Offre de thèse
Prediction multimodale pour robots en interaction avec des humains
Date limite de candidature
30-09-2027
Date de début de contrat
01-10-2024
Directeur de thèse
IVALDI Serena
Encadrement
Bertrand Luvison, ingenieur de recherche au CEA-LIST
Type de contrat
école doctorale
équipe
LARSENcontexte
PhD funded by PEPR O2R, with co-supervision between INRIA and CEA.spécialité
Informatiquelaboratoire
LORIA - Laboratoire Lorrain de Recherche en Informatique et ses Applications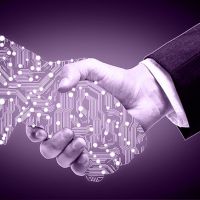
Mots clés
machine learning, robotique
Détail de l'offre
The objective of this Ph.D. is to propose new algorithms to accurately predict the future whole-body motion of a human, which is critical for human-robot collaboration. This has a direct application in social robots co-existing or interacting with humans in their environment, in exoskeletons, and in the direct teleoperation of robots with different degrees of anthropomorphism.
The thesis will target several advances in human motion prediction, such as predicting the intention of motion at different time scales, with different families of models, adding physical priors to guarantee the prediction follows the model of the human and physics constraints (e.g., the human is balanced, with the feet on the floor), and incorporating external sources of information to improve the prediction while taking the environment into account (objects nearby, payloads, obstacles from RGB-D sensors, etc.).
The level of physical interaction in this thesis will be minimal, as the focus will be particularly on situations when the onset of action must be detected to ensure a prompt anticipatory action from the robot. As an example, handovers or collaborative lifting/carrying will be studied.
The software and methods produced by Ph.D. will be a basic “building block' for many interaction scenarios addressed in the PEPR project: developing open software that can be re-used in other projects will be of paramount importance.
Keywords
machine learning, robotics
Subject details
The objective of this Ph.D. is to propose new algorithms to accurately predict the future whole-body motion of a human, which is critical for human-robot collaboration. This has a direct application in social robots co-existing or interacting with humans in their environment, in exoskeletons, and in the direct teleoperation of robots with different degrees of anthropomorphism. The thesis will target several advances in human motion prediction, such as predicting the intention of motion at different time scales, with different families of models, adding physical priors to guarantee the prediction follows the model of the human and physics constraints (e.g., the human is balanced, with the feet on the floor), and incorporating external sources of information to improve the prediction while taking the environment into account (objects nearby, payloads, obstacles from RGB-D sensors, etc.). The level of physical interaction in this thesis will be minimal, as the focus will be particularly on situations when the onset of action must be detected to ensure a prompt anticipatory action from the robot. As an example, handovers or collaborative lifting/carrying will be studied. The software and methods produced by Ph.D. will be a basic “building block' for many interaction scenarios addressed in the PEPR project: developing open software that can be re-used in other projects will be of paramount importance.
Profil du candidat
Experience with machine learning, vision, or real robots is necessary.
Experience with LLMs, visual models, generative AI, diffusion models is a plus.
Familiarity with robotic platforms and simulation environments (e.g., ROS2, Gazebo, MuJoCo, CasADi, Eigen, Pinocchio, Nvidia Isaac GYM) is a plus.
Proficiency in programming languages such as C/C++ and/or Python is required.
Ability to work independently and as part of a team.
Good communication and writing skills in English.
Candidate profile
Experience with machine learning, vision, or real robots is necessary.
Experience with LLMs, visual models, generative AI, diffusion models is a plus.
Familiarity with robotic platforms and simulation environments (e.g., ROS2, Gazebo, MuJoCo, CasADi, Eigen, Pinocchio, Nvidia Isaac GYM) is a plus.
Proficiency in programming languages such as C/C++ and/or Python is required.
Ability to work independently and as part of a team.
Good communication and writing skills in English.
Référence biblio
Penco, L.; Mouret, J.-B.; Ivaldi, S. (2023) Prescient teleoperation of humanoid robots. IEEE/RAS International Conference on Humanoid Robots (HUMANOIDS).