Offre de thèse
ENACT Architectures matérielles hiérarchiques, flexibles et adaptables pour les réseaux de neurones compétitifs dynamiques et évolutifs appliqués à l'apprentissage continu
Date limite de candidature
21-04-2025
Date de début de contrat
01-10-2025
Directeur de thèse
JOVANOVIC Slavisa
Encadrement
This PhD is a joint project between the Jean Lamour Institute and the LORIA laboratory. It will be supervised by Slavisa Jovanovic (IJL) and Bernard Girau (LORIA). Joint meetings at a regular (at least bi-monthly) basis between two labs will be organized.
Type de contrat
école doctorale
équipe
DEPARTEMENT 4 - N2EV : 406 - Mesures et architectures électroniquescontexte
This PhD is a joint project between the Jean Lamour Institute and the LORIA laboratory. It will be supervised by Slavisa Jovanovic (IJL) and Bernard Girau (LORIA). Slavisa Jovanovic is the member of the MAE team (team 406 of the IJL) of the Jean Lamour Institute. The Institute Jean Lamour (IJL - https://ijl.univ-lorraine.fr/ ) is a joint research unit of CNRS and Université de Lorraine. Focused on materials, processes science, engineering and electronics, it covers: materials, metallurgy, plasmas, surfaces, nanomaterials and electronics. It regroups 183 researchers/lecturers, 91 engineers/technicians/administrative staff, 150 PhD students and 25 post-doctoral fellows. Partnerships exist with 150 companies and our research groups collaborate with more than 30 countries throughout the world. Its exceptional instrumental platforms are spread over 4 sites; the main one is located on Artem campus in Nancy. Slavisa Jovanovic (Associate Professor UL) is the member of the MAE team. One part of the research activities of the MAE team is related to the hardware implementations (FPGA-based) of neural and neuromorphic circuits and systems. Different neural networks models are considered among others SOM-based algorithms, CNN-based (1D, 2D), Transformers, ... The research focus of the team is also on unconventional computing paradigms using traditional CMOS and emergent technologies. Bernard Girau (Professor UL) is head of the Biscuit team of the LORIA laboratory. LORIA is the French acronym for the “Lorraine Research Laboratory in Computer Science and its Applications” and is a research unit (UMR 7503), common to CNRS, the University of Lorraine, CentraleSupélec and Inria. LORIA's missions mainly deal with fundamental and applied research in computer sciences. With 500 people working in the lab, LORIA is today one of the biggest research labs in the Grand Est Region. The long-term goal of the Biscuit team is to propose bio-inspired computing architectures that will learn to control persistent autonomous systems interacting with dynamical, complex and unforeseeable environments. These architectures are mostly inspired by neural mechanisms of self-organization and computation emergence from neural populations driven by sensorimotor feedback. The adaptation of these architectures for hardware implementations (on FPGAs or neuromorphic chips) is also a major topic of the team.spécialité
Systèmes électroniqueslaboratoire
IJL - INSTITUT JEAN LAMOUR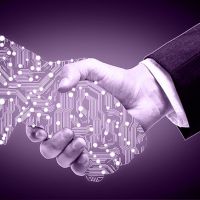
Mots clés
Apprentissage continu, Apprentissage basé sur prototype, Réseaux de neurones dynamiques et évolutifs , Quantification vectorielle, Apprentissage incrémental, Architecture matérielle
Détail de l'offre
Les modèles d'apprentissage automatique (ML) conventionnels (i.e. CNN) sont entraînés sur des distributions de données statiques [1]. En effet, ils sont optimisés pour les données vues pendant les phases d'entraînement. Si les données changent, en introduisant de nouvelles classes ou en modifiant le contexte, une dégradation des performances des modèles ML conventionnels est observée.
Dans les problèmes du monde réel, la distribution des données est souvent dynamique, et les modèles ML et réseaux neuronaux (NN) utilisés doivent faire face à cette évolution continue. De plus, ces modèles sont continuellement exposés au dilemme stabilité-plasticité [1], où un compromis entre la mémoire disponible utilisée pour stocker (et conserver) les anciennes connaissances et la possibilité d'apprendre de nouvelles connaissances (sans sacrifier les anciennes) est constamment recherché. Par conséquent, les problèmes du monde réel devraient être abordés avec des modèles ML et NN capables d'apprendre en continu sans interruption [3] et restant dans une boucle d'apprentissage continu et ouvert [4].
Il existe de nombreux domaines d'application où des scénarios d'apprentissage continu (CL) peuvent être trouvés : traitement du langage naturel (NLP) [6] ; robotique [7-9] ; détection de dérive pour les systèmes IoT utilisés dans les Smart Grids [10] ; analyse dynamique des données [11] ; cartographie topologique en ligne [12], etc. Toutes ces approches CL courantes sont basées sur des hypothèses concernant la disponibilité de données étiquetées [13]. En pratique, les données ne sont pas toujours disponibles, elles sont non étiquetées, souvent inconnues, et les modèles doivent être capables d'intégrer des nouveautés aux connaissances déjà acquises [13].
Dans ce projet, l'accent est mis sur l'apprentissage basé sur des prototypes (PB) et les modèles non supervisés pour relever les défis liés à l'apprentissage continu. Différentes approches non supervisées pour l'apprentissage continu existent [14-16]. Parmi les modèles possibles, nous nous intéressons à ceux basés sur les méthodes PB (cartes auto-organisatrices, réseaux incrémentaux).
Des études récentes ont montré que les méthodes PB sont des candidates pour relever les défis de l'apprentissage continu [21, 22]. Une multitude de modèles PB différents (LVQ, GG, GCS, GNG, RW, SOM, etc.) permet de résoudre les problèmes liés à l'apprentissage continu. De plus, du côté de la mise en œuvre, de nombreux travaux de recherche ont également été publiés [23]. Dans le contexte de l'apprentissage continu, les modèles dynamiques et croissants sont préférés en raison de leur propriété intrinsèque d'adapter leur topologie aux nouveaux flux de données, mais ils sont très complexes pour les implémentations matérielles (HW).
La thèse proposée est liée à la conformité des algorithmes PB avec les contraintes matérielles (HW) et les choix de conception spécifiques à HW, permettant ainsi de proposer et déployer des modèles neuronaux dynamiques, adaptatifs, flexibles et hiérarchiques en HW. Les deux objectifs principaux sont : étudier, analyser et identifier les besoins architecturaux HW pour ces modèles neuronaux hiérarchiques, dynamiques et croissants (spécifications HW), et adapter nos modèles et algorithmes aux contraintes HW telles que la précision limitée, la bande passante, la mémoire, la consommation. Avec ces spécifications HW et algorithmes conformes aux HW clairement identifiés, différentes architectures seront proposées, permettant de construire et structurer hiérarchiquement différents modèles et graphes neuronaux croissants et dynamiques, et ainsi de relever la nature toujours changeante des données.
Le doctorat proposé bénéficiera des résultats et des modèles actuellement en développement dans le projet ANR SORLAHNA, où les modèles et architectures matérielles de certains modèles neuronaux croissants sont en développement [25-30].
Keywords
Continual Learning, Prototype-based Learning, Growing and dynamic NN models, Vector Quantization, Incremental and Lifelong learning, Hardware architecture
Subject details
Conventional machine learning (ML) models (i.e. CNNs) are trained on static data distributions [1]. Indeed, they are optimized for the data seen during the training phases and their generalization is successful if data classes were presented to the model during the training. If data changes, by introducing new classes or by changing the context, performance degradation of the conventional ML models is observed. Thus, these models are unsuitable to cope with real-world dynamics. In real-world problems, the data distribution is often dynamic and the used ML and neural network (NN) models must cope with this continuous evolution. Moreover, these models are continuously exposed to the stability-plasticity dilemma [1], where a trade-off between the available memory used to store (and keep) the old knowledge and the possibility to learn new knowledge (not at the detriment of the old one) is always looked for. Consequently, the real-world problems should be tackled with ML and NN models capable of learning continuously without stopping [3] and being in open-ended continual learning loop [4]. There are many application fields where CL scenarios can be found: natural language processing (NLP) [6]; robotics [7-9]; drift detection for IoT-enabled Smart Grid Systems [10]; dynamic data analysis [11]; online topological mapping [12], etc. All these common CL approaches are based on the assumptions on the availability of labeled data [13]. In practice, the data are not always available, they are unlabelled, often unknown and the models must be capable of integrating novelties to the current body of knowledge [13]. Consequently, unsupervised learning is in good position to tackle the mentioned issues. In this project, the main focus is on the prototype-based (PB) learning and the unsupervised models to tackle the challenges related to CL. Different unsupervised approaches for CL can be found [14-16]. Among the possible models, we are interested in models based on PB methods (self-organizing maps, incremental networks). The PB methods under investigation are not only limited for the CL context, but are used in many other approaches, such as the non-uniform quantization of Large-Language-Models (LLM) [17-20]. Recent studies have shown that PB methods are one of the candidates to tackle the challenges of CL [21, 22]. A myriad of different PB models (LVQ, GG, GCS, GNG, RW, SOM, etc) allow to tackle the problems related to CL. Moreover, on the implementation side many research works have also been published [23]. In the context of CL, the dynamic and growing models are preferred due to their inherent property to adapt their topology to new data streams, but are very challenging for hardware (HW) implementations. The proposed PhD is related to the compliance of PB algorithms with HW constraints and HW specific design choices to take allowing to propose and deploy flexible, adaptable, dynamic and hierarchical growing neural models and graphs in HW. The two main objectives are : to investigate and identify HW architectural needs for these hierarchical dynamic and growing neural models (HW specifications), and to adapt our models and algorithms to HW constraints such as limited precision, bandwidth, memory, consumption. With these HW specifications and HW compliant algorithms clearly identified, different architectures will be proposed, allowing to hierarchically build and structure different growing and dynamic neural models and graphs, and thus to tackle the ever-changing nature of data. Moreover, the flexibility of proposed architectures will allow to modify at run time the expected Quality-of-Results (QoR) for a given application by choosing a trade-off between performances, accuracy, consumption or used resources. The proposed PhD will benefit from the results and the models currently under development in the ANR project SORLAHNA, where the HW models and architecture of some growing neural models are under development [25-30].
Profil du candidat
Le candidat à la thèse doit avoir les compétences suivantes:
• une bonne maitrise de l'électronique numérique et de la conception matérielle (HW) (VHDL/Verilog, SystemC, HLS) - FPGA et/ou ASIC,
• un bon niveau en programmation (C/C++, Python),
• un bon niveau d'anglais (oral et écrit) est obligatoire, un niveau élémentaire en français est souhaitable,
• un très grand intérêt et motivation pour la recherche et développement.
Candidate profile
The future PhD candidate should have the following skills:
• strong knowledge of hardware (HW) digital design (VHDL/Verilog, SystemC, HLS) - FPGA and/or ASIC,
• good level of programming skills are expected (C/C++, Python),
• good knowledge of English (oral and written) is mandatory, basic knowledge of French would be an
advantage,
• high motivation for research and development.
Référence biblio
[1] L. Wang, X. Zhang, H. Su, and J. Zhu, “A comprehensive survey of continual learning: Theory, method and application,” IEEE Transactions on Pattern Analysis and Machine Intelligence, 2024.
[2] F. Zenke, B. Poole, and S. Ganguli, “Continual learning through synaptic intelligence,” in International conference on machine learning, 2017, pp. 3987–3995.
[3] G. A. Tahir and C. K. Loo, “An Open-Ended Continual Learning for Food Recognition Using Class Incremental Extreme Learning Machines,” IEEE Access, vol. 8, pp. 82328–82346, 2020.
[4] G. M. Van De Ven, T. Tuytelaars, and A. S. Tolias, “Three types of incremental learning,” Nature Machine Intelligence, vol. 4, no. 12, pp. 1185–1197, Dec. 2022.
[5] G. M. van de Ven and A. S. Tolias, “Three scenarios for continual learning.” 15-Apr-2019.
[6] K. Ahrens, F. Abawi, and S. Wermter, “DRILL: Dynamic representations for imbalanced lifelong learning,” in Artificial neural networks and machine learning–ICANN 2021: 30th international conference on artificial neural networks, bratislava, slovakia, september 14–17, 2021, proceedings, part II 30, 2021, pp. 409–420.
[7] N. Duczek, M. Kerzel, P. Allgeuer, and S. Wermter, “Self-organized Learning from Synthetic and Real-World Data for a Humanoid Exercise Robot,” Frontiers in Robotics and AI, vol. 9, p. 669719, Oct. 2022.
[8] M. Iwasa, N. Kubota, and Y. Toda, “Multi-scale batch-learning growing neural gas for topological feature extraction in navigation of mobility support robots,” in The 7th international workshop on advanced computational intelligence and intelligent informatics (IWACIII 2021), 2021.
[9] M. Jayaratne, D. Alahakoon, and D. De Silva, “Unsupervised skill transfer learning for autonomous robots using distributed Growing Self Organizing Maps,” Robotics and Autonomous Systems, vol. 144, p. 103835, Oct. 2021.
[10] Z. Jiang, R. Lin, and F. Yang, “An Incremental Clustering Algorithm with Pattern Drift Detection for IoT-Enabled Smart Grid System,” Sensors, vol. 21, no. 19, p. 6466, Sep. 2021.
[11] R. Dwivedi et al., “An incremental clustering method based on multiple objectives for dynamic data analysis,” Multimedia Tools and Applications, vol. 83, no. 13, pp. 38145–38165, Oct. 2023.
[12] A. Junaedy et al., “Online Topological Mapping on a Quadcopter with Fast Growing Neural Gas,” Journal of Advanced Computational Intelligence and Intelligent Informatics, vol. 28, no. 6, pp. 1354–1366, Nov. 2024.
[13] N. Hajinazar et al., “SIMDRAM: A Framework for Bit-Serial SIMD Processing Using DRAM,” arXiv:2012.11890 [cs], Dec. 2020.
[14] S. Basterrech, L. Clemmensen, and G. Rubino, “A Self-Organizing Clustering System for Unsupervised Distribution Shift Detection,” in 2024 International Joint Conference on Neural Networks (IJCNN), 2024, pp. 1–9.
[15] N. Masuyama, Y. Nojima, F. Dawood, and Z. Liu, “Class-Wise Classifier Design Capable of Continual Learning Using Adaptive Resonance Theory-Based Topological Clustering,” Applied Sciences, vol. 13, no. 21, p. 11980, Nov. 2023.
[16] Z. Li, X. Jiang, R. Missel, P. K. Gyawali, N. Kumar, and L. Wang, “CONTINUAL UNSUPERVISED DISENTANGLING OF SELF-ORGANIZING REPRESENTATIONS,” 2023.
[17] J. Martinez, J. Shewakramani, T. Wei Liu, I. Andrei Barsan, W. Zeng, and R. Urtasun, “Permute, Quantize, and Fine-tune: Efficient Compression of Neural Networks,” in 2021 IEEE/CVF Conference on Computer Vision and Pattern Recognition (CVPR), 2021, pp. 15694–15703.[18] M. Van Baalen et al., “Gptvq: The blessing of dimensionality for llm quantization,” arXiv preprint arXiv:2402.15319, 2024.
[19] Z. Liu et al., “VQ-LLM: High-performance code generation for vector quantization augmented LLM inference,” arXiv preprint arXiv:2503.02236, 2025.
[20] T. F. van der Ouderaa, M. L. Croci, A. Hilmkil, and J. Hensman, “Pyramid vector quantization for llms,” arXiv preprint arXiv:2410.16926, 2024.
[21] Y. Wei, J. Ye, Z. Huang, J. Zhang, and H. Shan, “Online prototype learning for online continual learning,” in 2023
IEEE/CVF international conference on computer vision (ICCV), 2023, pp. 18718–18728.
[22] S. Ho, M. Liu, L. Du, L. Gao, and Y. Xiang, “Prototype-Guided Memory Replay for Continual Learning,” IEEE Transactions on Neural Networks and Learning Systems, pp. 1–11, 2023.
[23] S. Jovanovic and H. Hikawa, “A Survey of Hardware Self-Organizing Maps,” IEEE Transactions on Neural Networks and Learning Systems, vol. 34, no. 11, pp. 8154–8173, Nov. 2023.
[24] E. Verwimp et al., “Continual learning: Applications and the road forward,” arXiv preprint rXiv:2311.11908, 2023.
[25] F. DERUE, S. JOVANOVIC, H. RABAH, and S. WEBER, “Scalable and fully configurable NoC-based hardware implemention of growing neural gas for continual learning,” in 2024 31st IEEE international conference on electronics, circuits and systems (ICECS), 2024, pp. 1–4.
[26] F. DERUE, S. JOVANOVIC, H. RABAH, and S. WEBER, “SWAP-GNG: A Scalable HW Architecture for Multi Growing Neural Gas Graph Learning for Continual Learning,” in 2025 7th IEEE international conference on Artificial Inteligence circuits and systems (AICAS), 2025, pp. 1–4.
[27] B. Girau and C. Torres-Huitzil, “Fault tolerance of self-organizing maps,” Neural Computing and Applications, vol. 32, no. 24, pp. 17977–17993, Dec. 2020.
[28] A. Upegui, B. Girau, N. Rougier, F. Vannel, and B. Miramond, “Pruning Self-Organizing Maps for Cellular Hardware Architectures,” in 2018 NASA/ESA Conference on Adaptive Hardware and Systems (AHS), 2018, pp. 272–279.
[29] L. Khacef, B. Girau, N. Rougier, A. Upegui, and B. Miramond, “Neuromorphic hardware as a self-organizing computing system.” 30-Oct-2018.
[30] B. Girau and C. Torres-Huitzil. Fault tolerance of Self-Organizing Maps. Neural Computing and Applications. Doi : 10.1007/s00521- 018-3769-6, 2018.