Offre de thèse
Utilisation de méthodes de détection d'anomalies “one class” pour entraîner un robot par apprentissage par démonstration
Date limite de candidature
30-09-2027
Date de début de contrat
01-10-2024
Directeur de thèse
MOURET Jean-Baptiste
Encadrement
Co-encadrant : Fabrice Mayran de Chamisso Modalité : thèse principalement réalisée au CEA ; réunions régulières avec l'encadrant HDR (UL) en visio et visites plusieurs fois par an.
Type de contrat
école doctorale
équipe
LARSENcontexte
Cette thèse se déroule dans le cadre entre le centre Inria de l'Université de Lorraine et le CEA-LIST.spécialité
Informatiquelaboratoire
LORIA - Laboratoire Lorrain de Recherche en Informatique et ses Applications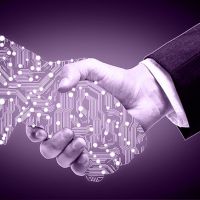
Mots clés
Aprentissage, Robotique
Détail de l'offre
L'apprentissage d'une tâche complexe en peu de démonstrations est un des enjeux majeurs de la robotique intelligente.
Cette proposition de thèse vise à utiliser des techniques de détection d'anomalies « one class » issues de la vision par ordinateur et du traitement du signal (séries temporelles, texte) pour modéliser un ensemble de démonstrations d'opérateur et isoler le concept de « normalité » combinant l'état de l'environnement et les actions du ou des acteurs (humains ou robots). Le modèle ainsi constitué sera ensuite utilisé pour apprendre à un robot à effectuer la tâche montrée par l'opérateur.
Résultats attendus : (1) Approche « one class » permettant de modéliser un ensemble de démonstrations arbitraires de manière unifiée (2) Approche de contrôle robot selon la modélisation « one class »
Keywords
Machine Learning, Robotics
Subject details
Learning complex tasks with few demonstrations is a major challenge in smart robotics. This PhD proposal aims at using “one class” anomaly detection techniques from the fields of computer vision and signal processing (time series and text) to model a set of instructor demonstrations and isolate the concept of “normality”, combining the state of the environment and that of the actors (humans or robots). The obtained model will then be used to teach a robot how to perform tasks demonstrated by the instructor. Expected results: (1) “one class” approach modeling a set of demonstrations in a unified manner (2) Robot control approach using said approach
Profil du candidat
Etudiant avec des connaissances en vision par ordinateur et robotique, type école d'ingénieur / M2 robotique ou vision.
Candidate profile
Master student with knowledge of robotics and computer vision.
Référence biblio
(1) Osa, T.; Pajarinen, J.; Neumann, G.; Bagnell, J. A.; Abbeel, P. & Peters, J.,
An Algorithmic Perspective on Imitation Learning
Foundations and Trends® in Robotics, 2018, 7, 1-179
(2) Ravichandar, H.; Polydoros, A. S.; Chernova, S. & Billard, A.
Recent Advances in Robot Learning from Demonstration
Annual Review of Control, Robotics, and Autonomous Systems, 2020, 3, 297-330
(3) Arora, S. & Doshi, P.
A survey of inverse reinforcement learning: Challenges, methods and progress
Artificial Intelligence, 2021, 297, 103500
(4) Cui, Y.; Liu, Z. & Lian, S.
A Survey on Unsupervised Visual Industrial Anomaly Detection Algorithms
2022
(5) Oh, Min-hwan; Iyengar, Garud
Sequential Anomaly Detection using Inverse Reinforcement Learning
In Proceedings of the 25th ACM SIGKDD International Conference on Knowledge Discovery & Data Mining, pp. 1480–1490, Anchorage, AK, USA, 2019
(6) Anthony Brohan, et al. Rt-1 : Robotics transformer for real-world control at scale, 2023.