Offre de thèse
ENACT Représentations and Intentionnalité des Réseaux de Neurones Artificiels
Date limite de candidature
28-04-2025
Date de début de contrat
01-09-2025
Directeur de thèse
REBUSCHI Manuel
Encadrement
KAMMERER François, CR CNRS, AHP-PReST francois.kammerer@cnrs.fr
Type de contrat
école doctorale
équipe
contexte
Cette thèse est l'occasion d'établir un lien entre la philosophie des sciences faite au sein des Archives Poincaré, et la recherche en robotique et en informatique faite au sein du Loria. Une collaboration interdisciplinaire entre philosophes, informaticiens et roboticiens peut permettre l'émergence d'une nouvelle manière de penser et de faire de l'intelligence artificielle, en rendant le champ plus connecté aux neurosciences, à la philosophie de l'esprit, mais aussi aux enjeux éthiques et sociaux contemporains.spécialité
Philosophielaboratoire
AHP-PReST - Archives Henri Poincaré - Philosophie et Recherches sur les Sciences et les Technologies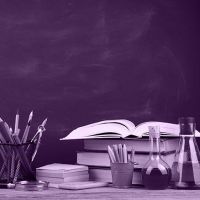
Mots clés
Intelligence Artificielle, LLM, Représentation, Intentionnalité, Connexionnisme, Fondements
Détail de l'offre
Ce projet propose d'évaluer la capacité de modèles d'intelligence artificielle (IA), notamment les Large Language Models (LLM), à représenter leur environnement et à apprendre de leurs erreurs de représentation. Les représentations artificielles sont souvent identifiées dans la recherche faite en IA avec des motifs d'activation du réseau de neurones. Les systèmes biologiques sont eux couramment décrits comme doués d'intentionnalité, capables de représentations dont le contenu porte sur le monde extérieur. Si représentation biologique et représentation artificielle sont du même ordre, comme semblent le suggérer de nombreux auteurs, certains modèles doivent être pensés comme dotés d'intentionnalité. Est-ce le cas ?
Questions :
(1) Cette première question concerne le fondement des représentations artificielles, l'information représentée, et comment cette information permet d'expliquer le fonctionnement des modèles. Deux grandes conceptions peuvent être opposées : le referential grounding, où le contenu d'une représentation est fondé sur l'objet du monde représenté, et le relational grounding, où le contenu d'une représentation est fondé sur les relations qu'elle entretient avec les autres représentations du système. Les représentations biologiques sont le plus souvent décrites comme des représentations de l'environnement et sont donc référentiellement fondées. Cependant, le relational grounding semble particulièrement bien adapté aux LLM. Il s'agit donc d'interpréter d'un point de vue philosophique les résultats de la recherche scientifique pour éclairer le débat fondationnaliste.
(2) La capacité d'un système à mal représenter son environnement et à apprendre de ses erreurs est souvent considérée comme une caractéristique majeure et décisive des agents biologiques. Qu'en est-il des réseaux de neurones ? Peut-on considérer l'apprentissage qui opère en machine learning comme une forme de correction des représentations fausses ? Si oui, par rapport à quoi est jugée la fausseté de ces représentations et est-ce suffisant pour considérer que l'IA apprend du monde extérieur comme le fait un agent biologique ? Est-ce que l‘apprentissage renforcé, systématique dans l'entraînement des LLMs, est un moyen de fonder les représentations dans le monde réel ?
(3) Peut-on penser de nouveaux modèles qui représentent le monde par une interaction dynamique et multi-variée avec l'environnement, comme le font les systèmes biologiques ? Cette partie du travail est consacrée à une réflexion philosophiquement éclairée sur la conception de tels modèles. Cette réflexion peut être mise en pratique grâce à des modèles simples qui apprennent à partir d'interactions avec les objets d'un monde virtuel comme celui d'un jeu vidéo, mais aussi par l'implémentation de modèles dans des robots. Le travail, essentiellement théorique, trouve ici une application pratique très concrète, à travers la mise en œuvre de tests empiriques d'hypothèses philosophiques.
Thématiques susceptibles d'être éclairées par les résultats de la thèse
(a) Développement d'IAs qui apprennent de manière diversifiée et sur de plus longues échelles de temps, de manière à étendre leur environnement causal. Cet élargissement de l'environnement peut permettre à certains modèles de représenter un contenu qui ne porte pas simplement des textes ou sur des images mais porte directement sur des objets du monde.
(b) Les LLM constituent-ils un argument en faveur d'une conception connexionniste de l'esprit ?
(c) Eclairer certains débats sociétaux contemporains sur l'IA. La question des représentations est liée à celle de l'agentivité qui est un enjeu central des réflexions éthiques sur l'IA. Interroger la nature des représentations des réseaux de neurones permet de mieux aborder les débats éthiques autour de l'IA en comprenant plus finement la nature des objets traités, permettant ainsi la construction d'arguments éthiques et politiques philosophiquement et mathématiquement solides.
Keywords
Artificial Intelligence, LLM, Representation, Intentionality, Connectionism, Grounding
Subject details
This project proposes to explore issues at the crossroads of artificial intelligence, neuroscience, philosophy of mind and language by evaluating the ability of artificial intelligence (AI) models, notably large language models (LLMs), to represent their environment and to learn from their representational errors. Artificial representations are most often identified in AI research with neural network activation patterns. Causal intervention techniques are implemented on artificial neural networks (ANNs) to link the activation of certain neurons to a specific behaviour. Biological systems are commonly described as having intentions, i.e. representations whose content relate to the external world. If mental representation and artificial representation are of the same order, as many authors seem to suggest, certain models must be seen as endowed with intentions. Is this the case? Research areas (1) There are two main grounding conceptions: referential grounding, where the content of a representation is grounded in the object in the world, and relational grounding, where the content of a representation is based on the relations it entertains with other representations in the system. The latter seems particularly well suited to LLMs. Biological representations are commonly described as representations of the environment and are thus referentially grounded. This is why seeing ANNs as capable of grounding the content of their representations in the external world is a big step in favor of identifying artificial and biological representations. The aim is therefore to interpret the results of scientific research on LLMs from a philosophical point of view to clarify the grounding debate. (2) The ability of a system to misrepresent its environment and learn from its mistakes is often considered to be a decisive characteristic of biological agents. What about neural networks? Can machine learning be considered as a form of correction of misrepresentations? If so, how is the falsity of these representations judged, and is this enough to consider that AI learns from the outside world in the same way as a biological agent? Is reinforced learning with human feedback in LLMs a way of basing representations in the real world? (3) Can we design new models that represent the world through dynamic, multivariate interaction with the environment, as biological systems do? This part of the work would therefore be devoted to thinking about the design of such models using advanced philosophical theories of content. But it would also be interesting to implement them in practice to test certain hypotheses, using simple models that learn from interactions with objects in a virtual world, such as that of a video game. This could also be achieved by implementing AI models in robots. The work, which is mainly philosophical and theoretical, could find a very concrete practical application here, through the implementation of empirical tests of the hypotheses. Broader questions (a) Development of AIs that learn in a diversified way and on longer time scales, with extended causal environment. This extended environment may enable certain models to represent content that is not simply text or images and does not emerge from feedback from a small group of socially homogeneous humans but instead relates directly to objects in the world. (b) Can the existence of referentially grounded ANNs such as LLMs be considered as an argument in favor of a connectionist conception of the mind? (c) The question of representations is linked to that of agentivity, which is a central issue in ethical thinking on AI. Questioning the nature of neural network representations can help to tackle the ethical debates surrounding AI by providing a more detailed understanding of the nature of the objects dealt with, thus enabling the construction of philosophically and mathematically sound ethical and political arguments.
Profil du candidat
Candidats titulaires d'un master en philosophie, informatique, sciences cognitives ou dans un domaine connexe. Solide compréhension des concepts en philosophie de l'esprit et du langage. Capacité à analyser de manière critique les implications éthiques et épistémologiques de l'IA. Maîtrise des méthodologies de recherche qualitative et quantitative. Connaissance approfondie des réseaux de neurones et de l'apprentissage automatique. Les candidats doivent démontrer une aptitude à la rédaction académique, une curiosité intellectuelle et une capacité à travailler de manière autonome sur des projets de recherche complexes. La maîtrise de l'anglais est requise.
Candidate profile
Candidates with a master's degree in philosophy, computer science, cognitive science or a related field. Solid understanding of concepts in the philosophy of mind and language. Ability to critically analyze the ethical and epistemological implications of AI. Mastery of qualitative and quantitative research methodologies. In-depth knowledge of neural networks and machine learning. Candidates must demonstrate an aptitude for academic writing, intellectual curiosity and the ability to work independently on complex research projects. Fluency in English is required.
Référence biblio
Andler, D. (2023). Intelligence artificielle, intelligence humaine: la double énigme (Vol. 4, No. 05). Paris: Gallimard.
Bender, Emily. M., & Koller, Alexandre. (2020). Climbing towards NLU: On meaning, form, and understanding in the age of data. In Proceedings of the 58th annual meeting of the association for computational linguistics (pp. 5185-5198).
Bielecka, K., & Miłkowski, M. (2020). Error detection and representational mechanisms. What are mental representations, 287-313.
Buckner, C. (2019). Deep learning: A philosophical introduction. Philosophy compass, 14(10), e12625.
Buckner, C. (2021). A forward-looking theory of content. Ergo an Open Access Journal of Philosophy, 8.
Butlin, Patrick (2024). Reinforcement learning and artificial agency. Mind and Language 39 (1):22-38.
Chaudhari, Shreya et al. (2024). RLHF Deciphered: A Critical Analysis of Reinforcement Learning from Human Feedback for LLMs. arXiv preprint arXiv:2404.08555.
Christiano, Paul F., et al. Deep reinforcement learning from human preferences. (2017) Advances in neural information processing systems 30.
Chuang, Y. S., Xie, Y., Luo, H., Kim, Y., Glass, J., & He, P. (2023). Dola: Decoding by contrasting layers improves factuality in large language models. arXiv preprint arXiv:2309.03883.
Geshkovski, B., Letrouit, C., Polyanskiy, Y., & Rigollet, P. (2023). A mathematical perspective on transformers. arXiv preprint arXiv:2312.10794.
Dretske, F. I. (1981). Knowledge and the Flow of Information.
Fodor, J. A. (2000). The mind doesn't work that way: The scope and limits of computational psychology. MIT press.
Geva, M., Schuster, R., Berant, J., & Levy, O. (2020). Transformer feed-forward layers are key-value memories. arXiv preprint arXiv:2012.14913.
Grindrod, Jumbly (2024). Large language models and linguistic intentionality. Synthese 204 (2):1-24.
Guest, O., & Love, B. C. (2019). Levels of representation in a deep learning model of categorization. BioRxiv, 626374.
Harnad, Stevan (1990). The symbol grounding problem. Physica D 42:335-346.
Hicks, Michael Townsen ; Humphries, James & Slater, Joe (2024). ChatGPT is bullshit. Ethics and Information Technology 26 (2):1-10.
Kripke, Saul A. (1980). Naming and Necessity: Lectures Given to the Princeton University Philosophy Colloquium. Cambridge, MA: Harvard University Press. Edited by Darragh Byrne & Max Kölbel.
Lederman, Harvey & Mahowald, Kyle (2024). Are Language Models More Like Libraries or Like Librarians? Bibliotechnism, the Novel Reference Problem, and the Attitudes of LLMs. Transactions of the Association for Computational Linguistics 12:1087-1103.
Liu, Hao, Carmelo Sferrazza, and Pieter Abbeel. (2023) Chain of hindsight aligns language models with feedback. arXiv preprint arXiv:2302.02676.
Mandelkern, Matthew, & Linzen, Tal. (2024). Do language models refer?. Computational Linguistics, 50(3).
Mikolov, T., Chen, K., Corrado, G., & Dean, J. (2013). Efficient estimation of word representations in vector space. arXiv preprint arXiv:1301.3781.
Millière, R., & Buckner, C. (2024). A philosophical introduction to language models-part ii: The way forward. arXiv preprint arXiv:2405.03207.
Millikan, Ruth Garrett (1984). Language, Thought, and Other Biological Categories: New Foundations for Realism. MIT Press.
Mollo, Dimitri Coelho, & Millière, Raphaël. (2023). The vector grounding problem. arXiv e-prints, arXiv-2304.
Neander, Karen (2017). A Mark of the Mental: A Defence of Informational Teleosemantics. Cambridge, USA: MIT Press.
Orgad, H., Toker, M., Gekhman, Z., Reichart, R., Szpektor, I., Kotek, H., & Belinkov, Y. (2024). Llms know more than they show: On the intrinsic representation of llm hallucinations. arXiv preprint arXiv:2410.02707.
Ouyang, Long, et al. Training language models to follow instructions with human feedback. (2022) Advances in neural information processing systems: 35 27730-27744.
Papineau, David (2005). Naturalist Theories of Meaning. In Ernie Lepore & Barry C. Smith, The Oxford Handbook of Philosophy of Language. Oxford, England: Oxford University Press. pp. 175-188.
Pavlick, Ellie. (2023). Symbols and grounding in large language models. Philosophical Transactions of the Royal Society A, 381(2251), 20220041.
Piantadosi, Steven T., & Hill, Felix. (2022). Meaning without reference in large language models. arXiv preprint arXiv:2208.02957.
Piccinini, Gualtiero (2020a). Neurocognitive Mechanisms: Explaining Biological Cognition. Oxford University Press.
Piccinini, G. (2020b). Nonnatural mental representation. What are mental representations, 254-286.
Piccinini, G. (2022). Situated neural representations: Solving the problems of content. Frontiers in Neurorobotics, 16, 846979.
Putnam, Hilary (1975). The meaning of 'meaning'. Minnesota Studies in the Philosophy of Science 7:131-193.
Selva Birunda, S., & Kanniga Devi, R. (2021). A review on word embedding techniques for text classification. Innovative Data Communication Technologies and Application: Proceedings of ICIDCA 2020, 267-281.
Shea, N. (2007). Content and its vehicles in connectionist systems. Mind & Language, 22(3), 246-269.
Shea, N. (2018). Representation in cognitive science (p. 304). Oxford University Press.
Søgaard, Anders (2021). Grounding the Vector Space of an Octopus: Word Meaning from Raw Text. Minds and Machines 33 (1):33-54.
Vaswani, A., Shazeer, N., Parmar, N., Uszkoreit, J., Jones, L., Gomez, A. N., ... & Polosukhin, I. (2017). Attention is all you need. Advances in neural information processing systems, 30.
Wang, Y. (2023). The Mathematics Underlying Transformers and ChatGPT.
Zhang, Tianjun, et al. (2023) The wisdom of hindsight makes language models better instruction followers. International Conference on Machine Learning. PMLR.
Ziegler, Daniel et al. (2019). Fine-tuning language models from human preferences. arXiv preprint arXiv:1909.08593.