Offre de thèse
Vers un apprentissage fédéré à large échelle explicable et équitable
Date limite de candidature
09-05-2025
Date de début de contrat
01-10-2025
Directeur de thèse
BRUN Armelle
Encadrement
Un suivi hebdomadaire est prévu avec les deux encadrants, pour permettre au doctorant de présenter ses avancées et de poser des questions. Tout besoin d'interaction en dehors de ce créneau est évidemment possible.
Type de contrat
école doctorale
équipe
BIRDcontexte
In an increasingly data-driven world, decentralized artificial intelligence (AI) has emerged as a key approach to addressing privacy concerns while adhering to regulations such as the General Data Protection Regulation (GDPR) 1 and the AI Act2 . This inherently minimizes reliance on a single point of data storage and enables decentralized AI to process and use data locally, thereby protecting personal information. Federated learning (FL), one of the main approaches to decentralized AI, enables models to be trained on multiple devices/servers without transferring any data. This approach aligns well with privacy regulations, but it also introduces new challenges in managing client participation (data sources) in a large-scale context. Indeed, the focus on large-scale FL meets the growing demand for AI solutions capable of managing millions of FL clients, which is crucial for the future of AI in many sectors where the use of the Internet of Things is exploding. As the number of FL clients increases, the challenge of managing heterogeneous non-independent and identically distributed data (IID) becomes more pronounced, which can compromise the fairness of the model as well as its explainability. Indeed, fairness in FL can be defined as ensuring that all participating clients benefit in a fair and balanced way from the overall model, according to their contribution and needs. While explainability in FL refers to the ability to understand, interpret and justify the decisions of the global model trained in a decentralized way. In the literature fairness and explainability are often addressed separately. Indeed, both are closely linked, as they both contribute to a more transparent, ethical and reliable system. However, their interaction can be complementary or con:licting, requiring compromise in the design of FL models, especially within a large-scale context.spécialité
Informatiquelaboratoire
LORIA - Laboratoire Lorrain de Recherche en Informatique et ses Applications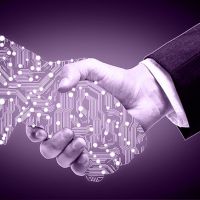
Mots clés
Apprentissage fédéré, big data, explicabilité , équité
Détail de l'offre
Dans un monde de plus en plus axé sur les données, l'intelligence artificielle (IA) décentralisée s'est imposée comme une approche clé pour répondre aux préoccupations en matière de protection de la vie privée tout en adhérant à des réglementations telles que le Règlement général sur la protection des données (RGPD) 1 et la loi sur l'IA2 . Cela minimise intrinsèquement la dépendance à l'égard d'un point unique de stockage des données et permet à l'IA décentralisée de traiter et d'utiliser les données localement, protégeant ainsi les informations personnelles.
L'apprentissage fédéré, l'une des principales approches de l'IA décentralisée, permet d'entraîner des modèles sur plusieurs appareils/serveurs sans transférer de données. Cette approche s'aligne bien sur les réglementations en matière de protection de la vie privée, mais elle introduit également de nouveaux défis dans la gestion de la participation des clients (sources de données) dans un contexte à grande échelle. En effet, l'accent mis sur la FL à grande échelle répond à la demande croissante de solutions d'IA capables de gérer des millions de clients FL, ce qui est crucial pour l'avenir de l'IA dans de nombreux secteurs où l'utilisation de l'internet des objets explose.
À mesure que le nombre de clients FL augmente, le défi de la gestion de données hétérogènes, non indépendantes et identiquement distribuées (IID) devient plus prononcé, ce qui peut compromettre l'équité du modèle ainsi que sa capacité d'explication. En effet, l'équité en FL peut être définie comme la garantie que tous les clients participants bénéficient de manière équitable et équilibrée du modèle global, en fonction de leur contribution et de leurs besoins. L'explicabilité, quant à elle, renvoie à la capacité de comprendre, d'interpréter et de justifier les décisions du modèle global formé de manière décentralisée.
Dans la littérature, l'équité et l'explicabilité sont souvent abordées séparément. En effet, ces deux aspects sont étroitement liés, car ils contribuent tous deux à rendre le système plus transparent, plus éthique et plus fiable. Toutefois, leur interaction peut être complémentaire ou contradictoire, ce qui nécessite un compromis dans la conception des modèles de FL, en particulier dans un contexte à grande échelle.
D'une part, un conflit apparaît lorsque l'amélioration de l'équité conduit à un modèle plus complexe, par exemple en appliquant des mécanismes de pondération pour corriger les biais liés aux données non IID. Ces ajustements peuvent rendre l'apprentissage moins transparent et plus difficile à expliquer, compromettant ainsi l'explicabilité du modèle. D'un autre côté, l'explicabilité et l'équité sont également complémentaires, car l'explicabilité permet d'identifier les inégalités dans la distribution des performances du modèle et d'ajuster les méthodes d'apprentissage pour assurer une distribution plus équitable des bénéfices. Ainsi, tout en assurant une plus grande équité, il est essentiel de ne pas sacrifier l'explicabilité du modèle, d'où la nécessité d'un compromis : adopter des approches qui rendent l'apprentissage équitable sans trop altérer sa compréhension et sa transparence.
L'objectif de la thèse est de créer des modèles FL qui soutiennent les principes éthiques, avec un accent particulier sur l'équité et l'explicabilité, même s'ils s'adaptent à un grand nombre de clients. Contributions attendues
Keywords
Federated Learning, big data, explainability, fairness
Subject details
In an increasingly data-driven world, decentralized artificial intelligence (AI) has emerged as a key approach to addressing privacy concerns while adhering to regulations such as the General Data Protection Regulation (GDPR) 1 and the AI Act2 . This inherently minimizes reliance on a single point of data storage and enables decentralized AI to process and use data locally, thereby protecting personal information. Federated learning (FL), one of the main approaches to decentralized AI, enables models to be trained on multiple devices/servers without transferring any data. This approach aligns well with privacy regulations, but it also introduces new challenges in managing client participation (data sources) in a large-scale context. Indeed, the focus on large-scale FL meets the growing demand for AI solutions capable of managing millions of FL clients, which is crucial for the future of AI in many sectors where the use of the Internet of Things is exploding. As the number of FL clients increases, the challenge of managing heterogeneous non-independent and identically distributed data (IID) becomes more pronounced, which can compromise the fairness of the model as well as its explainability. Indeed, fairness in FL can be defined as ensuring that all participating clients benefit in a fair and balanced way from the overall model, according to their contribution and needs. While explainability in FL refers to the ability to understand, interpret and justify the decisions of the global model trained in a decentralized way. In the literature fairness and explainability are often addressed separately. Indeed, both are closely linked, as they both contribute to a more transparent, ethical and reliable system. However, their interaction can be complementary or con:licting, requiring compromise in the design of FL models, especially within a large-scale context. On the one hand, a conflict emerges when improving fairness leads to a more complex model, for example by applying weighting mechanisms to correct biases linked to non IID data. These adjustments may make learning less transparent and more difficult to explain, thus compromising the model's explicability. On the other hand, explicability and fairness are also complementary, as explicability makes it possible to identify inequalities in the distribution of model performance and adjust learning methods to ensure a more fair distribution of benefits. So, while ensuring greater fairness, it is essential not to sacrifice the explainability of the model, thus the need for a compromise: adopting approaches that make learning fair without altering its understanding and transparency too much. The thesis's objective is to create FL models that support ethical principles, with a particular focus on fairness and explainability, even as they scale to accommodate large numbers of clients. Expected contributions - Develop a methodological framework for analyzing the interaction between fairness and explicability in a large-scale federated learning context. - Propose metrics for simultaneously assessing fairness and explainability in a large-scale federated learning system, to better understand their interactions and the necessary trade-offs. - Propose a federated learning approach that guarantees fairness while maintaining robust explicability within a large-scale context. - Validation on open datasets such as MNIST and CIFAR is expected.
Profil du candidat
Master's degree (or equivalent) in computer science, machine learning, artificial intelligence or related field.
- Solid experience in machine learning and deep learning, with a focus on model training, optimization and evaluation.
- Knowledge of federated learning or distributed AI is a plus.
Candidate profile
Master's degree (or equivalent) in computer science, machine learning, artificial intelligence or related field.
- Solid experience in machine learning and deep learning, with a focus on model training, optimization and evaluation.
- Knowledge of federated learning or distributed AI is a plus.
Référence biblio
- Ezzeldin, Y. H., Yan, S., He, C., Ferrara, E., & Avestimehr, A. S. (2023, June). Fairfed: Enabling group fairness in federated learning. In Proceedings of the AAAI conference on artificial intelligence (Vol. 37, No. 6, pp. 7494-7502).
- Shi, Y., Yu, H., & Leung, C. (2023). Towards fairness-aware federated learning. IEEE Transactions on Neural Networks and Learning Systems.
- Yu, H., Liu, Z., Liu, Y., Chen, T., Cong, M., Weng, X., ... & Yang, Q. (2020, February). A fairness-aware incentive scheme for federated learning. In AI, Ethics, and Society (pp. 393-399). Proceedings of the AAAI/ACM
- Bárcena, J. L. C., Daole, M., Ducange, P., Marcelloni, F., Renda, A., Ruffini, F., & Schiavo, A. (2022, January). Fed-XAI: Federated Learning of Explainable Artificial Intelligence Models. In IA (pp. 104-117). XAI. it@ AI*
- Huong, T. T., Bac, T. P., Ha, K. N., Hoang, N. V., Hoang, N. X., Hung, N. T., & Tran, K. P. (2022). Federated learning-based explainable anomaly detection for industrial control systems. IEEE Access, 10, 53854-53872.
- Sánchez, P. M. S., Celdrán, A. H., Xie, N., Bovet, G., Pérez, G. M., & Stiller, B. (2024). Federatedtrust: A solution for trustworthy federated learning.Systems, 152, 83-98. Future Generation Computer
- Zhang, Y., Zeng, D., Luo, J., Fu, X., Chen, G., Xu, Z., & King, I. (2024). A survey of trustworthy federated learning: Issues, solutions, and challenges and Technology, 15(6), 1-47.