Offre de thèse
ENACT. Fiabilité et fiabilisme dans le contexte des découvertes scientifiques reposant sur des méthodes d'IA
Date limite de candidature
27-04-2025
Date de début de contrat
01-10-2025
Directeur de thèse
IMBERT Cyrille
Encadrement
Co-supervision: Cyrille Imbert (AHP, CNRS, Université de Lorraine) Claus Beisbart (Institute of Philosophy at the University of Bern)
Type de contrat
école doctorale
équipe
contexte
This PhD offer is provided by the ENACT AI Cluster and its partners. Find all ENACT PhD offers and actions on https://cluster-ia-enact.ai/.' The doctoral student will be co-supervised by Cyrille Imbert (Archives Poincaré, Nancy, France) and Claus Beisbart (University of Bern; Switzerland). Cyrille Imbert is a senior researcher at CNRS, affiliated with Archives Poincaré (CNRS, Université de Lorraine). His main research interest lies in the general philosophy of science (in particular, explanation, complexity, and models), the philosophical analysis of computational science, and the social epistemology of science and epistemic activities (in particular with formal models). Claus Beisbart is a professor of philosophy of science at the University of Bern, where he is affiliated with the Institute of Philosophy and the Center for Artificial Intelligence in Medicine. His main focus is the investigation of computer-based methods in the sciences. On April 1st, he will start a new research project on the epistemology of machine learning. He is also co-directing the Embedded Ethics Lab at the Center for Artificial Intelligence in Medicine. Several people in his research group focus on AI. The group is also collaborating with a group about neuromorphic computing. Claus Beisbart's group will thus offer a stimulating environment for the PhD candidate and allow for fruitful interaction with other researchers. Archives Poincaré (Université de Lorraine, CNRS, Nancy-Strasbourg, France) The Archives Poincaré is a research institute UMR 7117 that is affiliated with the Université de Lorraine and CNRS (National Institute for Scientific Research), and it benefits from their joint support in terms of hiring academics, facilitating staff, and financial support. The IA PhD fellowships are in the continuity of the research carried out at Poincaré Archives for decades and how it will extended in the coming years. Information about the institute, its members, and salient activities may be found on its site https://poincare.univ-lorraine.fr/fr/axes. The Poincaré Archives are recognized in France and internationally for analysis by philosophers of mathematical and scientific practices in direct dialogue with science, its practices, and the new schemes of scientific reasoning. The following orientations are of particular relevance in the context of research IA research. - Analysis of theoretical and applied mathematics and their mutations - Epistemological analysis is anchored in practices, particularly mathematics and computational science. - Studies related to computer science (codes, concepts, history, digital humanities) - Study of scientific-technological mutations and their social impact (one health medicine, big data, human-machine interactions) - Ethical and political analysis of the transformations of human action: epistemic and digital democracy, social epistemology, climate ethics, decision support Research in the philosophy of science and mathematics, particularly computational science and IA, benefits from strong and regular interactions with the network of other major French or international institutions working on these questions. They typically comprise collaborative work, joint seminars, co-organized workshops and conferences, and cosupervised doctoral students. The Poincaré Archives also has a tradition of strong interactions with scientific fields. The University de Lorraine and the local institutes belonging to national research institutions (such as CNRS, INRIA, INRA, INSERM, GeorgiaTech, or AgroParisTech) provide a rich context for this purpose on fields related to physics, energy science, agriculture, computer science, or health, with various funding opportunities for interdisciplinary research, in particular in the framework of the https://www.univ-lorraine.fr/lue/ The Ph.D. candidates are expected to make the best of this appropriate research environment and to contribute to developing interactions with relevant partners in relation to the philosophy of IA. Institute of Philosophy at the University of Bern The Institute of Philosophy at the University of Bern is one of the largest philosophy departments in Switzerland. It consists of up to 35 researchers and employees. In addition, the Institute is pleased to be welcoming many world-renowned philosophers each semester, as guests to a considerable number of international workshops, lecture series, and courses organized by the Institute. The Institute of Philosophy is subdivided into three departments, the department for logic and theoretical philosophy, the department for practical philosophy and the department for history of philosophy, which are supported by a shared administrative office. The Logic and Theoretical Philosophy division deals in teaching and research, systematically and in part historically, with problems in the areas of logic, metaphysics, epistemology, ontology, philosophy of mind, theory of action, philosophy of language, semantics, and philosophy of mathematics. In addition, in Bern we also focus on the theory and philosophy of natural sciences, and the history of the theory of natural sciences. Research working on subjects related to the philosophy and epistemology of AI involve Claus Beisbart, Dr. Tim Räz Julie Jebeile, or Vincent Lam. Recent or ongoing projects in theoretical philosophy include: - Extending the Scope of Causal Realism - Climate Change Adaptation through the Feminist Kaleidoscope - Ethical Considerations of the Relationships and Interactions between Science, Policy and the Media during the COVID-19 pandemic (ESPRIM) , MCID ECRG_03 - Ethik der Infektionskrankheiten - Improving Interpretability. Philosophy of Science Perspectives on Machine Learning - Explaining Human Nature - The Epistemology of Climate Change - The Rationales and Risks of Systematization: A Pragmatic Account of When to Systematize Thought More details available on https://www.philosophie.unibe.ch/index_eng.html.spécialité
Philosophielaboratoire
AHP-PReST - Archives Henri Poincaré - Philosophie et Recherches sur les Sciences et les Technologies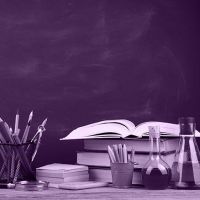
Mots clés
Apprentissage machine, Fiabilité, Fiabilisme, Conséquentialisme, Epistémologie, Règles de bonnes pratiques
Détail de l'offre
Why should we trust artificial intelligence (AI) in science and beyond, although AI models are highly opaque? A possible answer is that AI is highly reliable. This, at least, is the answer of reliabilism, a well-known position in epistemology (Durán & Formanek 2018). As a matter of fact, with the increasing use of AI in science, the reliability of AI-based methods and practices has become a central issue. Still, it seems that various fields in which AI is applied differ in how they conceptualize reliability, assess reliability, and which scientific norms they use to evaluate AI. This PhD project aims to explore the epistemological challenges related to the reliability of AI-based methods by bridging existing analyses associated with the reliability of computational practices, scientific studies concerning the assessment of AI-based methods, and recent discussions in epistemology concerning reliabilism and its fruitfulness.
This research is expected to
- contribute to a deeper understanding of the epistemological foundations of AI reliability;
- help to clarify the conditions under which AI systems can be trusted to generate epistemically justified outcomes by different types of users;
- inform the development of rules of good practice for AI-based science both within fundamental and applied science;
- provide insights about whether and where human intervention and human oversight can ensure that AI-based methods work properly and, more generally, clarify, both from a descriptive and normative perspective, the roles of humans in computational science;
- provide unifying conceptual tools to connect epistemological and ethical issues related to the use of AI-based methods and their reliability.
Target questions may, in particular, include:
- How can the epistemological theory of reliabilism, particularly its processual and consequentialist version, inform our understanding of AI reliability?
- To what extent do the well-known problems that these theories meet call for a renewed analysis in the context of AI-based methods?
- How does the use of AI-based tools impact the reliability of the larger inquiries in which they are used?
- What epistemic types of opacity are involved in AI-based methods? Are they specific to AI methods, what are their implications in terms of the reliability of these methods, and how can they be conceptualized?
- How does the reliability of AI-based methods compare with that of other empirical methods, typically those based on big data?
- To what extent is the reliability of AI-based scientific practices based on cognitive, epistemic, technological, or contextual features of the environment in which they are used?
- Are the epistemological questions concerning AI-based methods and their reliability general? Or do they significantly vary across fields, epistemic contexts, and types of practices involved?
Seed literature and methods may, in particular, involve
- mainstream philosophy of science; analytical epistemology;
- conceptual clarification; formal result interpretations; case studies, especially those comparing IA-based methods and other computational methods.
The PhD project will select some of these questions and answer them. We anticipate the following stages:
- Survey salient cases in which the factors that contribute to the (non)-reliability AIs can be analyzed
- Analyze the existing philosophical literature about reliabilism, the reliability of computational methods, and the specific scientific literature about the reliability of AIs
- Bringing together the scientific literature and the problems met by reliabilism (e.g., generality problem, conceptual issues related to epistemic consequentialism)
- Draw consequences and discuss norms concerning the designs of AI, rules of good practices, and safety rules for users.
- Analyze to what extent reliabilism may be a unifying account for ethical and epistemological purposes.
Keywords
Machine learning , Reliability, Reliabilism, Consequentialism, Epistemology , Rules of Good Practice
Subject details
Why should we trust artificial intelligence (AI) in science and beyond, although AI models are highly opaque? A possible answer is that AI is highly reliable. This, at least, is the answer of reliabilism, a well-known position in epistemology (Durán & Formanek 2018). As a matter of fact, with the increasing use of AI in science, the reliability of AI-based methods and practices has become a central issue. Still, it seems that various fields in which AI is applied differ in how they conceptualize reliability, assess reliability, and which scientific norms they use to evaluate AI. This PhD project aims to explore the epistemological challenges related to the reliability of AI-based methods by bridging existing analyses associated with the reliability of computational practices, scientific studies concerning the assessment of AI-based methods, and recent discussions in epistemology concerning reliabilism and its fruitfulness. This research is expected to - contribute to a deeper understanding of the epistemological foundations of AI reliability; - help to clarify the conditions under which AI systems can be trusted to generate epistemically justified outcomes by different types of users; - inform the development of rules of good practice for AI-based science both within fundamental and applied science; - provide insights about whether and where human intervention and human oversight can ensure that AI-based methods work properly and, more generally, clarify, both from a descriptive and normative perspective, the roles of humans in computational science; - provide unifying conceptual tools to connect epistemological and ethical issues related to the use of AI-based methods and their reliability. Target questions may, in particular, include: - How can the epistemological theory of reliabilism, particularly its processual and consequentialist version, inform our understanding of AI reliability? - To what extent do the well-known problems that these theories meet call for a renewed analysis in the context of AI-based methods? - How does the use of AI-based tools impact the reliability of the larger inquiries in which they are used? - What epistemic types of opacity are involved in AI-based methods? Are they specific to AI methods, what are their implications in terms of the reliability of these methods, and how can they be conceptualized? - How does the reliability of AI-based methods compare with that of other empirical methods, typically those based on big data? - To what extent is the reliability of AI-based scientific practices based on cognitive, epistemic, technological, or contextual features of the environment in which they are used? - Are the epistemological questions concerning AI-based methods and their reliability general? Or do they significantly vary across fields, epistemic contexts, and types of practices involved? Seed literature and methods may, in particular, involve - mainstream philosophy of science; analytical epistemology; - conceptual clarification; formal result interpretations; case studies, especially those comparing IA-based methods and other computational methods. The PhD project will select some of these questions and answer them. We anticipate the following stages: - Survey salient cases in which the factors that contribute to the (non)-reliability AIs can be analyzed - Analyze the existing philosophical literature about reliabilism, the reliability of computational methods, and the specific scientific literature about the reliability of AIs - Bringing together the scientific literature and the problems met by reliabilism (e.g., generality problem, conceptual issues related to epistemic consequentialism) - Draw consequences and discuss norms concerning the designs of AI, rules of good practices, and safety rules for users. - Analyze to what extent reliabilism may be a unifying account for ethical and epistemological purposes.
Profil du candidat
- The candidate is expected to have a Master's degree in philosophy of science, philosophy, or epistemology or to be about to complete this degree. By default, his/her curriculum should provide strong evidence of his/her ability to engage in a philosophical analysis of formal and scientific methods and, in particular, the scientific uses of AI. Strong evidence of excellent writing skills is particularly expected.
- The candidate should have a sufficient understanding of AI methods or a demonstrated ability to quickly acquire relevant and sufficient knowledge about these methods and their application in some scientific fields. Typically, the candidate may have some training or relevant courses in AI methods, an advanced scientific education such as the possession of a Bachelor of Science in a relevant field, or academic evidence that the candidate is able to develop philosophical arguments relying on information about AI methods.
- The candidate should have advanced teamwork skills and be prepared to develop regular interactions in two research sites in order to make the best of his/her joint philosophical research environment in Nancy (France) and Bern (Switzerland).
- The candidate is expected to have strong interaction and organizational skills in order to engage in exchanges with relevant scientists, philosophers in the larger international philosophy of AI community, especially in Europe, and philosophers who have relevant expertise but are not specialized in AI.
Candidate profile
- The candidate is expected to have a Master's degree in philosophy of science, philosophy, or epistemology or to be about to complete this degree. By default, his/her curriculum should provide strong evidence of his/her ability to engage in a philosophical analysis of formal and scientific methods and, in particular, the scientific uses of AI. Strong evidence of excellent writing skills is particularly expected.
- The candidate should have a sufficient understanding of AI methods or a demonstrated ability to quickly acquire relevant and sufficient knowledge about these methods and their application in some scientific fields. Typically, the candidate may have some training or relevant courses in AI methods, an advanced scientific education such as the possession of a Bachelor of Science in a relevant field, or academic evidence that the candidate is able to develop philosophical arguments relying on information about AI methods.
- The candidate should have advanced teamwork skills and be prepared to develop regular interactions in two research sites in order to make the best of his/her joint philosophical research environment in Nancy (France) and Bern (Switzerland).
- The candidate is expected to have strong interaction and organizational skills in order to engage in exchanges with relevant scientists, philosophers in the larger international philosophy of AI community, especially in Europe, and philosophers who have relevant expertise but are not specialized in AI.
Référence biblio
Buijsman, Stefan, 2024 “Over What Range Should Reliabilists Measure Reliability?” Erkenntnis 89, no. 7, 2641–61.
Durán, Juan M., and Nico Formanek. 'Grounds for trust: Essential epistemic opacity and computational reliabilism.' Minds and Machines 28 (2018): 645-666.
Duran, J. M. (2025). Beyond transparency: computational reliabilism as an externalist epistemology of algorithms. arXiv preprint arXiv:2502.20402.
Goldman, Alvin, and Bob Beddor. “Reliabilist Epistemology.” In The Stanford Encyclopedia of Philosophy, edited by Edward N. Zalta, Edward N. Zalta. Stanford University: Metaphysics Research Lab, 2016. https://plato.stanford.edu/archives/win2016/entries/reliabilism/.
Grote, T., Genin, K., & Sullivan, E. (2024). Reliability in machine learning. Philosophy Compass, 19(5), e12974.
Smart, Andrew, Larry James, Ben Hutchinson, Simone Wu, and Shannon Vallor, 2020. “Why Reliabilism Is Not Enough: Epistemic and Moral Justification in Machine Learning.” In Proceedings of the AAAI/ACM Conference on AI, Ethics, and Society, 372–77. AIES '20. New York, NY, USA: Association for Computing Machinery