Offre de thèse
ENACT Méthode du Backstepping en dimension supérieure et IA pour équations non linéaires
Date limite de candidature
31-03-2025
Date de début de contrat
01-10-2025
Directeur de thèse
CHARPILLET François
Encadrement
voir site école doctorale IAEM : https://doctorat.univ-lorraine.fr/fr/les-ecoles-doctorales/iaem/presentation
Type de contrat
école doctorale
équipe
LARSENcontexte
Bolstered by the 500 people working in the lab, Loria, French acronym for the “Lorraine Research Laboratory in Computer Science and its Applications”, has 28 research teams, including 14 common teams with Inria. Loria is today one of the biggest research labs in the Grand Est Region. 
Team Larsen's vision is to have robots outside of the research labs and manufacturing industries. Toreach this goal, the team Larsen is developing methods to endow robots with long-termautonomy and interaction skills, taking into account the embedded and/or external sensorsin the environment. These skills are grounded on physical and social interaction, machinelearning, and planning under uncertainty. Experiments, especially in service and assistiverobotics, are at the core of our methodology. Our techniques will potentially impact all therobotics fields and catalyze the ongoing efforts to transfer robots into our society.

Find more on the Loria : https://www.loria.fr/en/loria/
And on Larsen's team: https://team.inria.fr/larsen/ Specializing in a wide range of pure and applied mathematical disciplines, the Institut Élie Cartan de Lorraine (IECL) is one of France's largest mathematics laboratories, and the largest in the Grand Est region. It has hosted world-renowned mathematicians such as Jean Leray, Jean Dieudonné, Laurent Schwartz (Fields Medal 1950), Jean-Pierre Serre (Fields Medal 1954), Alexander Grothendieck (Fields Medal 1966) and Jacques-Louis Lions. Partial differential equations (PDE) team is one of the four research group of the IECL. It gathers around 30 permanent researchers in different aspects of the PDEs, from theoretical research to numerical analysis and scientific computing. Recent developments in scientific computing at IECL has led to numerous industrial collaborations. Find more information on PDE research group at : https://iecl.univ-lorraine.fr/recherche/equations-aux-derivees-partielles/equations-aux-derivees-partielles-presentation/ 
This PhD offer is provided by the ENACT AI Cluster and its partners. Find all ENACT PhD offers and actions on https://cluster-ia-enact.ai/.spécialité
Mathématiqueslaboratoire
LORIA - Laboratoire Lorrain de Recherche en Informatique et ses Applications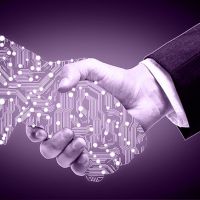
Mots clés
Stabilisation, contrôle, EDO, EDP, IA, Robotic
Détail de l'offre
Dans la théorie du contrôle, la stabilisation fait référence à la capacité de ramener un système à un équilibre stable ou instable. Pour ce faire, une action sur l'état du système est effectuée en fonction de son état actuel. Ce processus est appelé boucle de rétroaction. La stabilisation peut être obtenue pour toute une série de systèmes physiques, de faire tenir un crayon en équilibre sur le bout d'un doigt jusqu'à l'atterrissage de la fusée SpaceX.
Il existe un large éventail de méthodes en théorie du contrôle pour parvenir à la stabilisation, et plus particulièrement pour concevoir une loi de rétroaction de sorte que la boucle de rétroaction soit stable. L'une d'entre elles, qui a fait l'objet d'une attention particulière au cours de la dernière décennie, est la méthode du backstepping pour les équations différentielles partielles (EDP).
Il a été démontré [Cor15] que cette méthode fonctionne pour les équations différentielles ordinaires (ODE) linéaires, et plusieurs travaux ont été réalisés sur la méthode de backstepping pour les EDP linéaires, donnant lieu à plus de 1 000 articles dans la littérature au cours des deux dernières décennies. Malgré les progrès réalisés pour donner des conditions nécessaires et suffisantes sur l'existence de la méthode de backstepping ([GHXZ22], [GHXZ24]), cette méthode est pour l'instant restreinte aux EDP unidimensionnelles en espace.
Le premier objectif de la thèse est d'étendre cette méthode à des EDP multidimensionnelles pour des opérateurs auto-adjoints. Le but ici est de modifier le système stable cible habituellement utilisé dans la littérature, conçu pour déplacer toutes les valeurs propres d'un même facteur, en introduisant une projection spectrale pour ne déplacer que les modes propres qui ne décroissent pas suffisamment vite. Cette idée a l'avantage de lever les limitations actuelles de la méthode du backstepping, dues à la croissance non borné de la multiplicité des valeurs propres dans une dimension spatiale supérieure à 1.
Le deuxième objectif est d'explorer la philosophie de la méthode de backstepping aux EDO et EDP non linéaires en utilisant l'IA. Seuls des résultats locaux sont connus dans la littérature pour la stabilisation d'EDO et d'EDP non linéaires à l'aide de la méthode de backstepping, local signifiant ici que l'état du système doit déjà être proche de l'équilibre. Cette restriction provient principalement de la non-linéarité et de notre incapacité dans ce cas à démontrer l'existence et l'inversibilité globale de la transformation. Nous voulons contourner cette restriction en concevant des réseaux neuronaux capables d'apprendre du système stable et de concevoir une loi de rétroaction pour stabiliser le système initial. Cette méthode présenterait de nombreux avantages significatifs.

Tout d'abord, l'utilisation de l'IA pour cette procédure d'apprentissage évite complètement la nécessité de formuler l'équation d'équivalence non linéaire sur la transformation pour qu'elle cartographie le système à stabiliser vers le système cible stable, une tâche très compliquée pour les équations non linéaires. En outre, elle peut fournir des preuves numériques significatives de l'existence d'une telle transformation, ce qui permet de déterminer s'il s'agit d'une piste à suivre sur le plan théorique. Enfin, cette approche pourrait être beaucoup plus efficace pour mettre en œuvre une loi de rétroaction dans la pratique, étant donné que la convergence numérique de la rétroaction n'est pas assurée par les méthodes d'approximation numérique classiques.
Différents exemples de systèmes non linéaires seront étudiés. Tout d'abord, une EDP parabolique non linéaire à deux dimensions sera considérée, pour laquelle des réseaux neuronaux non supervisés ont déjà été conçus [AGHZ25]. Une application plus difficile, tirée des applications robotiques, sera d'entraîner les robots à imiter des mouvements humains.
Keywords
Stabilization, Control, ODE, PDE, Robotic, AI
Subject details
In control theory, stabilization refers to the ability to return a system to a stable or unstable equilibrium. To do so, an action on the state of the system is done according to its current state. This process is called a feedback loop. Stabilization may be achieved for a variety of physical system, from everyone's childhood experience to hold a pencil straight up on the tip of a finger to the landing of the SpaceX rocket. 
 A wide array of methods exist in control theory to achieve stabilization, and specifically to design a feedback law so that the feedback loop is stable. One that has gained significant attention over the past decade is the backstepping method for partial differential equations (PDE). The idea is simple : assume you want to stabilize a system to an equilibrium, and that you know a similar system that is already stable. The goal is to «learn» from the stability of the latter system to deduce the feedback law to achieve the stability of the former system. From the mathematical point of view, «learning» means to prove the existence of an invertible transformation mapping the state to stabilize to the stable state. 

 This method has been shown [Cor15] to work for linear ordinary differential equations (ODE), and extensive work has been done on the backstepping method for linear PDEs, leading to over 1000 papers in the literature over the past two decades. Despite advances to give necessary and sufficient conditions on the backstepping method to work ([GHXZ22], [GHXZ24]), this method is restricted for the moment to 1-dimensional PDEs in space. 

The first objective of the PhD thesis is to extend this method to multi-dimensional PDEs for self-adjoint operators. The goal here is to modify the target stable system usually used in the literature, designed to shift all the eigenvalues by the same factor, by introducing a spectral projection to only shift the eigenmodes not decaying sufficiently fast. This idea has the advantage of lifting the current limitations of the backstepping method, due to the unbounded growth of the multiplicity of the eigenvalues in spatial dimension greater than 1. The second objective is to explore the extension of the philosophy of the backstepping method to nonlinear ODEs or PDEs using AI. Only local results are known in the literature for the stabilization of nonlinear ODEs and PDEs using the backstepping method, local meaning here that the state of the system has to be already close to the equilibrium. This restriction mainly comes from our inability to use a global approach to prove the existence and invertibility of the transformation. We want to circumvent this restriction by designing Neural Networks able to learn from the stable system, and design a feedback law to stabilize the initial system. 

This present many significant advantages. First, the use of AI for this learning procedure avoids completely the need to formulate the nonlinear equivalence equation on the transformation for it to maps the system to stabilize to the stable target system, a very complicated task for nonlinear equations. Moreover, it may provide significant numerical evidences of the existence of such transformation, helping to determine whether it is a lead to follow theoretically. Finally, this approach could be much more efficient to implement a feedback law in practice, as the equation giving the feedback law is rather complex, and the numerical convergence of the feedback is not ensured by classical numerical approximation methods. Different examples of nonlinear systems will be investigated. First, a two-dimensional nonlinear parabolic PDE will be considered, for which unsupervised Neural Networks were already designed [AGHZ25]. A more challenging application, drawn from robotic applications, will be to train robots to learn from imitating humans movements. 

Profil du candidat
Le financement est ouvert à d'excellents étudiants en physique, en mathématiques appliquées, en ingénierie mécanique/chimique/des procédés ou dans d'autres disciplines. Nous recherchons des candidats ayant :
- un master dans une discipline pertinente.
- Une solide expérience des méthodes numériques et de l'apprentissage automatique.
- De bonnes compétences en programmation informatique.
- Une bonne Maîtrise de la rédaction et de la présentation de rapports techniques.
- D'excellente capacité à travailler en équipe, et ayant le sens de l'initiative et aptitude à travailler dans un environnement multidisciplinaire.
- Une bonne maîtrise de l'anglais,
- Une connaissance du français est souhaitable.


Candidate profile
The funding is open to excellent students from physics, applied mathematics, mechanical/chemical/process engineering or other disciplines. We are looking for candidates with:
- Master's degree in a relevant discipline.
- Solid background in numerical methods and machine learning.
- Good computer programming skills.
- Proficiency in technical report writing and presentation.
- Excellent capacity for teamwork, sense of initiative, and ability to work in a multidisciplinary environment.
- Fluent in English, some knowledge of French is beneficial.
Référence biblio
[AGHZ25] B. Appolaire, L. Gagnon, C.-K. Huang, M. Založnik, Neural Network Approximation of a Phase-Field Model for Dendritic Growth, in preparation, 2025.
[Cor15] J.-M. Coron. Stabilization of control systems and nonlinearities. In Proceedings of the 8th International Congress on Industrial and Applied Mathematics, pages 17–40. Higher Ed. Press, Beijing, 2015.
[GHXZ22] L. Gagnon, A. Hayat, S. Xiang, and C. Zhang. Fredholm transformation on 
Laplacian and rapid stabilization for the heat equation. J. Funct. Anal., 283(12):Paper No. 109664, 67p., 2022.

[GHXZ24] L. Gagnon, A. Hayat, S. Xiang, and C. Zhang. Fredholm backstepping for critical operators and application to rapid stabilization for the linearized water waves. Annales de l'Institut Fourier, Online first, 78 p., 2024