Offre de thèse
ENACT Contribution au Jumeau Numérique Cognitif pour la gestion de la santé et le pronostic des systèmes de production par combinaison d'apprentissage automatique et d'ontologies
Date limite de candidature
01-07-2025
Date de début de contrat
01-09-2025
Directeur de thèse
VOISIN Alexandre
Encadrement
CSI conformément à la réglementation de l'école doctorale
Type de contrat
école doctorale
équipe
MPSI - Modélisation, Pilotage, Sûreté des Systèmes Industrielscontexte
Présentation du laboratoire CRAN Created in 1980, CRAN is a “Joint Research Unit ‐ UMR 7039” shared between the University of Lorraine (Scientific Pole “Automation, Mathematics, Computer Science, and their Interactions ‐ AM2I”) and CNRS (Institute “CNRS Computer Science”). The laboratory brings together 250 members including 120 researchers (8 CNRS researchers ‐ section 7 of CoNRS), researchers from the Lorraine Cancer Institute (ICL), the Regional Hospital Centers (CHRU Nancy and CHR Thionville), or several other external organizations. The administrative service and research support services bring together 30 staff members. CRAN hosts nearly hundred doctoral and post-doctoral students. The reserach works are based on more than 20 prototypes, demonstrators, platforms or software. Some of them are labelled INFRA+. CRAN benefits from industrial collaborations with major companies such as Airbus, ArcelorMittal, CNES, EDF, Dassault Aviation, POST Luxembourg, Renault, SAFRAN, SAFT SAS, Schneider, SPIE. CRAN has strong collaborations with foreign universities or research centres. The research conducted at CRAN covers the fields of automation, signal and image processing, computer engineering, manufacturing, biology, and neurology in connection with cancer research and neurosciences. The laboratory is organized into three scientific departments: - Control Identification Diagnosis Department (CID) : automatic control, dynamical systems; - Modeling, Control, and Safety of Industrial Systems Department (MPSI) : automation, manufacturing, computer engineering; - Biology, Signals, and Systems in Oncology and Neurosciences Department (BioSiS) : biology related to cancer and neurology, signal and image processing.spécialité
Automatique, Traitement du signal et des images, Génie informatiquelaboratoire
CRAN - Centre de Recherche en Automatique de Nancy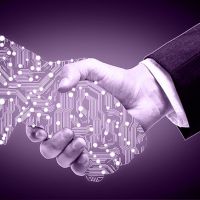
Mots clés
Jumeau numérique cognitif, intelligence artificielle, ontologie, machine learning, maintenance prévisionnelle, systèmes de production
Détail de l'offre
Les jumeaux numériques (DTs) sont de plus en plus utilisés en maintenance prédictive et en gestion de la santé des systèmes (PM&PHM). Les DTs traditionnels se concentrent sur des composants individuels (roulements, engrenages, etc.) et des services spécifiques comme la détection d'état, le diagnostic ou la prédiction de pannes. Une limitation majeure est l'absence d'une vision système, intégrant plusieurs DTs pour modéliser l'ensemble d'une ligne de production ou d'une machine complexe.
Une évolution récente est le Jumeau Numérique Cognitif (CDT), qui interconnecte sémantiquement plusieurs modèles numériques pour une coopération fluide. Contrairement aux DTs classiques, les CDTs intègrent des capacités cognitives : perception, mémoire, raisonnement, apprentissage et résolution de problèmes. Comme les DTs, ils reposent sur trois éléments : (1) un système physique, (2) une entité numérique, et (3) des connexions bidirectionnelles. Mais ils vont plus loin en intégrant plusieurs DTs avec une sémantique unifiée, leur permettant d'évoluer avec leurs homologues physiques. L'IA, les technologies sémantiques, l'IIoT et les capteurs avancés rendent ces avancées progressivement réalisables.
Les CDTs sont définis comme une représentation numérique d'un système physique augmentée de capacités cognitives facilitant des activités autonomes. Ils regroupent des modèles interconnectés couvrant tout le cycle de vie du système et de ses composants. Malgré des avancées technologiques, il n'existe pas encore de cadre global pour les CDTs appliqués au PM&PHM, et des technologies comme les ontologies, graphes de connaissances et apprentissage automatique restent sous-exploitées.
Cette recherche doctorale vise à :
Définir une architecture CDT adaptée au PM&PHM.
Modéliser et intégrer plusieurs DTs de manière sémantique pour favoriser la collaboration entre composants et services.
Ces objectifs soulèvent deux questions :
QR1 : Quels sont les éléments essentiels d'une architecture CDT pour le PM&PHM ?
QR2 : Comment les approches basées sur l'IA (ontologies, graphes de connaissances, machine learning) peuvent-elles intégrer plusieurs DTs pour un PHM intelligent ?
Répondre à ces questions nécessite l'intégration des ontologies et de l'IA basée sur les données (ex. deep learning) dans toutes les étapes du PM&PHM. Un cadre CDT doit définir comment les DTs interagissent, exploitant l'apprentissage automatique et les ontologies pour améliorer la cognition, avec des approches humain-dans-la-boucle. Une approche prometteuse combine données et raisonnement basé sur la connaissance pour la maintenance prédictive des systèmes complexes. L'apprentissage automatique extrait des modèles, tandis que les ontologies enrichissent ces données avec des requêtes sémantiques et du raisonnement basé sur des règles. L'intégration de sources de données variées améliore les modèles de prédiction, rendant les CDTs plus performants.
En combinant IA basée sur les données et raisonnement sémantique, les CDTs permettent une prédiction des pannes fiable et une interopérabilité accrue. Ils favorisent une prise de décision optimisée, une meilleure adaptabilité et ouvrent la voie à une maintenance industrielle plus autonome et efficace.
Keywords
cognitive digital twin, artificial intelligence, ontology, machine learning, predictive maintenance, productions systems
Subject details
Digital Twin (DT) technology has gained significant traction in manufacturing, particularly for predictive maintenance and Prognostic and Health Management (PM&PHM). Traditional DTs mainly focus on individual components like bearings or gearboxes, limiting their application to system-level maintenance. Recent research suggests integrating multiple DTs to enhance PM&PHM, bridging this gap. A major advancement is the Cognitive Digital Twin (CDT), which extends DTs by semantically interlinking multiple digital models for collaborative intelligence. Unlike traditional DTs, CDTs incorporate cognitive capabilities such as perception, reasoning, memory, learning, and problem-solving. Like DTs, they consist of (1) a physical entity, (2) a digital representation, and (3) bidirectional connections. However, CDTs also integrate multiple DT models with unified semantics, enabling continuous evolution alongside their physical counterparts. The CDT concept introduces a more intelligent and autonomous approach to system monitoring, supported by AI, semantic technologies, Industrial IoT (IIoT), and ubiquitous sensing. A widely accepted definition describes a CDT as a digital representation of a physical system, augmented with cognitive abilities to support autonomous decision-making. It interlinks multiple digital models across different lifecycle phases, ensuring adaptability and intelligence in real time. Despite promising developments, no comprehensive CDT framework currently exists for PM&PHM in production systems. Key enabling technologies—such as ontologies, knowledge graphs, and machine learning—remain underexplored in the context of DT cognition. Addressing this gap requires a structured approach to CDT design. This PhD research focuses on: Defining a CDT architecture and its key components for PM&PHM in production systems. Modeling and semantically integrating multiple DTs to facilitate collaborative intelligence across components and services. These objectives lead to two main research questions: RQ1: What are the essential elements of a CDT functional architecture for PM&PHM? RQ2: How can AI-driven techniques (ontologies, knowledge graphs, and ML) model and integrate multiple DTs for intelligent PHM in production systems? Solving RQ1 and RQ2 requires integrating ontologies and AI-driven approaches, including deep learning, into the PM&PHM lifecycle. A CDT framework must define interaction mechanisms between DTs while leveraging machine learning and semantic reasoning to enhance cognition. A promising direction is the fusion of machine learning, ontologies, and reasoning for predictive maintenance. Machine learning extracts patterns and failure indicators, while ontologies enhance data interpretation through semantic queries and rule-based reasoning. Integrating heterogeneous data sources improves failure prediction models, advancing CDT-driven PM&PHM. Data-driven AI allows DTs to detect and predict failures, ensuring high reliability. Meanwhile, ontologies and knowledge graphs enhance reasoning, inference, and interoperability. By combining data-driven and knowledge-based AI methods, CDTs can achieve both accuracy and interpretability, making them highly adaptable and context-aware. This hybrid approach is essential for scalable, reliable, and autonomous predictive maintenance solutions in complex industrial systems.
Profil du candidat
Le candidat idéal doit avoir une solide expertise en Machine Learning, Deep Learning et ontologies, avec une spécialisation en maintenance prédictive et jumeaux numériques pour les systèmes de fabrication et de production. Une bonne maîtrise des techniques de raisonnement basées sur l'IA, notamment les graphes de connaissances et les technologies sémantiques, est essentielle. La maîtrise de la programmation en Python est requise. Une connaissance des méthodologies d'intégration des données sera un atout.
Au-delà des compétences techniques, le candidat doit faire preuve d'excellentes capacités d'organisation et de gestion de projet, d'une autonomie avérée, ainsi que d'une approche proactive en matière d'apprentissage et de résolution de problèmes. De solides compétences en travail d'équipe et en communication sont indispensables, car la recherche impliquera une collaboration étroite avec des partenaires académiques.
Candidate profile
The ideal candidate should have a strong background in Machine Learning, Deep Learning, and Ontologies, with expertise in predictive maintenance and Digital Twins for manufacturing and production systems. A solid understanding of AI-driven reasoning techniques, including knowledge graphs and semantic technologies, is essential. Proficiency in Python programming is required. Familiarity with data integration methodologies will be advantageous. Beyond technical skills, the candidate must demonstrate strong organizational and project management abilities, the capacity to work independently, and a proactive approach to learning and problem-solving. Excellent teamwork and communication skills are essential, as the research will involve close collaboration with academic partners.
Référence biblio
- International Organization for Standardization. (2007). ISO 13374-2: 2007–Condition Monitoring and Diagnostics of Machines− Data Processing, Communication and Presentation− Part 2: Data Processing.
- van Dinter, R., Tekinerdogan, B., & Catal, C. (2022). Predictive maintenance using digital twins: A systematic literature review. Information and Software Technology, 151, 107008.
- Xiao, B., Zhong, J., Bao, X., Chen, L., Bao, J., & Zheng, Y. (2024). Digital twin-driven prognostics and health management for industrial assets. Scientific Reports, 14(1), 13443.
- Rožanec, J. M., Lu, J., Rupnik, J., Škrjanc, M., Mladenić, D., Fortuna, B., ... & Kiritsis, D. (2022). Actionable cognitive twins for decision making in manufacturing. International Journal of Production Research, 60(2), 452-478.
- Zheng, X., Lu, J., & Kiritsis, D. (2022). The emergence of cognitive digital twin: vision, challenges and opportunities. International Journal of Production Research, 60(24), 7610-7632.
- Franciosi, C., Eslami, Y., Lezoche, M., & Voisin, A. (2024). Ontologies for prognostics and health management of production systems: overview and research challenges. Journal of Intelligent Manufacturing, 1-31.
- Li, Y., Ouyang, S., & Zhang, Y. (2022). Combining deep learning and ontology reasoning for remote sensing image semantic segmentation. Knowledge-based systems, 243, 108469.