Recherche en Automatique de Nancy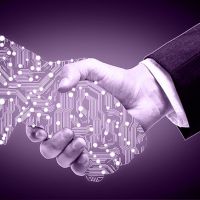
Mots clés
Maintenance prédictive intelligente et intégrée, Pronostics et gestion de l'état de santé (PHM), Diagnostic et pronostic intégrés, Grands modèles de langage (LLMs), Apprentissage fédéré, Environnement embarqué frugal
Détail de l'offre
Cette recherche propose une approche innovante pour la maintenance prédictive en milieu industriel, combinant les grands modèles de langage (LLM), l'apprentissage fédéré et l'IA frugale. L'objectif est de concevoir des modèles intelligents de diagnostic et de pronostic capables de fonctionner en temps réel sur du matériel embarqué à ressources limitées. Cette approche répond aux défis liés à la complexité des systèmes de fabrication modernes, où des données multimodales—issues de capteurs, jumeaux numériques et dispositifs IIoT—doivent être fusionnées pour surveiller l'état des machines, détecter les anomalies et prédire la durée de vie restante (RUL).
Le cadre proposé intègre divers flux de données pour générer un indice de santé dynamique. Cet indice alimente les algorithmes de diagnostic et de détection d'anomalies, permettant une identification rapide des pannes potentielles. Les modèles prédictifs anticipent la dégradation des composants, facilitant ainsi une planification optimale des interventions de maintenance tout en tenant compte des contraintes de production. Cette approche vise à équilibrer la planification de la maintenance, la continuité de la production et l'optimisation des coûts.
Les avancées récentes en Pronostic et Gestion de la Santé (PHM) exploitent l'apprentissage profond, la fusion de données multimodales et les architectures à base de transformeurs. Popularisés par Vaswani et al. (2017) et perfectionnés par Devlin et al. (2018), ces modèles ont ouvert de nouvelles perspectives pour la détection des pannes et le pronostic (Li et al. 2021 ; Chen et al. 2021 ; Alsaif et al. 2024 ; Zhang et al. 2025 ; Zheng et al. 2024). Par ailleurs, des approches intégrant des agents LLM et des autoencodeurs ont émergé pour soutenir les systèmes PHM.
S'appuyant sur cet état de l'art, cette thèse poursuit quatre objectifs. Premièrement, développer des autoencodeurs basés sur les LLM capables de fusionner des données hétérogènes (capteurs, vidéos, journaux textuels) afin de construire une représentation compacte de l'état des machines. Deuxièmement, améliorer le pronostic en intégrant la détection des anomalies avec des modèles séquentiels capturant les dynamiques temporelles et l'incertitude. Troisièmement, optimiser la planification de la maintenance à l'aide d'algorithmes avancés d'aide à la décision, garantissant un équilibre optimal entre production et maintenance. Enfin, concevoir un cadre d'apprentissage fédéré pour un entraînement décentralisé des modèles, assurant sécurité, évolutivité et respect de la confidentialité sur plusieurs sites industriels. Une attention particulière sera portée à l'IA frugale (élagage de modèles, quantification, distillation des connaissances) afin de garantir une mise en œuvre efficace sur matériel embarqué.
Les résultats attendus incluent une meilleure précision du diagnostic, une amélioration des prédictions de la RUL et une planification optimisée des interventions, réduisant ainsi les temps d'arrêt et les coûts opérationnels tout en améliorant l'efficacité des équipements. Cette recherche vise à fournir un cadre évolutif et sécurisé pour doter les systèmes industriels de capacités de maintenance prédictive en temps réel, améliorant ainsi la performance dans des environnements à ressources limitées.
Les approches seront validées sur des centres d'usinage du laboratoire LEM3 et testées en environnement industriel avec l'entreprise Idemoov.
Keywords
Intelligent and Integrated Predictive Maintenance, Prognostics and Health Management (PHM), Integrated Diagnosis and Prognosis, Large Language Models (LLMs), Federated Learning, Frugal Embedded Environment
Subject details
This research proposes a novel approach for predictive maintenance in industrial environments by leveraging large language model (LLM) based techniques integrated with federated learning and frugal AI deployment. The objective is to develop intelligent, integrated diagnostic and prognostic models that can operate in real-time on embedded, resource-constrained hardware. This approach addresses the challenges posed by the complexity and heterogeneity of modern manufacturing systems, where multimodal data—collected from onboard sensors, cyber-physical systems, digital twins, and IIoT devices—must be fused and processed to monitor machinery health, detect anomalies, and predict degradation behaviors such as remaining useful life (RUL). The proposed framework focuses on extracting and integrating diverse data streams to generate a dynamic health index. This index drives both diagnostic algorithms and anomaly detection methods, enabling the timely identification of potential failures. At the same time, predictive models built upon this health index forecast component degradation, facilitating the scheduling of maintenance interventions that harmonize with production constraints. By balancing objectives such as maintenance scheduling, production continuity, transportation logistics, and personnel availability, the research aims to optimize overall system performance and cost efficiency. Recent advances in Prognostics and Health Management (PHM) have shifted from traditional condition-based maintenance to sophisticated approaches that harness deep learning, multimodal data fusion, and transformer-based architectures. The emergence of transformer-based models, popularized by Vaswani et al. (2017) and refined in subsequent works (Devlin et al., 2018), has opened new avenues for fusing and processing sequential and multimodal data. Recent contributions have further extended these models to fault detection and prognostics (Li et al. 2021; Chen et al. 2021; Alsaif et al. 2024; Zhang et al. 2025; Zheng et al. 2024), while innovative paradigms employing LLM agents and autoencoder architectures have emerged to support integrated PHM systems. Building upon this state of the art, the thesis is organized around four primary objectives. First, it will develop LLM-based autoencoders capable of fusing heterogeneous data—such as sensor readings, video, and textual logs—to construct a compact latent representation of machinery health. Second, the diagnostic framework will be extended to prognostics, focusing on RUL prediction by integrating anomaly detection with sequential modeling techniques that capture temporal dynamics and uncertainty. Third, the research will utilize the combined diagnostic and prognostic outputs to optimize maintenance scheduling through advanced decision-support algorithms, ensuring an optimal balance between production and maintenance activities. Fourth, a federated learning framework will be established for decentralized model training and updating, enabling secure, scalable, and privacy-preserving operations across distributed industrial sites. Special emphasis will be placed on frugal AI techniques, including model pruning, quantization, and knowledge distillation, to ensure that the resulting models are lightweight enough for deployment on embedded hardware. Anticipated outcomes include enhanced diagnostic accuracy, improved RUL predictions, and optimized maintenance planning that together reduce downtime, lower operational costs, and increase equipment effectiveness. Ultimately, the proposed research aims to deliver a comprehensive, scalable, and secure framework that empowers modern industrial systems with real-time, context-aware predictive maintenance capabilities, thus transforming operational efficiency in resource-constrained environments. All the approaches will be validated on industrial machining centers at the LEM3 laboratory and also tested in industrial manufacturing environment with the company Idemoov.
Profil du candidat
Les candidats titulaires d'un master dans l'un des domaines suivants sont éligibles :
- Intelligence artificielle/science des données,
- Sciences informatiques,
- Traitement du signal et/ou Traitement du langage naturel,
- Ingénierie (mécatronique, systèmes complexes, génie électrique, systèmes embarqués, pronostic et gestion de la santé, etc.)
La connaissance de l'IA est essentielle pour tous les candidats.
Candidate profile
Applicants with a master's degree in one of the following fields are eligible:
- Artificial Intelligence/data Science,
- Computer Sciences,
- Signal Processing and/or Natural Language Processing,
- Engineering Systems (Mechatronics, Complex systems, Electrical engineering, Embedded systems, Prognostics and Health management, …)
AI Knowledge is essential for all candidates.
Référence biblio
Adekunle, A. A., Fofana, I., Picher, P., Rodriguez-Celis, E. M., & Arroyo-Fernandez, O. H. (2024). Analyzing transformer insulation paper prognostics and health management: A modeling framework perspective. IEEE Access.
Aizpurua, J. I., Stewart, B. G., McArthur, S. D., Penalba, M., Barrenetxea, M., Muxika, E., & Ringwood, J. V. (2022). Probabilistic forecasting informed failure prognostics framework for improved RUL prediction under uncertainty: A transformer case study. Reliability engineering & system safety, 226, 108676.
Alsaif, K. M., Albeshri, A. A., Khemakhem, M. A., & Eassa, F. E. (2024). Multimodal Large Language Model-Based Fault Detection and Diagnosis in Context of Industry 4.0. Electronics, 13(24), 4912.
Bainier, G., Marx, B. & Ponsart, J-C. (2025). Thresholding in Set-Membership Approaches to Fault Isolation using the Minkowski functional. International Journal of Robust and Nonlinear Control, to appear in 2025.
Chen, T., Zhang, J., & Wang, H. (2021). Remaining Useful Life Prediction of Aero-Engines Using a Transformer-Based Network. IEEE Access, 9, 12345–12355.
Cui, J., Kuang, W., Geng, K., & Jiao, P. (2025). Intelligent fault diagnosis and operation condition monitoring of transformer based on multi-source data fusion and mining. Scientific Reports, 15(1), 7606.
Devlin, J., Chang, M.-W., Lee, K., & Toutanova, K. (2018). BERT: Pre-training of Deep Bidirectional Transformers for Language Understanding.
El Hassani, I., Masrour, T., Kourouma, N., Motte, D., & Tavčar, J. (2024). Integrating Large Language Models for Improved Failure Mode and Effects Analysis (FMEA): A Framework and Case Study. Proceedings of the Design Society, 4, 2019-2028.
Fink, O., Wang, Q., Svensen, M., Dersin, P., Lee, W. J., & Ducoffe, M. (2020). Potential, challenges and future directions for deep learning in prognostics and health management applications. Engineering Applications of Artificial Intelligence, 92, 103678.
Garcia, R., et al. (2024). Federated learning-based health monitoring in industrial cyber-physical systems. Computers in Industry, 140, 103565.
Guo, Z., et al. (2024). Efficient edge computing with federated learning for predictive maintenance in industrial IoT. IEEE Internet of Things Journal, 11(4), 2350-2361.
Han, S., Pool, J., Tran, J., & Dally, W. (2015). Learning both Weights and Connections for Efficient Neural Network. Advances in Neural Information Processing Systems (NeurIPS).
Hervé de Beaulieu, M., Jha, M.S., Garnier, H. & Cerbah, F. (2024). Remaining Useful Life prediction based on physics-informed data augmentation. Reliability Engineering & System Safety, 252, 110451.
Hu, Y., Miao, X., Si, Y., Pan, E., & Zio, E. (2022). Prognostics and health management: A review from the perspectives of design, development and decision. Reliability Engineering & System Safety, 217, 108063.
Jardine, A. K., Lin, D., & Banjevic, D. (2006). A review on machinery diagnostics and prognostics implementing condition-based maintenance. Mechanical systems and signal processing, 20(7), 1483-1510.
Jha, Mayank Shekhar, et al. 'Particle Filter based hybrid prognostics of proton exchange membrane fuel cell in bond graph framework.' Computers & Chemical Engineering 95 (2016b): 216-230.
Jha, Mayank Shekhar, Geneviève Dauphin-Tanguy, and B. Ould-Bouamama. “Particle filter based hybrid prognostics for health monitoring of uncertain systems in bond graph framework.” Mechanical Systems and Signal Processing 75 (2016a): 301-329.
Kanso, S., Jha, M.S. & Theilliol, D. (2024). Degradation Tolerant Optimal Control Design for Stochastic Linear Systems. International Journal of Applied Mathematics and Computer Science (AMCS).
Knittel, D., Makich, H., Messer, I. & Nouari, M. (2025). Vibration Based Honeycomb Core Milling Diagnostics Using Machine Learning Approaches. To appear in Procedia CIRP Journal, Elsevier, accepted 1st February 2025.
Knittel, D., Makich, H. & Nouari, M. (2020). Milling diagnosis using artificial intelligence approaches. Mechanics and Industry 20(8):809.
Lee, S., Park, J., Kim, D., et al. (2023). Secure and scalable federated learning for industrial applications. IEEE Transactions on Industrial Informatics, 19(2), 1234-1242.
Li, W., Zhang, X., & Li, Y. (2021). Transformer-Based Neural Network for Prognostics of Complex Systems. IEEE Transactions on Industrial Informatics, 17(5), 3507–3517.
Li, Y. F., Wang, H., & Sun, M. (2024). ChatGPT-like large-scale foundation models for prognostics and health management: A survey and roadmaps. Reliability Engineering & System Safety, 243, 109850.
López-Estrada, F.R., Theilliol, D., Astorga-Zaragoza, C.M., Ponsart, J.C., Valencia-Palomo, G. & Camas-Anzueto, J. (2019). Fault diagnosis observer for descriptor Takagi-Sugeno systems. Neurocomputing, 331, 10-17.
Lukens, S., McCabe, L. H., Gen, J., & Ali, A. (2024, November). Large Language Model Agents as Prognostics and Health Management Copilots. Annual Conference of the PHM Society, Vol. 16, No. 1.
Lv, J., Yu, Z., Zhang, H., Sun, G., Muhl, P., & Liu, J. (2023). Transformer based long-term prognostics for dynamic operating PEM fuel cells. IEEE Transactions on Transportation Electrification, 10(1), 1747-1757.
McMahan, B., et al. (2017). Communication-Efficient Learning of Deep Networks from Decentralized Data. Proceedings of the 20th International Conference on Artificial Intelligence and Statistics (AISTATS).
Park, M., et al. (2022). Knowledge distillation for resource-constrained deep learning: Applications in industrial prognostics. Neural Computing and Applications, 34(10), 8725-8736.
Ringler, N., Knittel, D., Nouari, M., Ponsart, J.-C., Yakob, A., & Romani, D. (2025). Unsupervised Anomaly Detection using Vibration Signals for Milling Processes. To appear in Procedia CIRP Journal, Elsevier, accepted 1st February 2025.
Rezaeianjouybari, B., & Shang, Y. (2020). Deep learning for prognostics and health management: State of the art, challenges, and opportunities. Measurement, 163, 107929.
Schacht Rodríguez, R., Ponsart, J-C., Garcia-Beltran, C.D., Astorga Zaragoza, C.M. et al. (2018). Path planning generation algorithm for a class of UAV multirotor based on state of health of lithium polymer battery. Journal of Intelligent and Robotic Systems, Springer Verlag, 91(1), 115-131.
Suh, S., Mittal, D.A., Bello, H., Zhou, B., Jha, M.S. & Lukowicz, P. (2024). Remaining useful life prediction of Lithium-ion batteries using spatio-temporal multimodal attention networks. Heliyon, 10(16).
Sun, S., Peng, T., & Huang, H. (2023). Machinery Prognostics and High-Dimensional Data Feature Extraction Based on a Transformer Self-Attention Transfer Network. Sensors, 23(22), 9190.
Susto, G. A., Schirru, A., Pampuri, S., McLoone, S., & Beghi, A. (2015). Machine learning for predictive maintenance: A multiple classifier approach. IEEE Systems Journal, 9(3), 849–857.
Thuillier, J., Jha, M.S., Le Martelot, S. & Theilliol, D. (2024). Prognostics Aware Control Design for Extended Remaining Useful Life: Application to Liquid Propellant Reusable Rocket Engine. International Journal of Prognostics and Health Management, 15(1).
Tsai, Y.-H. H., Liang, P. P., Zadeh, A., & Morency, L.-P. (2019). Multimodal Transformer for Unaligned Multimodal Language Sequences. Proceedings of the 57th Annual Meeting of the Association for Computational Linguistics.
Vaswani, A., Shazeer, N., Parmar, N., et al. (2017). Attention is All You Need. Advances in Neural Information Processing Systems.
Wahid, A., Yahya, M., Breslin, J. G., & Intizar, M. A. (2023). Self-attention transformer-based architecture for remaining useful life estimation of complex machines. Procedia Computer Science, 217, 456-464.
Wang, X., Li, Y., & Zhou, D. (2022). Federated learning for industrial predictive maintenance: A review. IEEE Internet of Things Journal, 9(3), 1500-1512.
Zarrouk, T. & Nouari, M. (2024). Three-Dimensional Finite Element Modeling of Ultrasonic Vibration-Assisted Milling of the Nomex Honeycomb Structure, Algorithms 17(5):204.
Zarrouk, T., Nouari, M. & Benbouaza, A. (2023). Influence of cutting tool geometry when milling Nomex honeycomb structure. International Journal of Advanced Manufacturing Technology, 130, 649–663.
Zarrouk, T., Nouari, M. & Bouali, H. (2025). Numerical Modeling and Optimization of Nomex Honeycomb Core Milling: Influence of Longitudinal and Longitudinal-Torsional Ultrasonic Vibrations. Machines 13(2):99.
Zhang, J., Zhang, C., Lu, J., & Zhao, Y. (2025). Domain-specific Large Language Models for Fault Diagnosis of Heating, Ventilation, and Air Conditioning Systems by Labeled-data-supervised Fine-tuning. Applied Energy, 377, 124378.
Zhang, L., Lin, J., Liu, B., Zhang, Z., Yan, X., & Wei, M. (2019). A review on deep learning applications in prognostics and health management. Ieee Access, 7, 162415-162438.
Zhang, Y., Chen, L., et al. (2023). Frugal AI for industrial embedded systems: Model compression and efficient inference. IEEE Access, 11, 23456-23467.
Zheng, S., Pan, K., Liu, J., & Chen, Y. (2024). Empirical Study on Fine-tuning Pre-trained Large Language Models for Fault Diagnosis of Complex Systems. Reliability Engineering & System Safety, 252, 110382.
Zollanvari, A., Kunanbayev, K., Bitaghsir, S. A., & Bagheri, M. (2020). Transformer fault prognosis using deep recurrent neural network over vibration signals. IEEE Transactions on Instrumentation and Measurement, 70, 1-11.