Offre de thèse
Quantification de la théorie statistique de l'apprentissage pour l'identification de systèmes dynamiques
Date limite de candidature
01-04-2024
Date de début de contrat
01-10-2024
Directeur de thèse
LAUER Fabien
Encadrement
La thèse sera encadrée par Fabien Lauer et Marion Gilson dans le cadre d'une collaboration entre le CRAN et le LORIA.
Type de contrat
école doctorale
équipe
contexte
Le contexte de la thèse est celui d'une collaboration entre le laboratoire d'automatique de Nancy (CRAN) et celui d'informatique (LORIA), avec un encadrant dans chaque laboratoire.spécialité
Automatique, Traitement du signal et des images, Génie informatiquelaboratoire
CRAN - Centre de Recherche en Automatique de Nancy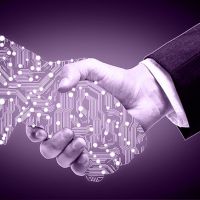
Mots clés
identification de systèmes, apprentissage machine, systèmes hybrides, théorie de l'apprentissage
Détail de l'offre
En automatique, l'identification joue un rôle essentiel dans l'obtention d'un modèle mathématique du système considéré à partir de données expérimentales, et il est crucial de pouvoir garantir les performances de ce modèle. La théorie statistique de l'apprentissage permet d'obtenir des garanties probabilistes et non asymptotiques sur la précision des modèles estimés sur des données avec un minimum d'hypothèses. Cependant, cette théorie repose en grande partie sur une hypothèse d'indépendance des données, qui n'est pas respectée lorsque celles-ci proviennent de l'observation du comportement d'un système dynamique.
Différentes pistes ont été explorées pour lever cette difficulté, notamment en s'appuyant sur la notion de processus mélangeants. Le sujet proposé ici vise quant à lui au développement d'une nouvelle approche reposant sur la prise en compte de la précision limitée des machines sur lesquelles les modèles sont implémentés, et en particulier sur la quantification des paramètres des modèles. Cette approche pourrait conduire à de nouvelles pistes pour l'obtention de garanties pour les modèles dynamiques tout en réduisant l'écart entre la théorie et la pratique.
Une attention particulière sera accordée aux systèmes dynamiques hybrides qui commutent entre plusieurs modes de fonctionnement. Ces systèmes offrent un cadre pour l'étude de nombreux systèmes cyber-physiques, mais leur identification est complexifiée dès lors que le mode de fonctionnement est inconnu. Bien que ces problèmes algorithmiques aient été largement étudiés [Lauer and Bloch, Hybrid system Identification, Spring, 2019], la question des garanties statistiques sur leurs performances reste ouverte. En effet, la plupart des travaux récents qui tentent d'adapter la théorie de l'apprentissage à l'identification des systèmes dynamiques se limitent bien souvent aux systèmes linéaires.
Keywords
system identification, machine learning, hybrid systems, statistical learning theory
Subject details
In automatic control, system identification plays a central role as it aims at the obtention of a mathematical model of the considered system from experimental data, and it is crucial to guarantee the accuracy of this model before considering its application for control. Statistical learning theory provides probabilistic and nonasymptotic guarantees on the accuracy of models estimated from data with minimal assumptions. However, this theory heavily relies on one assumption: the independence of data points, which is typically violated when the data comes from observations of the behavior of a dynamical system. Several approaches to this problem were explored, for instance by building on the notion of mixing processes. But the project here proposed aims at developing a new approach that takes into account the limited precision of computers on which the models are implemented in the derivation of learning theory results, by considering in particular the quantization of model parameters. This could provide a new way of deriving guarantees for dynamical models while also reducing the gap between theory and practice. The project will in particular focus on hybrid dynamical systems, which switch between different operating modes. These systems offer a solid framework for the analysis of numerous cyber-physical systems, but their identification is made more complex in cases where the operating mode is not observed. While these algorithmic issues have been largely studied [Lauer and Bloch, Hybrid system Identification, Spring, 2019], the issue of deriving statistical guarantees for these systems remains open. Indeed, most recent works that extend learning theoy to dynamical system identification are limited to linear systems.
Profil du candidat
Les candidats recherchés possèdent de bonnes compétences en automatique et/ou informatique et/ou apprentissage machine et/ou mathématiques appliquées, ainsi qu'un certain goût pour les travaux théoriques.
Candidate profile
The candiate should have strong skills in control theory and/or computer science and/or machine learning and/or applied mathematics, and a certain will to work on theoretical issues.
Référence biblio
F. Lauer and G. Bloch. Hybrid System Identification : Theory and Algorithms for Learning Switching Models. Springer, 2019.
L. Massucci, F. Lauer, and M. Gilson. A statistical learning perspective on switched linear system identification. Automatica, 145 :110532, 2022.