Offre de thèse
ENACT Chatbot axé sur le comportement social pour le coaching en communication des étudiants en médecine
Date limite de candidature
01-09-2025
Date de début de contrat
01-10-2025
Directeur de thèse
ILLINA Irina
Encadrement
Co-directeur : Patrice Gallet, PU/PH UL, CHRU Nancy, laboratoire NGERE Co-encadrant : · Fabrice Lefèvre, PR Avignon Université, LIA Collaborateur : Mathieu Kuchenbuch, MCU/PH UL, CHRU Nancy-Hôpitaux de Brabois
Type de contrat
école doctorale
équipe
MULTISPEECHcontexte
Thesis directors : · Irina Illina, MCF UL, HDR, Multispeech Team, Loria · Patrice Gallet, PU/PH UL, CHRU Nancy, NGERE Thesis co-supervisor: · Fabrice Lefèvre, PR Avignon University, LIA Collaborator: Mathieu Kuchenbuch, MCU/PH UL, CHRU Nancy-Hôpitaux de Brabois LORIA is a public computer science research laboratory jointly operated by CNRS, Université de Lorraine, and Inria. LORIA's primary mission encompasses both fundamental and applied research in computer science. The laboratory is structured into 28 research teams, organized across five departments: 1. Algorithms, Computation, Image & Geometry, 2. Formal Methods, 3. Networks, Systems and Services, 4. Natural Language Processing & Knowledge Discovery, 5. Complex Systems, Artificial Intelligence and Robotics. With a community of over 500 members, LORIA stands as one of the largest research laboratories in the Grand Est region of France. The Multispeech team is active in acoustic and language modeling of speech, emphasizing deep learning-based expressive speech synthesis, voice anonymisation, speech recognition and natural language processing. It has coordinated or participated in many projects (e.g., H2020 COMPRISE, ANR SyncoGest, ANR DEEP-PRIVACY, ANR VISAC, ANR-DFG IFCASL, EUREKA Emospeech, Equipex ORTOLANG). NGERE (Nutrition-Genetics and Environmental Risk Exposure) research unit, UMR U1254 UL-INSERM, focuses its research activity on the trajectories of normal and pathological aging, particularly in relation to the one-carbon metabolism, the genetics of obesity, cancers and the chronic inflammatory bowel diseases (IBD). They are developing preclinical studies on innovative treatments for rare diseases, steatohepatitis and chronic inflammatory bowel diseases. LIA (Laboratoire Informatique d'Avignon) is a public research laboratory in computer science affiliated with Avignon Université. It focuses on speech and language technologies. It often participates in international evaluation campaigns, showing its mastery of such technologies by reaching top rankings. It has coordinated or participated in several national and European projects (e.g., ANR VoxCrim, ANR-JST VoicePersonae, ANR DEEP-PRIVACY, ANR muDialBot, H2020 SELMA) and developed close partnerships with industry.spécialité
Informatiquelaboratoire
LORIA - Laboratoire Lorrain de Recherche en Informatique et ses Applications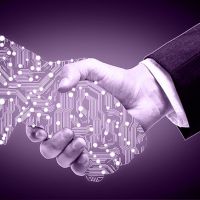
Mots clés
traitement du langage naturel, systèmes de dialogue, grands modèles de langage médicaux, personnalisation des chatbots, grands modèles de langage
Détail de l'offre
Motivations et contexte
Des compétences en communication efficaces sont cruciales dans les professions de santé pour une bonne transmission de l'information et le maintien de la confiance des usagers. Malheureusement, les possibilités de formation à ces compétences sont souvent limitées par le besoin d'interviewés humains, ce qui augmente les coûts et réduit l'accessibilité. Les progrès récents en traitement automatique du langage naturel (TALN) permettent de créer des agents multimodaux virtuels capables de simuler ces entretiens.
Cette thèse de doctorat porte sur la formation des étudiants en médecine aux examens cliniques structurés par objectifs (ECOS), un exercice où ils incarnent un médecin interagissant avec un « patient standardisé » joué par un volontaire en présence d'un évaluateur qui évalue leurs compétences cliniques et communicationnelles. L'ECOS compte pour un tiers des points de l'examen national de classement. La Faculté de médecine de l'UL a été pionnière dans la mise en œuvre de ces ECOS en France et vise à en élargir l'accès grâce aux technologies de l'IA.
Cette thèse abordera les défis de la recherche en IA liés à la création d'un système de coaching en communication pour les ECOS utilisant des agents conversationnels multimodaux. De nouvelles méthodologies seront étudiées pour générer la réponse textuelle du patient virtuel et pour modéliser la personnalité du patient capable de réagir d'une manière qui s'aligne sur le comportement social du médecin observé (verbal et non verbal).
État de l'art
Dans le domaine du TALN, l'intégration de la personnalité dans les chatbots suscite un intérêt croissant. Les chatbots personnalisés visent à offrir des interactions plus engageantes et contextuellement adaptées en incarnant différents traits de personnalité. Le Big Five (« OCEAN », acronyme de ses cinq traits) est un cadre largement utilisé (Mount15). Cependant, les approches existantes basées sur OCEAN ne parviennent souvent pas à représenter correctement la complexité de la personnalité humaine.
Objectifs de la thèse
Objectif 1 : Génération de texte de patient virtuel tenant compte des connaissances. Nous exploiterons les travaux récents du LIA sur la création de modèles neuronaux de haute qualité adaptés aux données médicales (Labrak24, Labrak24b) afin de produire des données spécifiques aux scénarios envisagés lors d'un ECOS. Pour générer la réponse textuelle du patient virtuel, nous étudierons les invites à zéro et à quelques coups, l'apprentissage par transfert, le RAG (Lewis20), etc. afin d'adapter les LLM du domaine ouvert au contexte professionnel (Njifenjou24), en tenant compte des connaissances médicales et du vocabulaire de spécialité.
Objectif 2 : Génération de texte tenant compte du comportement social et de la personnalité. La réponse textuelle du patient virtuel doit avoir une personnalité capable de réagir de manière conforme à la fois à cette personnalité et au comportement social du médecin observé. La nouvelle approche de modélisation de la personnalité pour les chatbots proposée dans (Njifendjou25) sera étendue pour relever un nouveau défi concernant le degré de révélation de soi de l'agent. En effet, l'agent est censé jouer le rôle d'un patient (naïf) tout en possédant une compréhension approfondie des subtilités médicales de sa situation (nécessaire pour simuler les symptômes appropriés). Un contrôle accru de sa personnalité sous-jacente lors de la conversation devrait garantir une meilleure simulation lors de l'ECOS.
À la fin de la thèse, un modèle patient complet sera élaboré et évalué à partir des données d'ECOS précédemment collectées.
Keywords
natural language processing, dialogue systems, medical large language models, personalizing chatbots., large language models
Subject details
Motivations and context Effective communication skills are crucial in healthcare professions for good information delivery and maintaining users' trust. Unfortunately, training opportunities for these skills are often limited by the need for human interviewees, increasing costs and reducing accessibility. Recent advances in natural language processing (NLP) make it feasible to create virtual multimodal agents that can simulate these interviews. This PhD thesis focuses on training medical students for the objective-structured clinical examinations (OSCEs), an exercise where they act as a doctor interacting with a “standardized patient” played by a volunteer in the presence of an evaluator who assesses their clinical and communication skills. The OSCE counts for one third of the points of the national ranking exam. The UL Faculty of Medicine has pioneered the implementation of these OSCEs in France and aims to expand their access using AI technologies. This thesis will address the AI research challenges behind creating a communication skills coaching system for OSCEs using multimodal conversational agents. New methodologies will be investigated to generate the virtual patient's textual response and to model the patient's personality capable of reacting in a way that aligns to the observed doctor's social behavior (verbal and non-verbal). State of the art Generic LLMs such as GPT-4 (OpenAI23), Gemini, Mistral, etc. have demonstrated exceptional performance across various NLP tasks. The adaptation of LLMs to specialized domains often involves fine-tuning LLMs on curated datasets rich in specialized languages, terminologies, etc. For the medical domain, several such models have been developed, ClinicalGPT (Wang23), BioMedGPT-LM-7B (Zhang24), etc. In the field of NLP, the integration of personality into chatbots has garnered increasing attention. Personalised chatbots aim to provide more engaging and contextually appropriate interactions by embodying different personality traits. The Big Five ('OCEAN', an acronym of its five traits) stands out as a widely used framework (Mount15). However, existing OCEAN-based approaches often fail to adequately represent the complexity of human personality. Recently, LIA proposed a powerful model based on OCEAN (Njifendjou25) enabling greater versatility in modelling personality. The model evaluation demonstrated that the assigned personality vectors are distinguishable by both humans and acting-as-a-judge LLMs. Thesis objectives Objective 1: knowledge-aware virtual patient text generation. We will make use of LIA's recent work on the creation of high-quality neural models adapted to medical data (Labrak24, Labrak24b) to produce data specific to the scenarios envisaged during OSCE. To generate the virtual patient's textual response we will investigate zero- and few-shot prompting, transfer learning, RAG (Lewis20), etc. so as to adapt LLMs from open-domain to professional settings (Njifenjou24), accounting for medical knowledge and speciality vocabulary. Objective 2 : social-behavior-aware text generation with personality. The virtual patient's textual response should have a personality capable of reacting in a way that conforms both to this personality and to the observed doctor's social behavior. The novel approach to personality modelling for chatbots proposed in (Njifendjou25) will be extended to address a novel challenge pertaining to the degree of self-disclosure exhibited by the agent. Indeed the agent is expected to assume the role of a (naive) patient while possessing a thorough understanding of the medical intricacies of its circumstances (required to simulate the right symptoms). An increased control of its underlying personality self-disclosure during the conversation should ensure a better simulation during OSCE. At the end of the thesis, a complete patient model will be derived and evaluated on the previously collected OSCE data.
Profil du candidat
Profil et compétences requises:
–Diplôme de master/maîtrise en traitement de texte/parole/audio/vidéo, vision par ordinateur, apprentissage automatique ou dans un domaine connexe,
–Capacité à travailler de manière autonome et en équipe,
–Solides compétences en programmation (Python, etc.) et connaissances en apprentissage profond (PyTorch, etc.),
–Bonne maîtrise de l'anglais (le français n'est pas obligatoire).
Candidate profile
Profile and skills required:
– MSc/MEng degree in text/speech/audio/video processing, computer vision, machine learning, or in a related field,
– ability to work independently and in a team,
– solid programming skills (Python etc), and deep learning knowledge (PyTorch etc),
– good command of English (French not mandatory).
Référence biblio
References
[Dhaussy25] T. Dhaussy, B. Jabaian, and F. Lefèvre, “FlowAct: A Proactive Multimodal Human-robot Interaction System with Continuous Flow of Perception and Modular Action Sub-systems,” in Proceedings of International Conference on Pattern Recognition Applications and Methods, 2025.
[Dorfner24] Dorfner, F.J., Dada, A., Busch, F., Makowski, M.R., Han, T., Truhn, D., Kleesiek, J., Sushil, M., Lammert, J., Adams, L.C., & Bressem, K.K. “Biomedical Large Languages Models Seem not to be Superior to Generalist Models on Unseen Medical Data.” ArXiv, abs/2408.13833, 2024.
[Gu23] H. Gu, C. Degachi, U. Genç, S. Chandrasegaran, and H. Verma, “On the Effectiveness of Creating Conversational Agent Personalities Through Prompting,” in Proceedings of ACM Conference, 2023.
[Huang23] Q. Huang et al., “Personalized Dialogue Generation with Persona-Adaptive Attention,” in Proceedings of 37th AAAI Conf. Artif. Intell. AAAI 2023, vol. 37, pp. 12916–12923, 2023.
[Jiang24] H. Jiang, X. Zhang, X. Cao, C. Breazeal, D. Roy, and J. Kabbara, “PersonaLLM: Investigating the Ability of Large Language Models to Express Personality Traits,” Find. Assoc. Comput. Linguist. NAACL 2024 - Find., pp. 3605–3627, 2024.
[Kovacevic24] N. Kovacevic, T. Boschung, C. Holz, M. Gross, and R. Wampfler, “Chatbots with Attitude: Enhancing Chatbot Interactions Through Dynamic Personality Infusion,” in Proceedings of 6th Conf. ACM Conversational User Interfaces, CUI 2024, 2024.
[Labrak22] Y. Labrak, A. Bazoge, R. Dufour, B. Daille, P.-A. Gourraud, E. Morin, and M. Rouvier. “ FrenchMedMCQA: A French Multiple-Choice Question Answering Dataset for Medical domain”, in Proceedings of the 13th International Workshop on Health Text Mining and Information Analysis (LOUHI), Abou Dhabi, United Arab Emirates, 2022.
[Labrak23] Y. Labrak, A. Bazoge, R. Dufour, M. Rouvier, E. Morin, B. Daille, and P.-A. Gourraud. “Drbert: A robust pre-trained model in French for biomedical and clinical domains.” In Proceedings of the 61st Annual Meeting of the Association for Computational Linguistics (Volume 1: Long Papers), ACL 2023, Toronto, Canada, July 9-14, 2023, pages 16207–16221.
[Labrak24] Y. Labrak, A. Bazoge, O. El Khettari, M. Rouvier, P. Constant Dit Beaufils et al. “DrBenchmark: A large language understanding evaluation benchmark for the French biomedical domain,” In Proceedings of the 2024 Joint International Conference on Computational Linguistics, Language Resources and Evaluation (LREC-COLING 2024), pages 5376–5390, Torino, Italia, 2024.
[Labrak24b] Labrak, Y., Bazoge, A., Morin, E., Gourraud, P., Rouvier, M., R. Dufour. “BioMistral: A Collection of Open-Source Pretrained Large Language Models for Medical Domains,” In Proceedings of Annual Meeting of the Association for Computational Linguistics, ACL 2024.
[Labrak24c] Y. Labrak, A. Moumen, R. Dufour, M. Rouvier. “Zero-Shot End-To-End Spoken Question Answering In Medical Domain,” In Proceedings of Interspeech 2024, Sep 2024, Kos Island, Greece, 2024.
[Lee24] S. Lee et al., “Do LLMs Have Distinct and Consistent Personality? TRAIT: Personality Testset designed for LLMs with Psychometrics,” arXiv.org, 2024.
[Lewis20] Lewis et al., “Retrieval-augmented generation for knowledge-intensive NLP tasks,” NIPS, 2020.
[Mao24] S. Mao, X. Wang, M. Wang, Y. Jiang, P. Xie, F. Huang, and N. Zhang. “Editing personality for large language models.”, Lecture Notes in Computer Science, LNAI,volume 15360.
[Mount15] Mount, M. K. “Big Five Personality Tests”, pages 1–1. John Wiley & Sons,Ltd, 2015.
[Njifenjou24] A. Njifenjou, V. Sucal, B. and Jabaian, and F. Lefèvre, “Role-play zero-shot prompting with Large Language Models for open-domain human-machine conversation,” arXiv.org, 2024.
[Njifenjou25] Njifenjou “Enabling Trait-based Personality Simulation in Conversational LLM Agents: Case Study of Customer Assistance in French”, In Proceedings of International Workshop on Spoken Dialog Systems, 2025.
[Serapio-Garca24] G. Serapio-Garca et al., “Personality Traits in Large Language Models,” arXiv.org, 2024.
[Sutcliffe23] R. Sutcliffe, “A Survey of Personality, Persona, and Profile in Conversational Agents and Chatbots,” arXiv.org, Dec. 2023.
[Völkel22] S. T. Völkel, “Conversational Agents with Personality,” Ludwig Maximilians Universität München, PhD Thesis Dissertation, 2022.